No Press Diplomacy: Modeling Multi-Agent Gameplay
NeurIPS(2019)
摘要
Diplomacy is a seven-player non-stochastic, non-cooperative game, where agents acquire resources through a mix of teamwork and betrayal. Reliance on trust and coordination makes Diplomacy the first non-cooperative multi-agent benchmark for complex sequential social dilemmas in a rich environment. In this work, we focus on training an agent that learns to play the No Press version of Diplomacy where there is no dedicated communication channel between players. We present DipNet, a neural-network-based policy model for No Press Diplomacy. The model was trained on a new dataset of more than 150,000 human games. Our model is trained by supervised learning (SL) from expert trajectories, which is then used to initialize a reinforcement learning (RL) agent trained through self-play. Both the SL and RL agents demonstrate state-of-the-art No Press performance by beating popular rule-based bots.
更多查看译文
关键词
reinforcement learning,supervised learning,semi-supervised learning,cooperative game
AI 理解论文
溯源树
样例
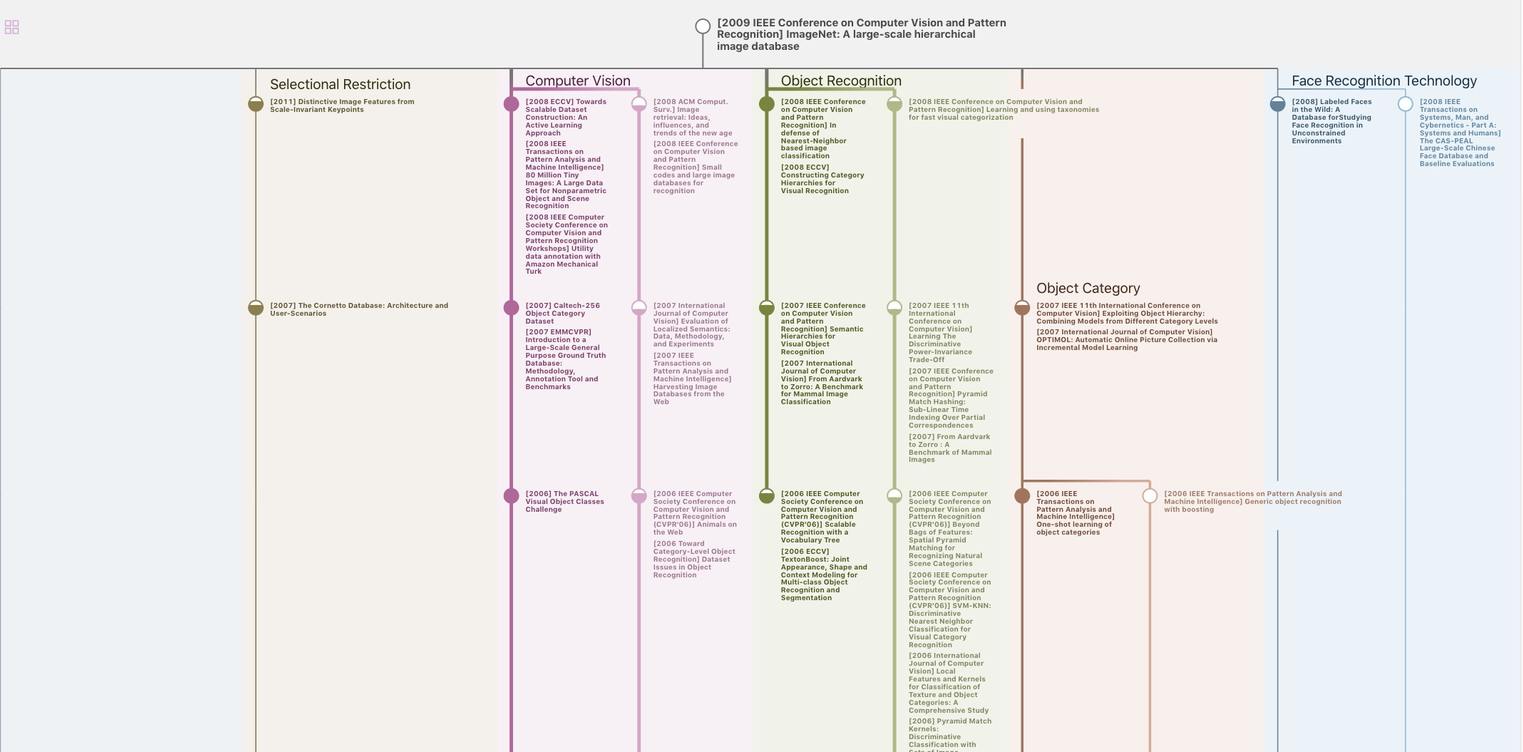
生成溯源树,研究论文发展脉络
Chat Paper
正在生成论文摘要