Tomographic Reconstruction Of Imaging Diagnostics With A Generative Adversarial Network
PLASMA AND FUSION RESEARCH(2019)
摘要
We have developed a tomographic reconstruction method using a conditional Generative Adversarial Network to obtain local-intensity profiles from imaging-diagnostic data. To train the network we prepared pairs of local-emissivity and line-integrated images that simulate the experimental system. After validating the accuracy of the trained network, we used it to reconstruct a local image from a measured line-integrated image. We applied this procedure to the He II-emission imaging diagnostic for RT-1 magnetospheric plasmas, including the effects of stray light within the measured image to remove reflections from the chamber walls in the reconstruction. The local intensity profiles we obtain clearly elucidate the effect of ion-cyclotron-resonance heating. This method is a powerful tool for systems where it is difficult to solve the inversion problem due to the involved contributions of nonlocal optical effects or measurement restrictions. (C) 2019 The Japan Society of Plasma Science and Nuclear Fusion Research
更多查看译文
关键词
deep learning, generative adversarial network, imaging diagnostic, tomographic reconstruction, laboratory magnetosphere
AI 理解论文
溯源树
样例
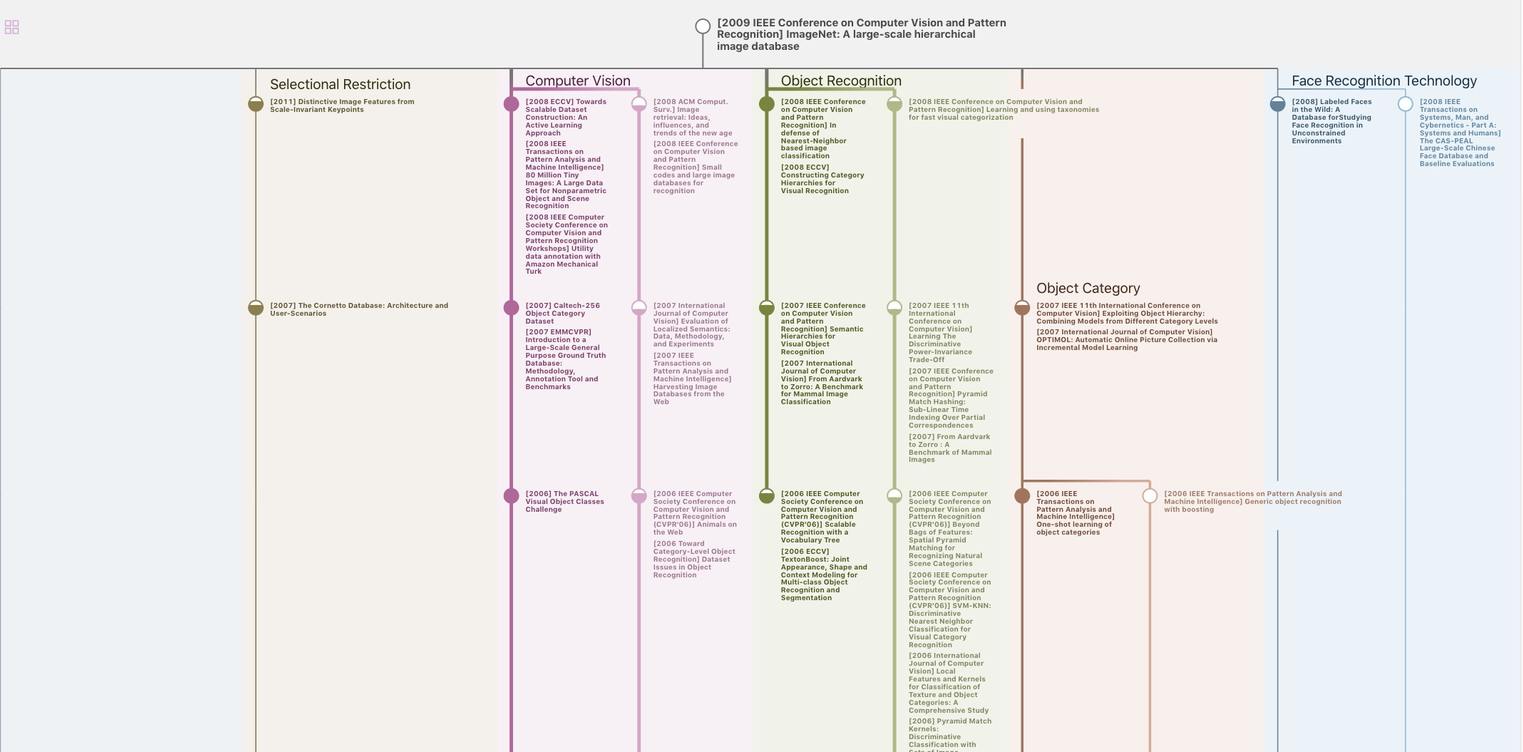
生成溯源树,研究论文发展脉络
Chat Paper
正在生成论文摘要