Homocentric Hypersphere Feature Embedding For Person Re-Identification
2019 IEEE INTERNATIONAL CONFERENCE ON IMAGE PROCESSING (ICIP)(2019)
摘要
Triplet loss and softmax loss are two widely used loss functions in Person Re-Identification (Person ReID). However, previous works that try to apply these two loss functions have measure inconsistency during training and testing stage and among different parts of the total loss function, which would cause inferior performance of models. To address this issue, we propose a novel homocentric hypersphere embedding scheme to decouple magnitude and orientation information for both feature and weight vectors, and reformulate the triplet loss and the softmax loss to their angular versions and combine them into an angular discriminative loss. We evaluate our proposed method extensively on the widely used Person ReID benchmarks. Our method demonstrates leading performance on all datasets.
更多查看译文
关键词
Person re-identification, deep learning, feature learning, metric learning
AI 理解论文
溯源树
样例
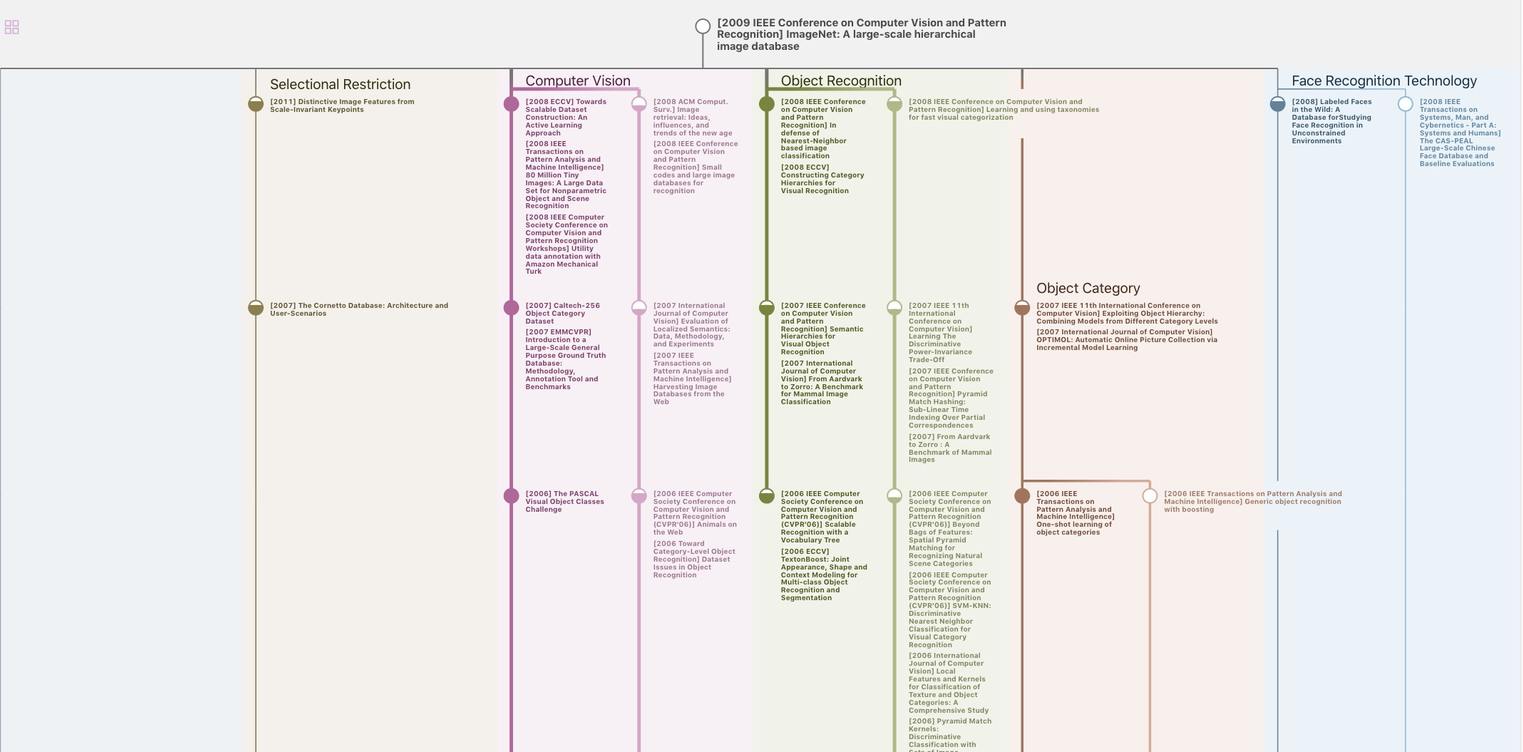
生成溯源树,研究论文发展脉络
Chat Paper
正在生成论文摘要