Fast and Scalable Approaches to Accelerate the Fuzzy k Nearest Neighbors Classifier for Big Data
IEEE Transactions on Fuzzy Systems(2020)
摘要
One of the best-known and most effective methods in supervised classification is the
k
-nearest neighbors algorithm (kNN). Several approaches have been proposed to improve its accuracy, where fuzzy approaches prove to be among the most successful, highlighting the classical fuzzy
k
-nearest neighbors (FkNN). However, these traditional algorithms fail to tackle the large amounts of data that are available today. There are multiple alternatives to enable kNN classification in big datasets, spotlighting the approximate version of kNN called hybrid spill tree. Nevertheless, the existing proposals of FkNN for big data problems are not fully scalable, because a high computational load is required to obtain the same behavior as the original FkNN algorithm. This article proposes global approximate hybrid spill tree FkNN and local hybrid spill tree FkNN, two approximate approaches that speed up runtime without losing quality in the classification process. The experimentation compares various FkNN approaches for big data with datasets of up to 11 million instances. The results show an improvement in runtime and accuracy over literature algorithms.
更多查看译文
关键词
Big Data,Runtime,Approximation algorithms,Proposals,Training,Scalability,Acceleration
AI 理解论文
溯源树
样例
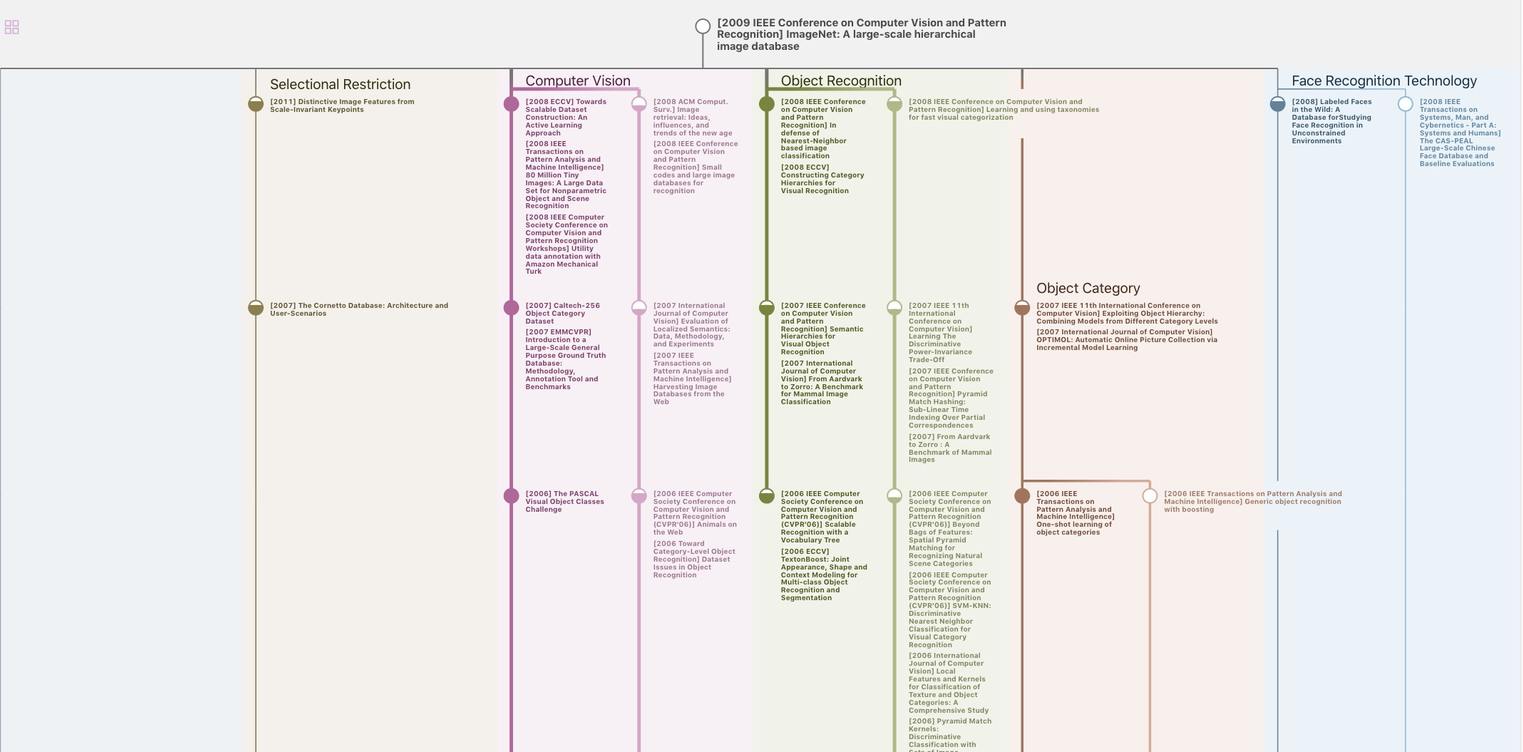
生成溯源树,研究论文发展脉络
Chat Paper
正在生成论文摘要