Curriculum-guided Hindsight Experience Replay
ADVANCES IN NEURAL INFORMATION PROCESSING SYSTEMS 32 (NIPS 2019)(2019)
摘要
In off-policy deep reinforcement learning, it is usually hard to collect sufficient successful experiences with sparse rewards to learn from. Hindsight experience replay (HER) enables an agent to learn from failures by treating the achieved state of a failed experience as a pseudo goal. However, not all the failed experiences are equally useful to different learning stages, so it is not efficient to replay all of them or uniform samples of them. In this paper, we propose to 1) adaptively select the failed experiences for replay according to the proximity to true goals and the curiosity of exploration over diverse pseudo goals, and 2) gradually change the proportion of the goal-proximity and the diversity-based curiosity in the selection criteria: we adopt a human-like learning strategy that enforces more curiosity in earlier stages and changes to larger goal-proximity later. This "Goal-and-Curiosity-driven Curriculum Learning" leads to "Curriculum-guided HER (CHER)", which adaptively and dynamically controls the exploration-exploitation trade-off during the learning process via hindsight experience selection. We show that CHER improves the state of the art in challenging robotics environments.
更多查看译文
关键词
hindsight experience replay
AI 理解论文
溯源树
样例
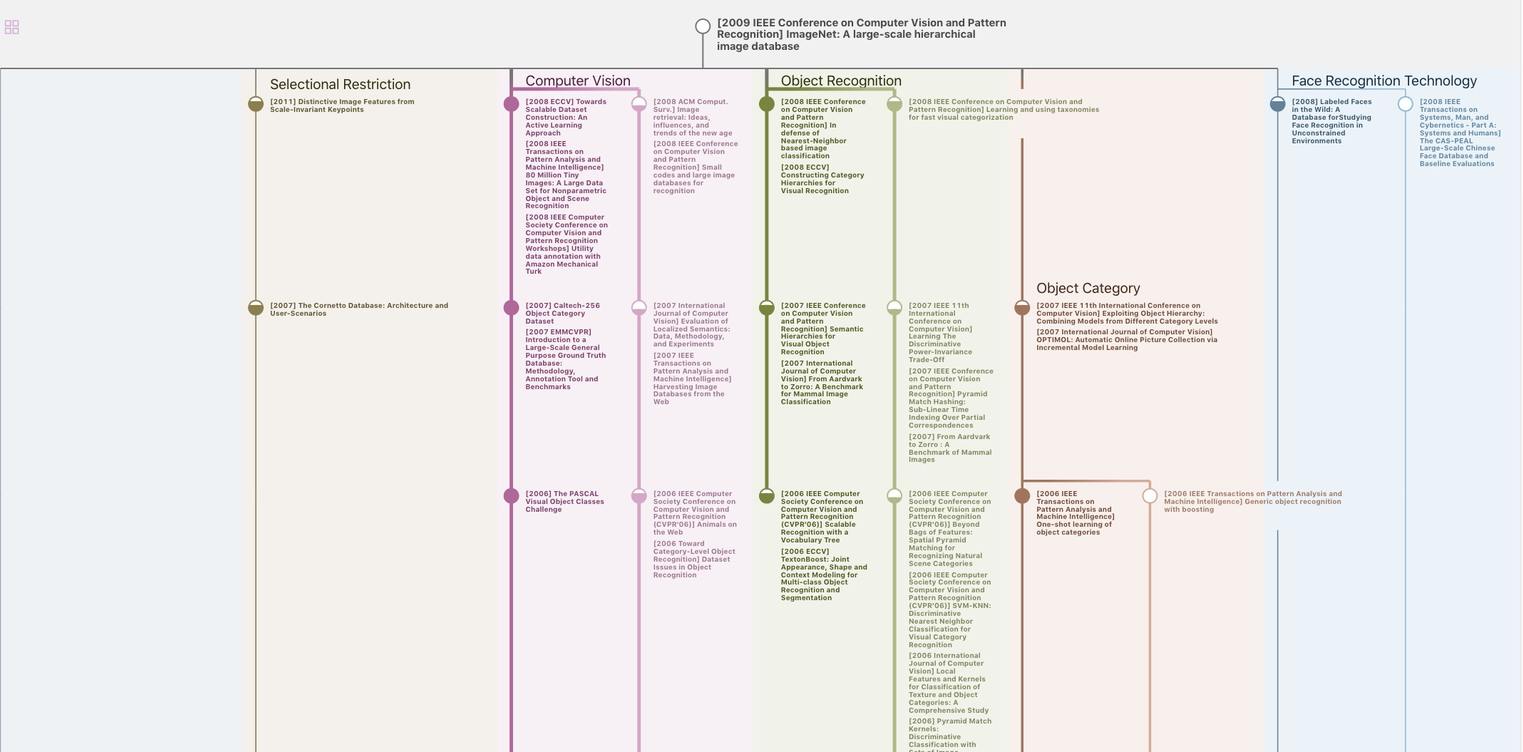
生成溯源树,研究论文发展脉络
Chat Paper
正在生成论文摘要