Multi-Stream Convolutional Networks For Indoor Scene Recognition
COMPUTER ANALYSIS OF IMAGES AND PATTERNS, CAIP 2019, PT I(2019)
摘要
Convolutional neural networks (CNNs) have recently achieved outstanding results for various vision tasks, including indoor scene understanding. The de facto practice employed by state-of-the-art indoor scene recognition approaches is to use RGB pixel values as input to CNN models that are trained on large amounts of labeled data (Image-Net or Places). Here, we investigate CNN architectures by augmenting RGB images with estimated depth and texture information, as multiple streams, for monocular indoor scene recognition. First, we exploit the recent advancements in the field of depth estimation from monocular images and use the estimated depth information to train a CNN model for learning deep depth features. Second, we train a CNN model to exploit the successful Local Binary Patterns (LBP) by using mapped coded images with explicit LBP encoding to capture texture information available in indoor scenes. We further investigate different fusion strategies to combine the learned deep depth and texture streams with the traditional RGB stream. Comprehensive experiments are performed on three indoor scene classification benchmarks: MIT-67, OCIS and SUN-397. The proposed multi-stream network significantly outperforms the standard RGB network by achieving an absolute gain of 9.3%, 4.7%, 7.3% on the MIT-67, OCIS and SUN-397 datasets respectively.
更多查看译文
关键词
Scene recognition, Depth features, Texture features
AI 理解论文
溯源树
样例
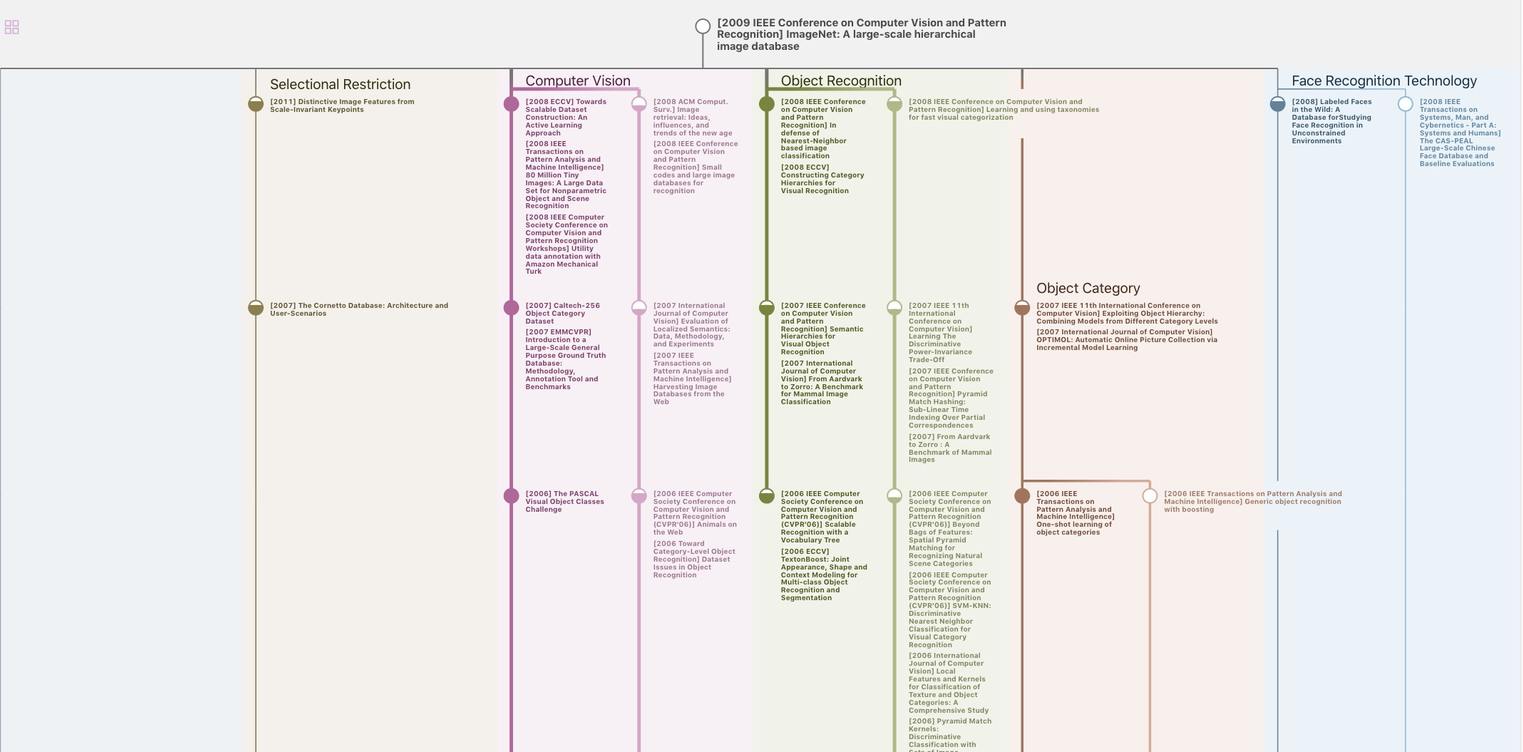
生成溯源树,研究论文发展脉络
Chat Paper
正在生成论文摘要