Online Dictionary Learning For Sparse Representation-Based Classification Of Motor Imagery Eeg
2019 27TH IRANIAN CONFERENCE ON ELECTRICAL ENGINEERING (ICEE 2019)(2019)
摘要
The use of Motor Imagery (MI) EEG signals in implementation of Brain-Computer Interfaces (BCIs) has been increased during recent years. Simpler interaction of users with these types of interfaces in comparison with other interfaces like BCI systems based on steady state visually evoked potential (SSVEP) as well as comprehensiveness of motor-imagery-based interfaces are the most important reasons of this popularity. In this paper, we propose to use Sparse Representation-based Classification (SRC) method for MI-EEG based BCI. In order to obtain more accurate sparse representation of the underlying EEG signal, we used dictionary learning approach instead of deterministic dictionary structures which leads to better classification performance. Therefore, we have used Correlation-Based Least Squares Update (CBLSU) in order to obtain an online dictionary learning scheme which is more suitable for BCI systems in terms of computational complexity. It is demonstrated that the proposed method shows better performance in comparison with the existing methods which more commonly use linear classifiers like LDA and SVM. The mean accuracy of 84.79 (SD = 8.51) is obtained for five subjects of dataset IVa from BCI Competition III database, which shows considerable improvement compared to the existing methods.
更多查看译文
关键词
Brain-Computer Interface (BCI), Motor Imagery (MI), electroencephalography (EEG), Sparse Representation-based Classification (SRC), Common Spatial Pattern (CSP), Online Dictionary Learning
AI 理解论文
溯源树
样例
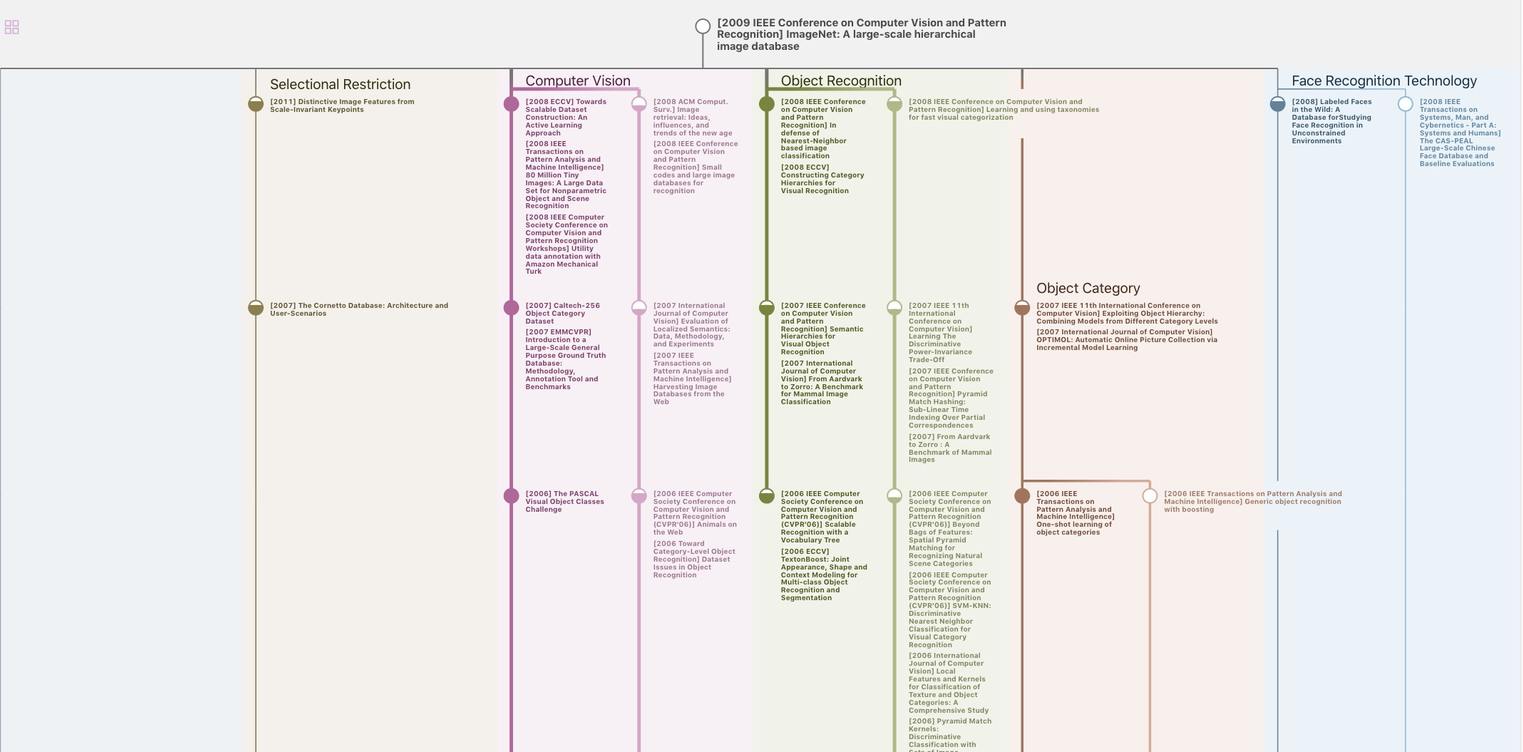
生成溯源树,研究论文发展脉络
Chat Paper
正在生成论文摘要