Differential Privacy-Based Location Protection in Spatial Crowdsourcing
IEEE Transactions on Services Computing(2022)
摘要
Spatial crowdsourcing (SC) is a location-based outsourcing service whereby SC-server allocates tasks to workers with mobile devices according to the locations outsourced by requesters and workers. Since location information contains individual privacy, the locations should be protected before being submitted to untrusted SC-server. However, the encryption schemes limit data availability, and existing differential privacy (DP) methods do not protect the tasks’ location privacy. In this paper, we propose a differential privacy-based location protection (DPLP) scheme, which protects the location privacy of both workers and tasks, and achieves task allocation with high data utility. Specifically, DPLP splits the exact locations of both workers and tasks into noisy multi-level grids by using adaptive three-level grid decomposition (ATGD) algorithm and DP-based adaptive complete pyramid grid (DPACPG) algorithm, respectively, thereby considering the grid granularity and location privacy. Furthermore, DPLP adopts an optimal greedy algorithm to calculate a geocast region around the task grid, which achieves the trade-off between acceptance rate and system overhead. Detailed privacy analysis demonstrates that our DPLP scheme satisfies
$\epsilon$
-differential privacy. The extensive analysis and experiments over two real-world datasets confirm high efficiency and data utility of our scheme.
更多查看译文
关键词
Location privacy,spatial crowdsourcing,differential privacy,task allocation,data utility
AI 理解论文
溯源树
样例
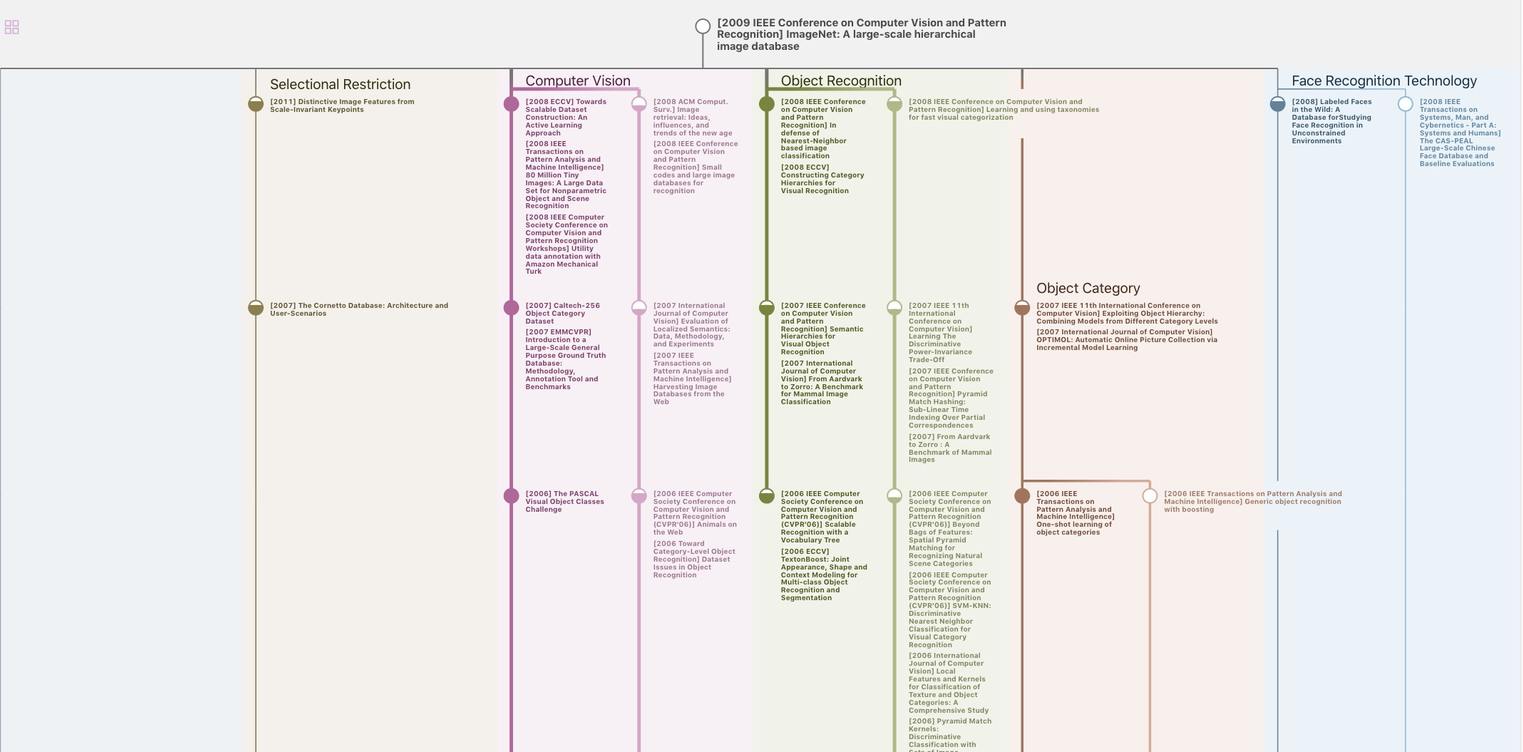
生成溯源树,研究论文发展脉络
Chat Paper
正在生成论文摘要