Efficient Rematerialization for Deep Networks
ADVANCES IN NEURAL INFORMATION PROCESSING SYSTEMS 32 (NIPS 2019)(2019)
摘要
When training complex neural networks, memory usage can be an important bottleneck. The question of when to rematerialize, i.e., to recompute intermediate values rather than retaining them in memory, becomes critical to achieving the best time and space efficiency. In this work we consider the rematerialization problem and devise efficient algorithms that use structural characterizations of computation graphs-treewidth and pathwidth-to obtain provably efficient rematerialization schedules. Our experiments demonstrate the performance of these algorithms on many common deep learning models.
更多查看译文
关键词
the question
AI 理解论文
溯源树
样例
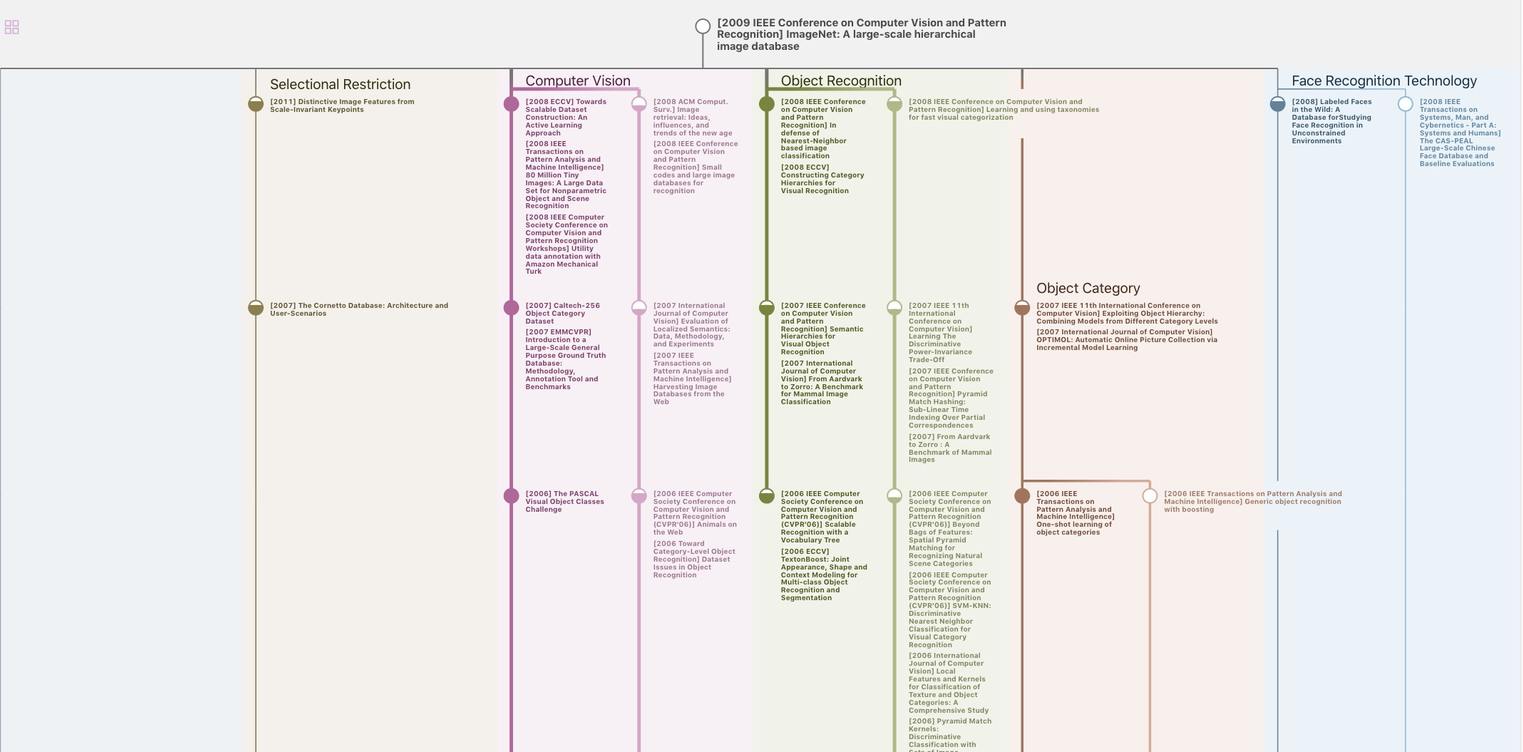
生成溯源树,研究论文发展脉络
Chat Paper
正在生成论文摘要