Nonlinear Blind Compressed Sensing Under Signal-Dependent Noise
2019 IEEE INTERNATIONAL CONFERENCE ON IMAGE PROCESSING (ICIP)(2019)
摘要
In this paper, we consider the problem of nonlinear blind compressed sensing, i.e. jointly estimating the sparse codes and sparsity-promoting basis, under signal-dependent noise. We focus our efforts on the Poisson noise model, though other signal-dependent noise models can be considered. By employing a well-known variance stabilizing transform such as the Anscombe transform, we formulate our task as a nonlinear least squares problem with the l(1) penalty imposed for promoting sparsity. We solve this objective function under non-negativity constraints imposed on both the sparse codes and the basis. To this end, we propose a multiplicative update rule, similar to that used in non-negative matrix factorization (NMF), for our alternating minimization algorithm. To the best of our knowledge, this is the first attempt at a formulation for nonlinear blind compressed sensing, with and without the Poisson noise model. Further, we also provide some theoretical bounds on the performance of our algorithm.
更多查看译文
关键词
Blind Compressed Sensing, Anscombe Transform, Multiplicative Update, Performance Bounds
AI 理解论文
溯源树
样例
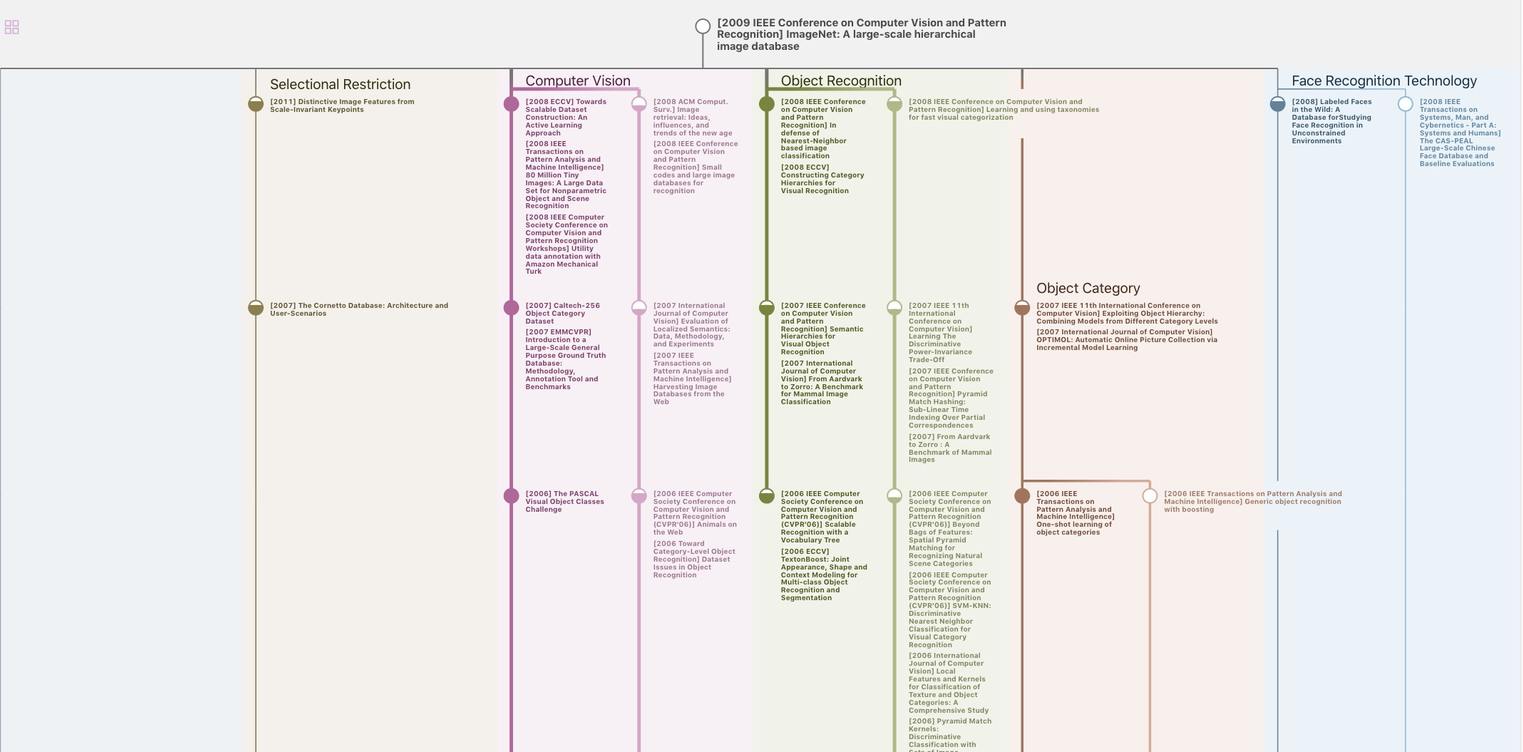
生成溯源树,研究论文发展脉络
Chat Paper
正在生成论文摘要