Speech Signal Recovery Using Block Sparse Bayesian Learning
Arabian Journal for Science and Engineering(2019)
摘要
Compressed sensing is based on the recovery of original signal from the low-quality and incomplete samples. Recently, ℓ _1 -norm is used for the estimation of signal elements from the underdetermined set of equations. In this paper, we propose a technique for speech signal recovery called block sparse Bayesian learning. The proposed technique is applied over the random set of speech samples and acquired better performance as compared to ℓ _1 -based recovery. Apart from the proposed recovery technique, this work is also intended to develop a trained and efficient sampling matrix through offline training. In this work, we apply structural similarity index as a metric to compare the performance of the proposed technique with an existing ℓ _1 based recovery. Sparse Bayesian learning and ℓ _1 -norm recovery are applied over the selected audio files from the datasets. The dataset consists of speech signals from three different languages: Urdu, Pashto and English. Structural similarity between the recovered and original speech signals is used as a metric to compare the performance of BSBL with ℓ _1 -norm minimization. The comparison based on structural similarity index shows the effectiveness of the proposed technique.
更多查看译文
关键词
Compressed sensing, BSBL, SSIM, Signal recovery, Wavelet denoising
AI 理解论文
溯源树
样例
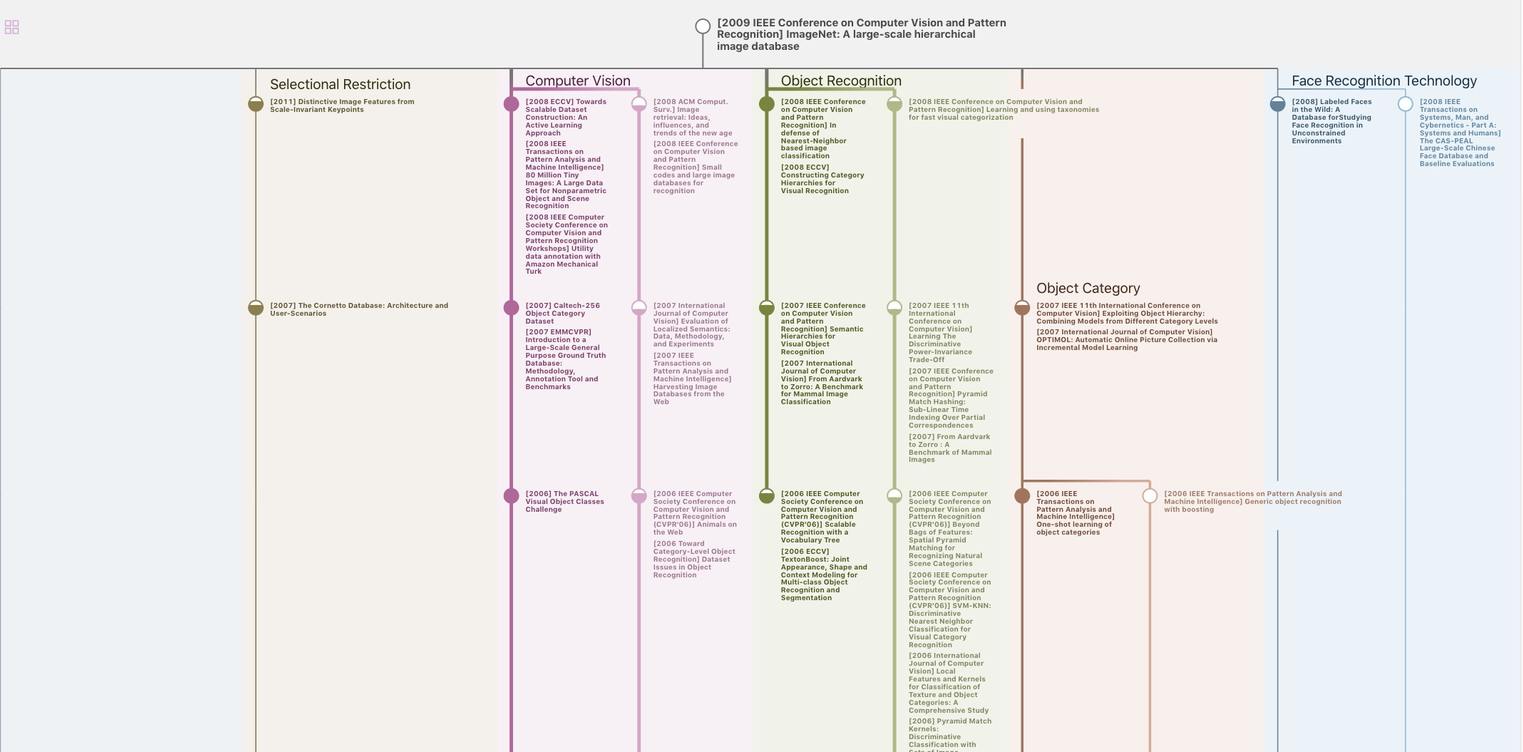
生成溯源树,研究论文发展脉络
Chat Paper
正在生成论文摘要