A Global-Matching Framework For Multi-View Stereopsis
COMPUTER ANALYSIS OF IMAGES AND PATTERNS, CAIP 2019, PT I(2019)
摘要
As deep neural network demonstrated its success on various Computer Vision problems, a number of approaches have been proposed for applying it to multi-view stereopsis. Most of these approaches train networks over small cropped image patches so that the requirements on GPU's processing power and memory space are manageable. The limitation of such approaches, however, is that the networks cannot effectively learn global information and hence have trouble handling large textureless regions. In addition, when testing on different datasets, these networks often need to be retrained to achieve optimal performances. To address this incompetency, we present in this paper a robust framework that is trained on high-resolution (1280x1664) stereo images directly. It is therefore capable of learning global information and enforcing smoothness constraints across the whole image. To reduce the memory space requirement, the network is trained to output the matching scores of different pixels under each depth hypothesis at a time. A novel loss function is designed to properly handle the unbalanced distribution of matching scores. Finally, trained over binocular stereo datasets only, we show that the network can directly handle the DTU multi-view stereo dataset and generate results comparable to existing state-of-the-art approaches.
更多查看译文
关键词
3D reconstruction, CNN, MVS, Point cloud
AI 理解论文
溯源树
样例
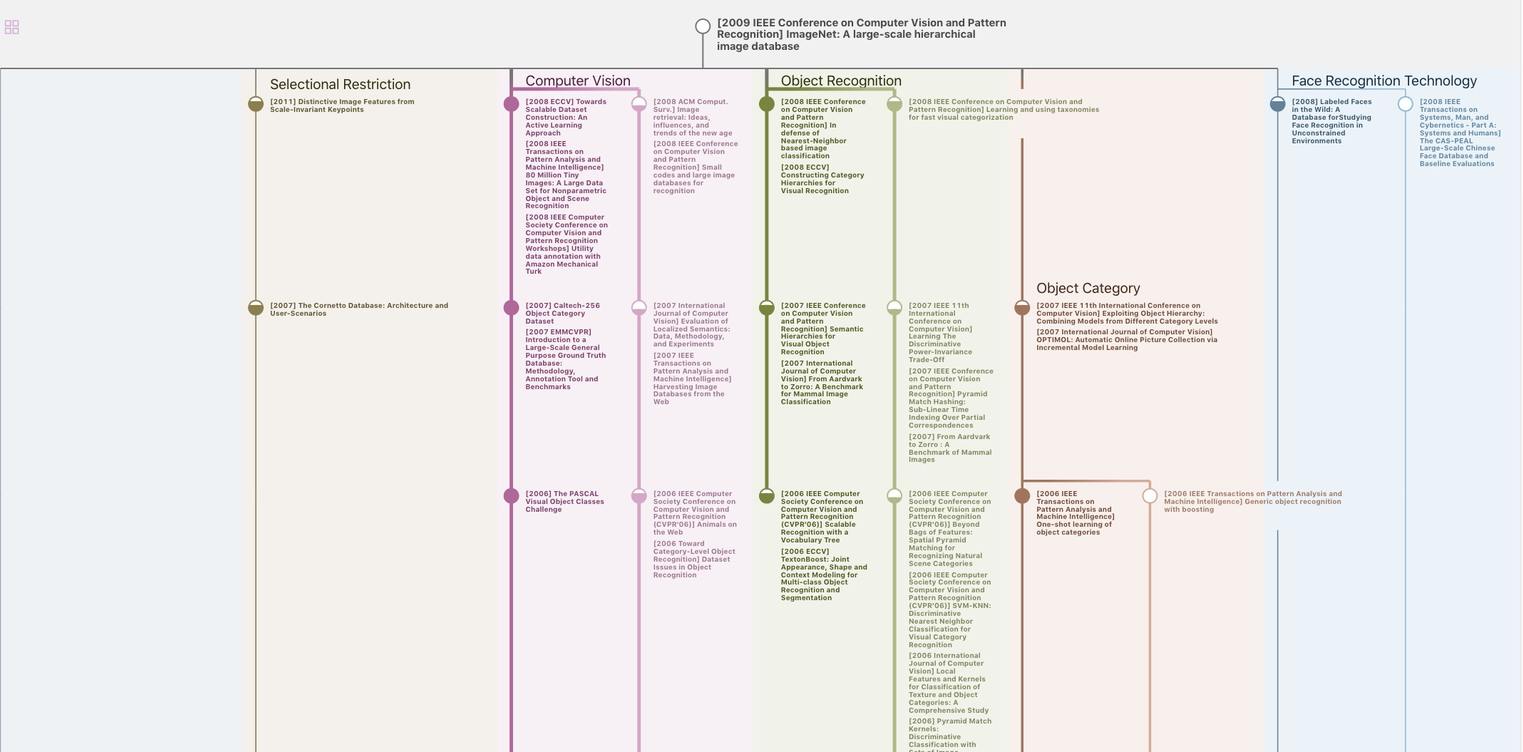
生成溯源树,研究论文发展脉络
Chat Paper
正在生成论文摘要