Adaptive Multilayered Dictionary Learning For Compressive-Sensing-Based Image Reconstruction
IEEE ACCESS(2019)
摘要
The accuracy of compressive-sensing-based image reconstruction largely depends on the performance of dictionaries used for sparse representation. Dictionaries are frequently obtained through dictionary learning (DL) process by using a set of training samples. However, conventional DL methods are limited by two factors. First, the sparse level for each training sample is fixed, which may lead under-fitting or over-fitting of sparse representation of a sample. Second, only the features of original samples are used for training dictionary. In fact, the objective samples will become different after removing the representation of one or more selected atoms during the DL process, yielding implicit features. Unfortunately, these features cannot be utilized by conventional DL methods. To overcome the two limitations, we propose a novel DL scheme named adaptive multilayered DL (AMDL) by dividing the sparse representation into several layers. The number of atoms selected for each layer is determined adaptively based on the correlation between atoms and residuals. Hence, the features of different layers are utilized and the under-fitting or over-fitting of sparse coding can be reduced. The proposed scheme can be employed to improve exiting dictionary learning methods, such as the method of direction (MOD), the k-singular value decomposition (K-SVD), and the online DL (ODL). Experimental results demonstrate that the proposed scheme could provide more accurate sparse representation for compressive-sensing-based image reconstruction, compared to the standard DL scheme.
更多查看译文
关键词
Compressive sensing, dictionary learning, sparse representation, image processing
AI 理解论文
溯源树
样例
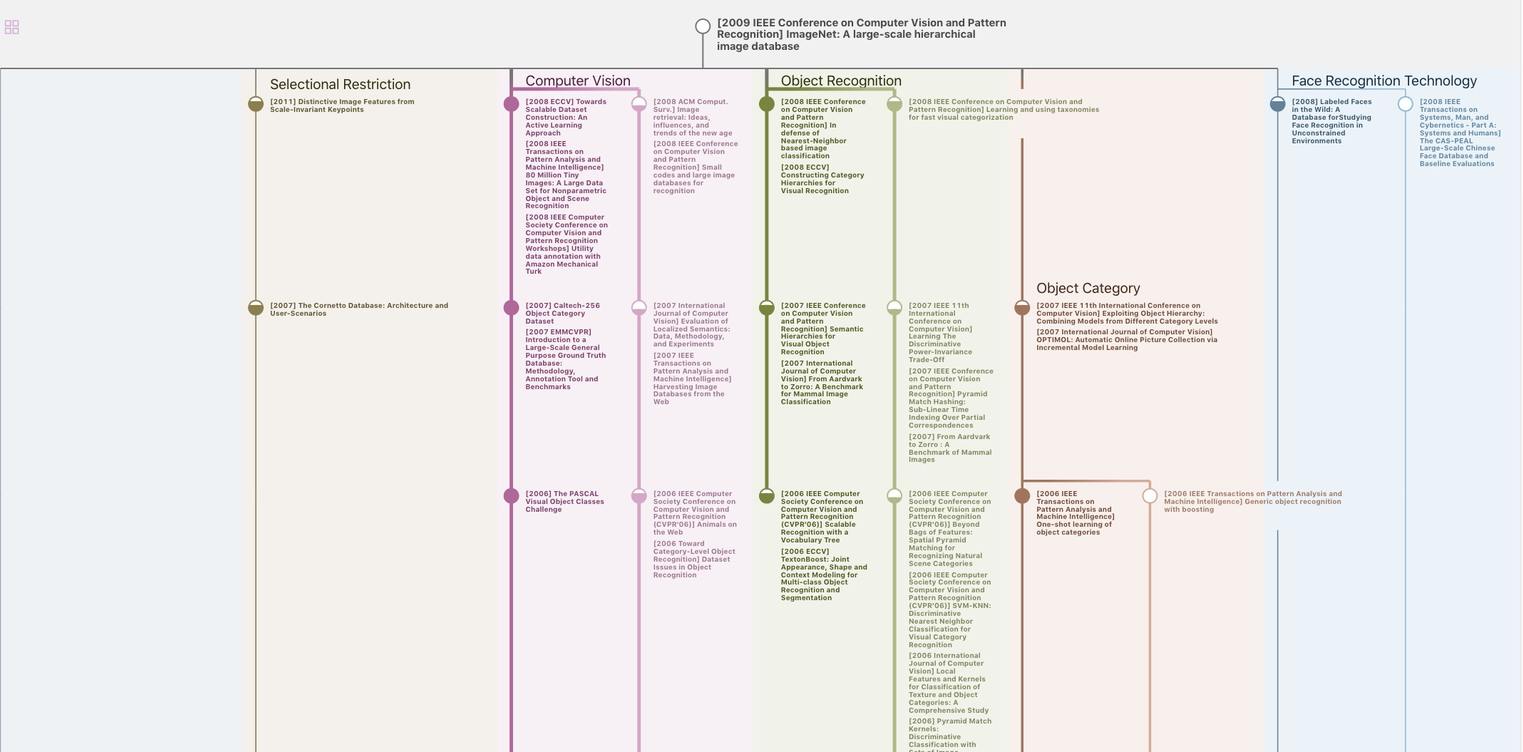
生成溯源树,研究论文发展脉络
Chat Paper
正在生成论文摘要