Stock market trend prediction using a functional time series approach
QUANTITATIVE FINANCE(2020)
摘要
Thanks to advanced technologies, ultra-high-frequency limit order book (LOB) data are now available to data analysts. An LOB contains comprehensive information on all transactions in a market. We use LOB data to investigate the high-frequency dynamics of market supply and demand (S-D) and inspect their impacts on intra-daily market trends. The intra-daily S-D curves are fitted with B-spline basis functions. Technique of multi-resolution is introduced to capture inhomogeneous curvature of the S-D curves and a lasso-type criterion is employed to select a common basis set. Based on empirical evidence, we model the time varying coefficients in the B-spline interpolation by vector autoregressive models of order . The Xgboost algorithm is employed to extract information from the areas under the S-D curves to predict the intra-daily market trends. In the empirical study, we analyze the LOB data from LOBSTER (). The results show that the proposed approach is able to recover the S-D curves and has satisfactory performance on both curve and market trend predictions.
更多查看译文
关键词
Area under curve,B-spline,Functional autoregressive model,Multi-resolution,Vector autoregressive model
AI 理解论文
溯源树
样例
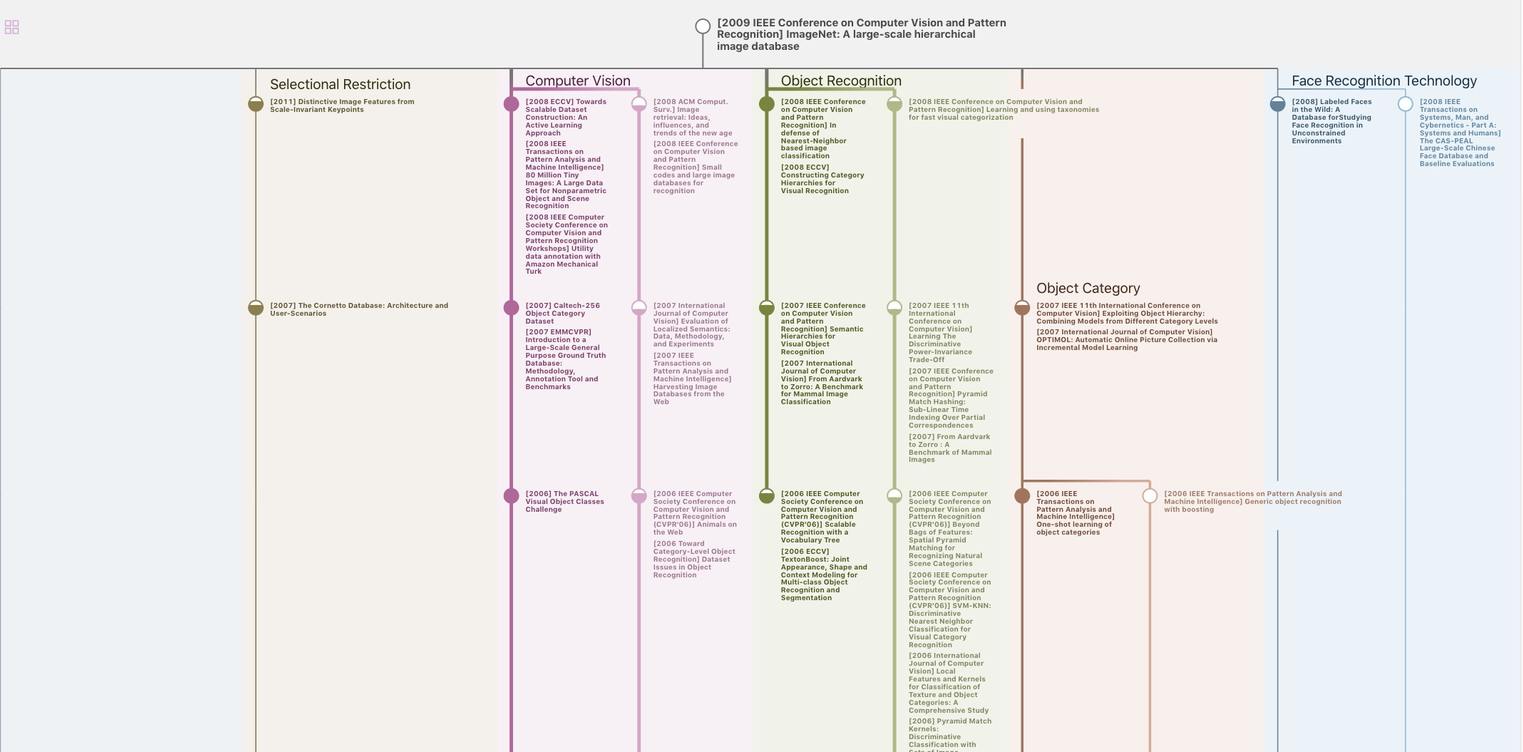
生成溯源树,研究论文发展脉络
Chat Paper
正在生成论文摘要