Ensemble Multiboost Based On Ripper Classifier For Prediction Of Imbalanced Software Defect Data
IEEE ACCESS(2019)
摘要
Identifying defective software entities is essential to ensure software quality during software development. However, the high dimensionality and class distribution imbalance of software defect data seriously affect software defect prediction performance. In order to solve this problem, this paper proposes an Ensemble MultiBoost based on RIPPER classifier for prediction of imbalanced Software Defect data, called EMR_SD. Firstly, the algorithm uses principal component analysis (PCA) method to find out the most effective features from the original features of the data set, so as to achieve the purpose of dimensionality reduction and redundancy removal. Furthermore, the combined sampling method of adaptive synthetic sampling (ADASYN) and random sampling without replacement is performed to solve the problem of data class imbalance. This classifier establishes association rules based on attributes and classes, using MultiBoost to reduce deviation and variance, so as to achieve the purpose of reducing classification error. The proposed prediction model is evaluated experimentally on the NASA MDP public datasets and compared with existing similar algorithms. The results show that EMR_SD algorithm is superior to DNC, CEL and other defect prediction techniques in most evaluation indicators, which proves the effectiveness of the algorithm.
更多查看译文
关键词
Software defect prediction, class imbalance, combined sampling, rule learning, MultiBoost
AI 理解论文
溯源树
样例
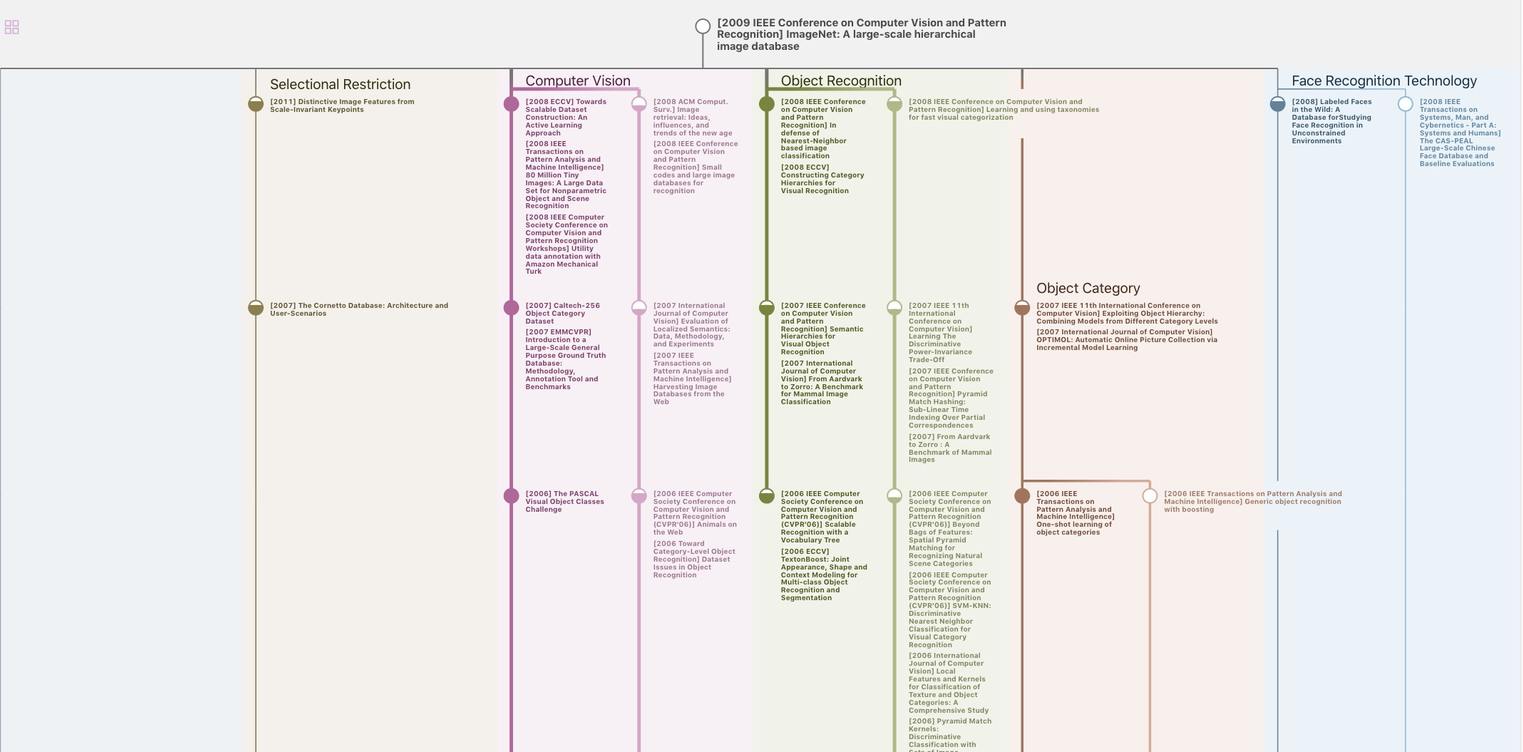
生成溯源树,研究论文发展脉络
Chat Paper
正在生成论文摘要