Refrigerant charge fault detection method of air source heat pump system using convolutional neural network for energy saving
Energy(2019)
摘要
In heat pumps, refrigerant leakage is one of the frequent faults. Since the systems have the best performance at the optimal charge, it is essential to predict refrigerant charge amount. Hence, the refrigerant charge fault detection (RCFD) methods have been developed by researchers. Due to improvements in computing speed and big-data, data-driven techniques such as artificial neural networks (ANNs) have been highlighted recently. However, most existing ANN-based RCFD methods use low-performance shallow neural networks (SNNs) and require the features extracted by experts’ experiences. Also, they have some critical limitations. First, they cannot provide quantitative information on recharge amount due to a simple classification such as undercharge or overcharge. Second, many ANN-based RCFD methods can be used in one operation mode (cooling or heating mode). To improve the limitations, a novel RCFD strategy based on convolutional neural networks (CNNs) was suggested. Two prediction models using classification and regression can predict the quantitative refrigerant amount in both cooling and heating mode with a single model. The mean accuracy of the CNN-based classification model was 99.9% for the learned cases. Also, the CNN-based regression model showed the excellent prediction performance with root-mean-square (RMS) error of 3.1% including the untrained refrigerant charge amount data.
更多查看译文
关键词
Heat pump system,Refrigerant charge fault detection,Convolutional neural network,Quantitative prediction,Classification,Regression
AI 理解论文
溯源树
样例
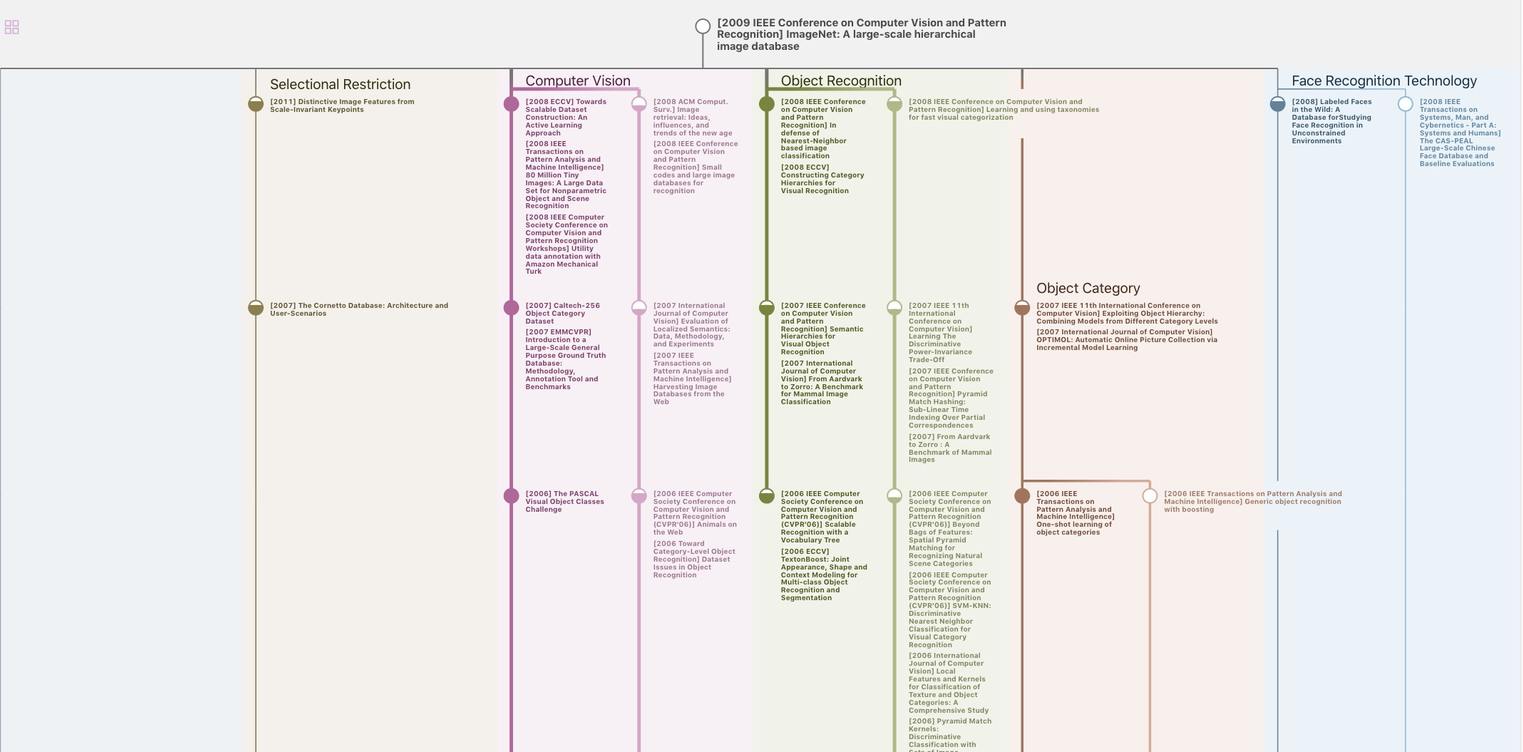
生成溯源树,研究论文发展脉络
Chat Paper
正在生成论文摘要