Multi-feature Joint Sparse Representation for RGB-D Object Recognition
2018 IEEE International Conference on Information and Automation (ICIA)(2018)
摘要
It’s still a challenge to recognize object with RGB-D information. HMP is a classical method based on sparse coding, which can adapt to learn feature from RGB-D. HMP method ignore gradient information. And SIFT based sparse coding could capture gradient information well, while cannot adaptively extract other feature from RGB-D. So we propose multi-feature joint sparse representation (MJSR) algorithms, which combine sparse coding based on SIFT and HMP. At first, we extract dense-SIFT from image. Then dictionary is captured with K-SVD algorithm. Sparse coding can be obtained by using Matching Pursuit (MP) on dictionary and sift features. Spatial pyramid pooling is applied on sparse coding based on SIFT and the features consist of patch feature and associated sparse coding as HMP to capture image feature. In the end, we conduct experiment on Washington RGB-D object dataset.
更多查看译文
关键词
RGB-D,object recognition,sparse coding,multi-feature joint
AI 理解论文
溯源树
样例
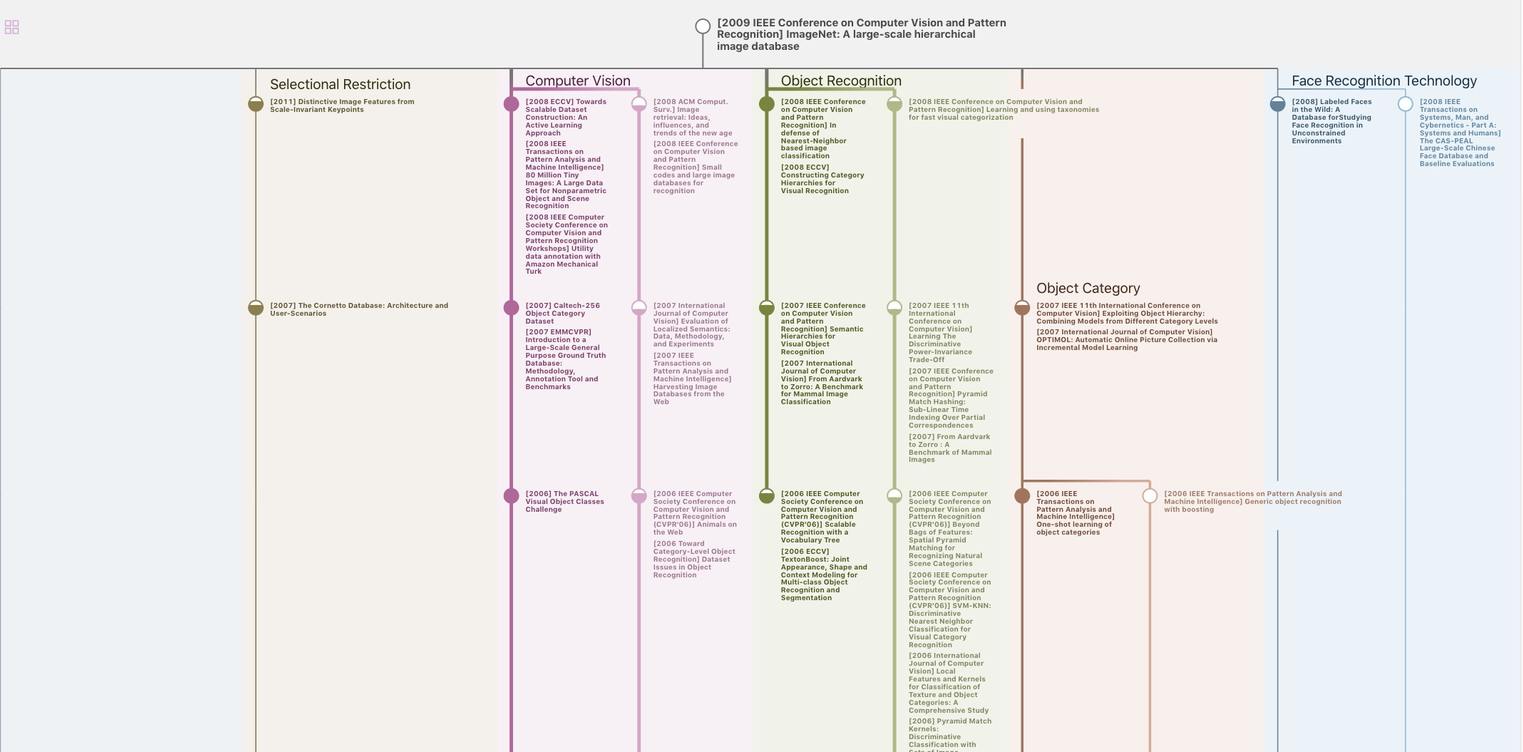
生成溯源树,研究论文发展脉络
Chat Paper
正在生成论文摘要