Integrated Gans: Semi-Supervised Sar Target Recognition
IEEE ACCESS(2019)
摘要
With the advantage of working in all weathers and all days, synthetic aperture radar (SAR) imaging systems have a great application value. As an efficient image generation and recognition model, generative adversarial networks (GANs) have been applied to SAR image analysis and achieved promising performance. However, the cost of labeling a large number of SAR images limits the performance of the developed approaches and aggravates the mode collapsing problem. This paper presents a novel approach namely Integrated GANs (I-GAN), which consists of a conditional GANs, an unconditional GANs and a classifier, to achieve semi-supervised generation and recognition simultaneously. The unconditional GANs assist the conditional GANs to increase the diversity of the generated images. A co-training method for the conditional GANs and the classifier is proposed to enrich the training samples. Since our model is capable of representing training images with rich characteristics, the classifier can achieve better recognition accuracy. Experiments on the Moving and Stationary Target Acquisition and Recognition (MSTAR) dataset proves that our method achieves better results in accuracy when labeled samples are insufficient, compared against other state-of-the-art techniques.
更多查看译文
关键词
Synthetic aperture radar (SAR), generative adversarial networks (GANs), semi-supervised learning, generation, recognition
AI 理解论文
溯源树
样例
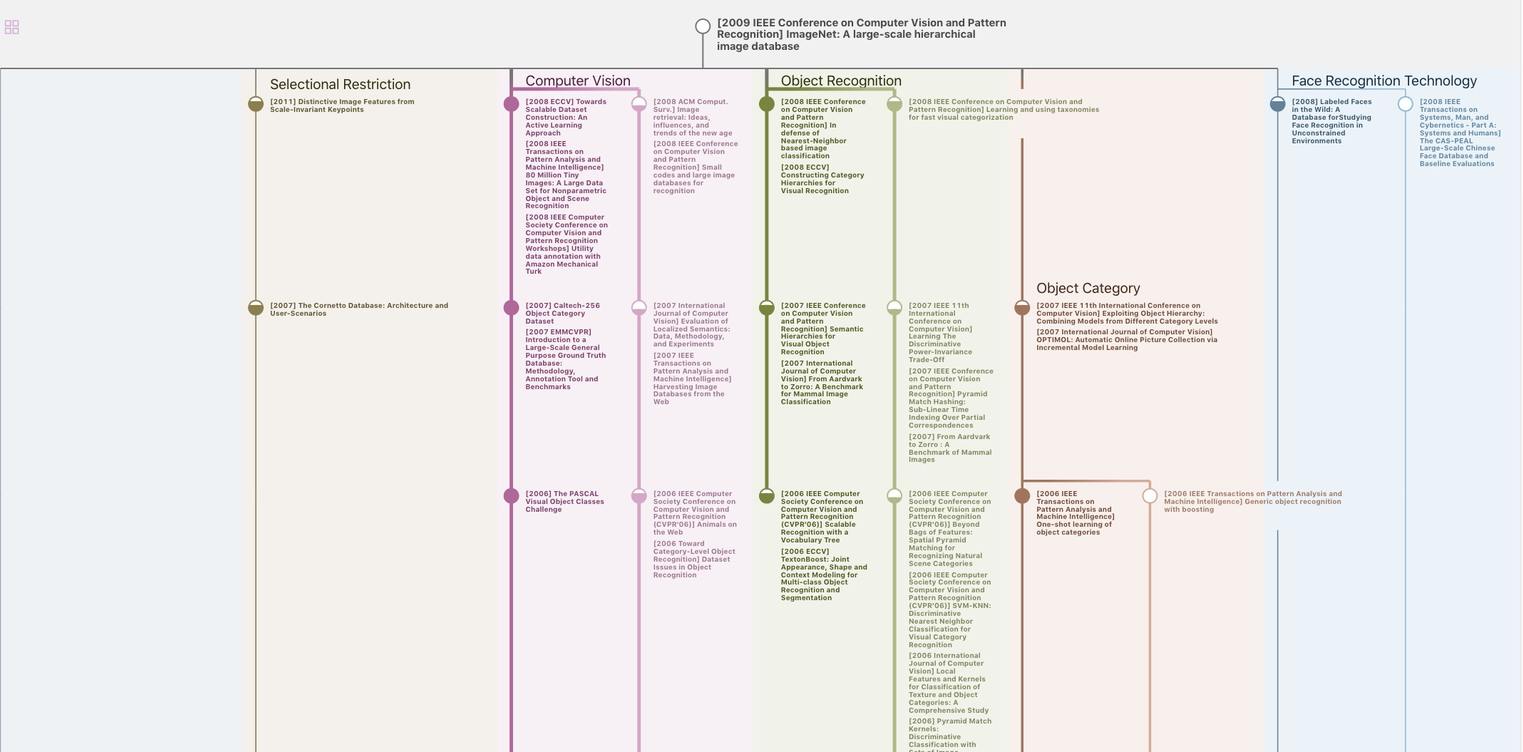
生成溯源树,研究论文发展脉络
Chat Paper
正在生成论文摘要