Discussion on dual–tree complex wavelet transform and generalized regression neural network based concentration-resolved fluorescence spectroscopy for oil identification
ANALYTICAL METHODS(2019)
摘要
There has been a growing concern in recent years about the increasing occurrence of oil spills into the environment and the proven toxic potential of these pollutants on human health and wildlife. Precisely and rapidly determining the sources of spilled oils can provide scientific evidence for the investigation and handling of spilled oil accidents. As traditional fluorescence spectroscopy detects in the linear concentration range, a concentration-resolved fluorescence spectroscopy (CRFS) is proposed in this paper, which introduces concentration as a new dimension. A data processing strategy combining multiple algorithms was applied to the CRFS for oil spill identification. Dual-tree complex wavelet transform (DTCWT) is used to extract multi-scale and multi-directional features of CRFS to ensure the accuracy of identification, while principal component analysis (PCA) is used to reduce the dimensions of the feature spectrum for the purpose of improving the identification speed. Three kinds of artificial neural networks (back propagation neural network (BP), probabilistic neural network (PNN), and generalized regression neural network (GRNN)), which are used as powerful classifiers for oil identification, were compared based on the spectral data processed by DTCWT and PCA. With 100% accuracy, GRNN was proved to be more suitable for oil classification and identification, especially for small sample sizes. The combination of the CRFS technique and this data processing strategy was revealed as a powerful methodology to differentiate a challenging sample set involving diesel (diesel 2002), fuel (heavy fuel 4#) and crude oils (Xia, Shang, Zhengqi), offering potential applications for use in real-time and economic oil fingerprint identification.
更多查看译文
AI 理解论文
溯源树
样例
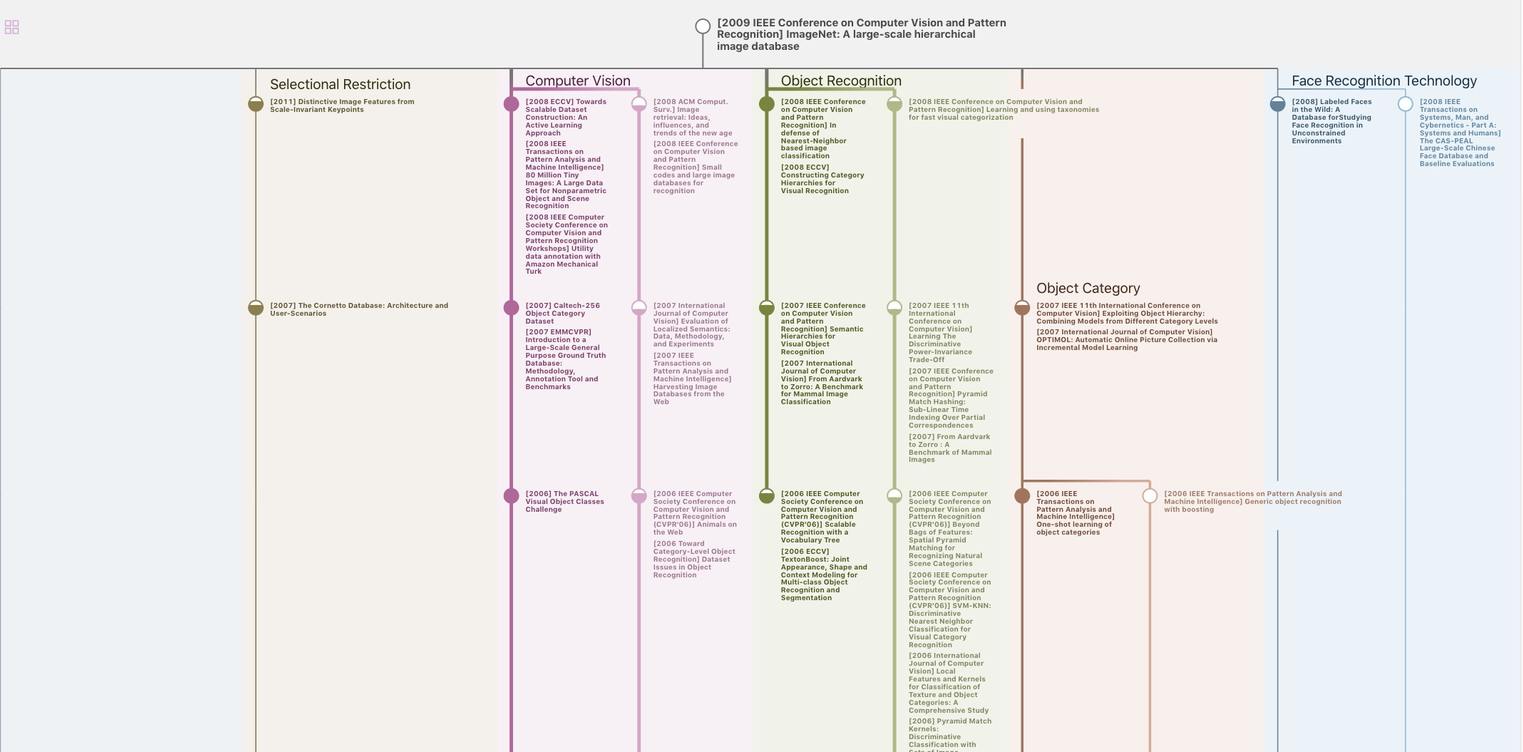
生成溯源树,研究论文发展脉络
Chat Paper
正在生成论文摘要