A Novel Thought Of Pruning Algorithms: Pruning Based On Less Training
PRICAI 2019: TRENDS IN ARTIFICIAL INTELLIGENCE, PT II(2019)
摘要
Pre-training of models in pruning algorithms plays an important role in pruning decision-making. We find that excessive pre-training is not necessary for pruning algorithms. According to this idea, we propose a pruning thought-Incremental pruning based on less training (IPLT). We can combine IPLT with almost all existing pruning algorithms. Compared with the original pruning algorithms based on a large number of pre-training, the modified algorithms (by IPLT) has competitive compression effect. On the premise of ensuring accuracy, the pruning algorithms modified by IPLT can achieve 8x-9x compression for VGG-16 on CIFAR-10 and only needs to pre-train few epochs. For VGG-16 on CIFAR-10, we can not only achieve 10x test acceleration, but also about 10x training acceleration. At present, the research mainly focuses on the compression and acceleration in the application stage of the models, while the compression and acceleration in the training stage are few. We newly proposed the thought of IPLT that can compress and accelerate in the training stage. It is novel to consider the amount of pre-training required by pruning algorithm. Our results have implications: Too much pre-training may be not necessary for pruning algorithms.
更多查看译文
关键词
Pruning algorithms, Amount of pre-training, Too many
AI 理解论文
溯源树
样例
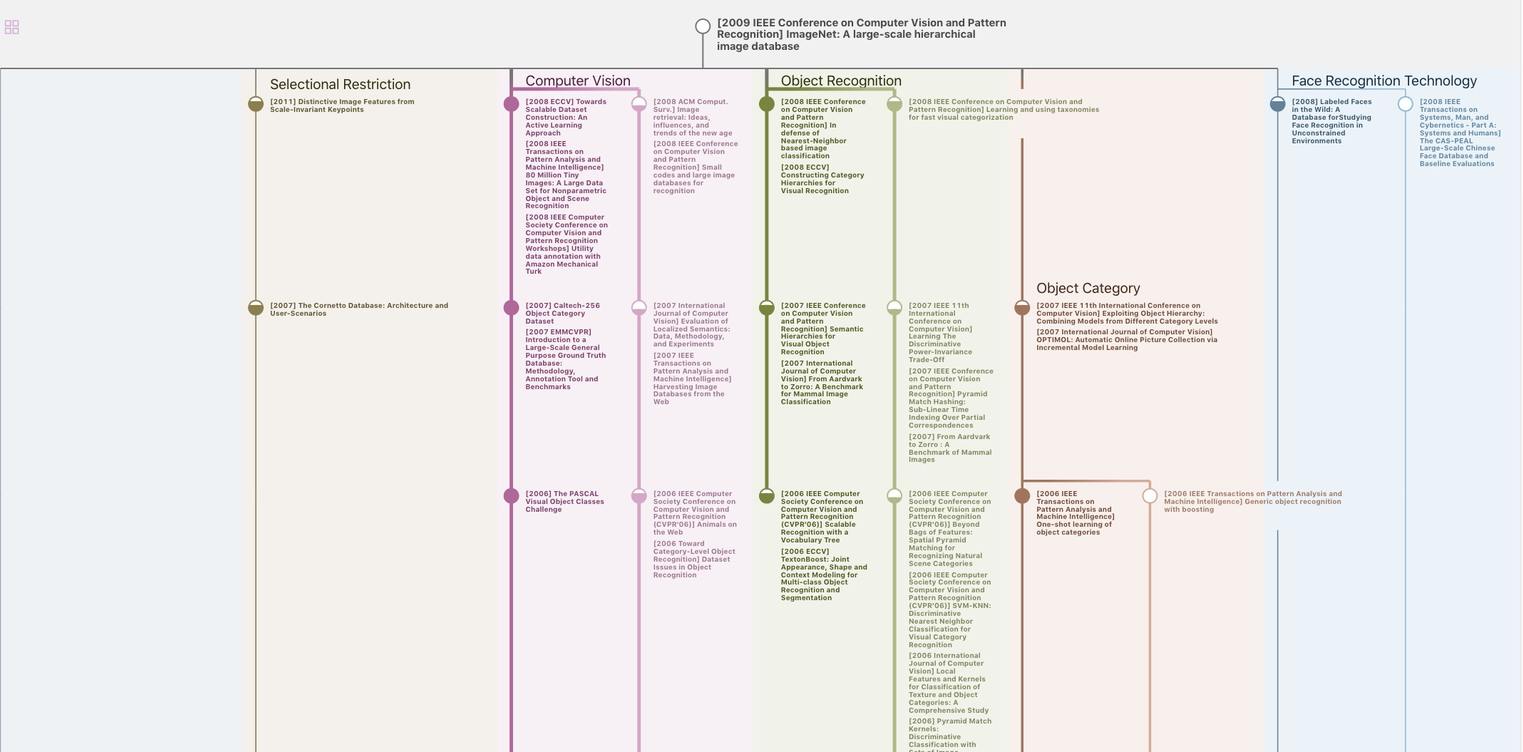
生成溯源树,研究论文发展脉络
Chat Paper
正在生成论文摘要