Joint Scheduling and Deep Learning-Based Beamforming for FD-MIMO Systems Over Correlated Rician Fading
IEEE ACCESS(2019)
摘要
In this paper, we investigate the downlink transmission for full-dimension multiple-input multiple-output (FD-MIMO) systems over correlated Rician fading channels. With only statistical channel state information at the BS, the beamforming vectors of each user are decoupled from each other through the maximization of average signal-to-leakage-plus-noise ratio (SLNR) lower bound, and the optimal beamforming vector which involves the line-of-sight component and correlation matrix of both directions is derived. To reduce the time of acquiring the beamforming vector for each user, a deep learning (DL)based algorithm is proposed. A sub-optimal analytical beamforming algorithm is also proposed for comparison. Based on the proposed beamforming method, the ergodic rate of each user is analyzed and simple closed-form approximations are derived, which are very useful in evaluating the system performance and user scheduling. Moreover, two user scheduling algorithms referred to as most dissimilar and minimum interference-to-signal factor algorithm are proposed, which greatly reduce the complexity compared to the sum rate based greedy scheduling algorithm. Simulation results validate the performance of the proposed beamforming and scheduling algorithms, and verify the accuracy of the derived ergodic rate approximations.
更多查看译文
关键词
Deep learning,massive MIMO,statistical CSI,three-dimension beamforming,user scheduling
AI 理解论文
溯源树
样例
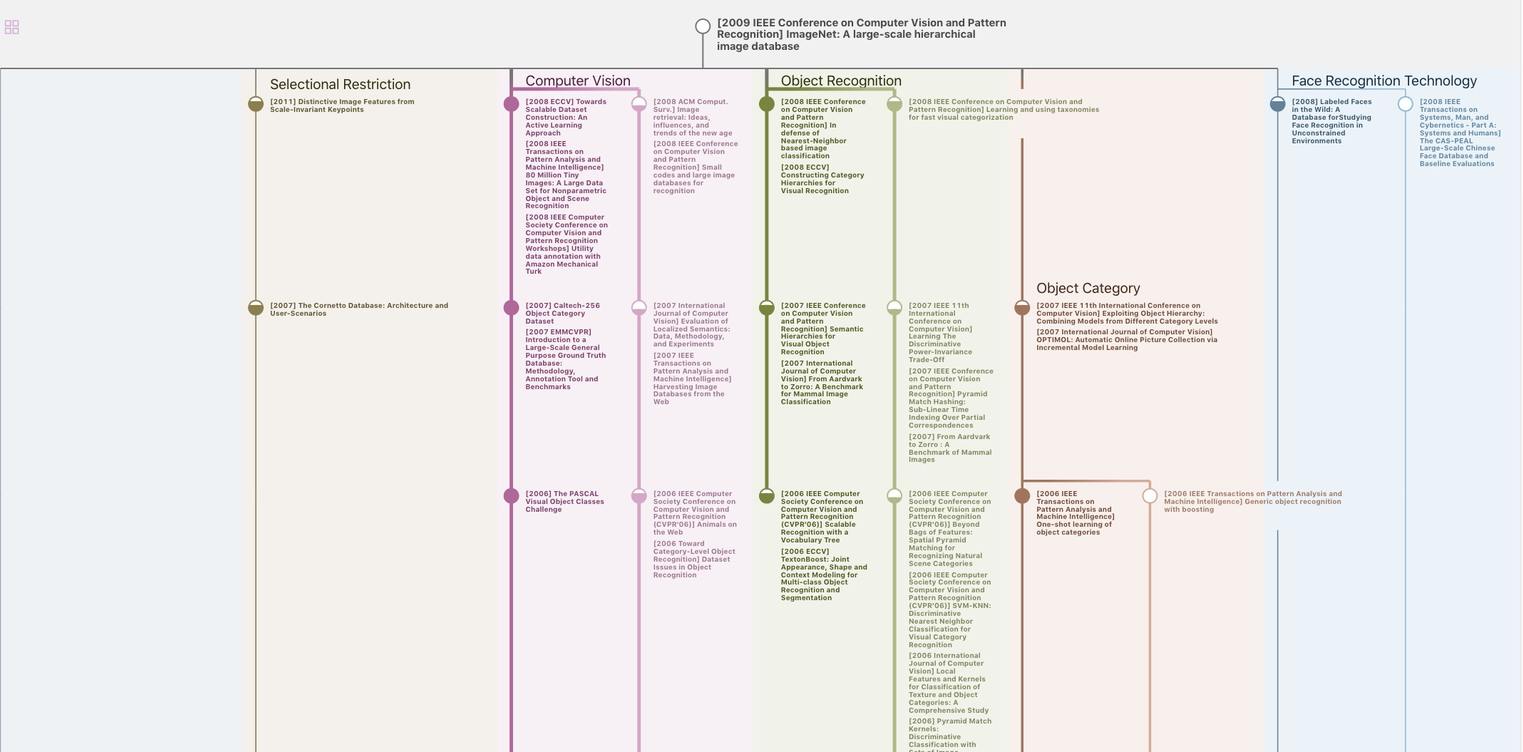
生成溯源树,研究论文发展脉络
Chat Paper
正在生成论文摘要