An efficient method for pruning redundant negative and positive association rules
Neurocomputing(2020)
摘要
One of the most important problems that occur when mining positive and negative association rules (PNARs) is that the number of mined PNARs is usually large, which increases the difficulties that users retrieve decision-information. Those methods that prune redundant positive association rules (PARs) don’t work when negative association rules (NARs) are taken into consideration. So in this paper, we first analyze what kinds of PNARs are redundant and then propose a novel method called LOGIC by using logical reasoning to prune redundant PNARs. In addition, we combine correlation coefficient and multiple minimum confidences (mc) to ensure that the mined PNARs are strongly correlated and their number can be flexibly controlled. Experimental results show that our method can prune up to 81.6% redundant PNARs. To the best of our knowledge, LOGIC is the first method to prune redundant PNARs simultaneously.
更多查看译文
关键词
Correlation coefficient,Multiple minimum confidence,Negative association rules,Redundant negative association rules
AI 理解论文
溯源树
样例
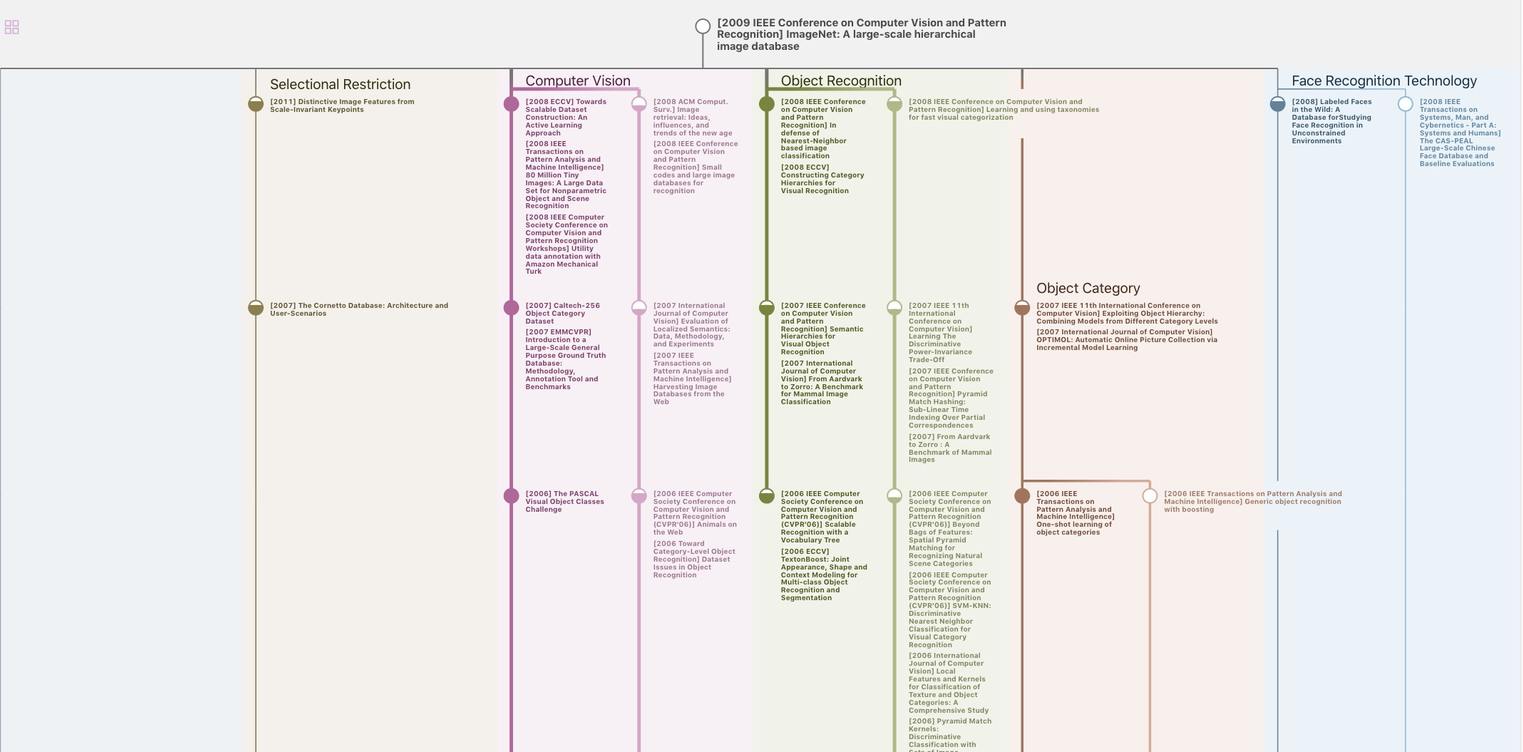
生成溯源树,研究论文发展脉络
Chat Paper
正在生成论文摘要