Memory, attention and prediction: a deep learning architecture for car-following
TRANSPORTMETRICA B-TRANSPORT DYNAMICS(2019)
摘要
Car-following (CF) models are an appealing research area because they fundamentally describe the longitudinal interactions of vehicles and contribute substantially to an understanding of traffic flow. In this study, by combining numerous deep neural network structures to mimic the memory, attention and prediction (MAP) mechanisms of real drivers, a deep learning-based car following model named MAP is built. The proposed MAP learned CF behaviour from real-world datasets. Experiments on predicting future driving behaviour show that the MAP model with memory, attention and prediction mechanisms outperforms models without those mechanisms. Several analysis are conducted to understand the MAP model, and quantitatively provides some explanations of how MAP achieves the CF behaviour. A simulation is also presented and explicitly analysed. The results show that the proposed approach can produce space-time diagrams similar to real traffic. The analysis also shows that the supervised learning models generate the most likely, rather than the best, CF behaviour.
更多查看译文
关键词
Car following models,deep neural networks,attention models,memory mechanism
AI 理解论文
溯源树
样例
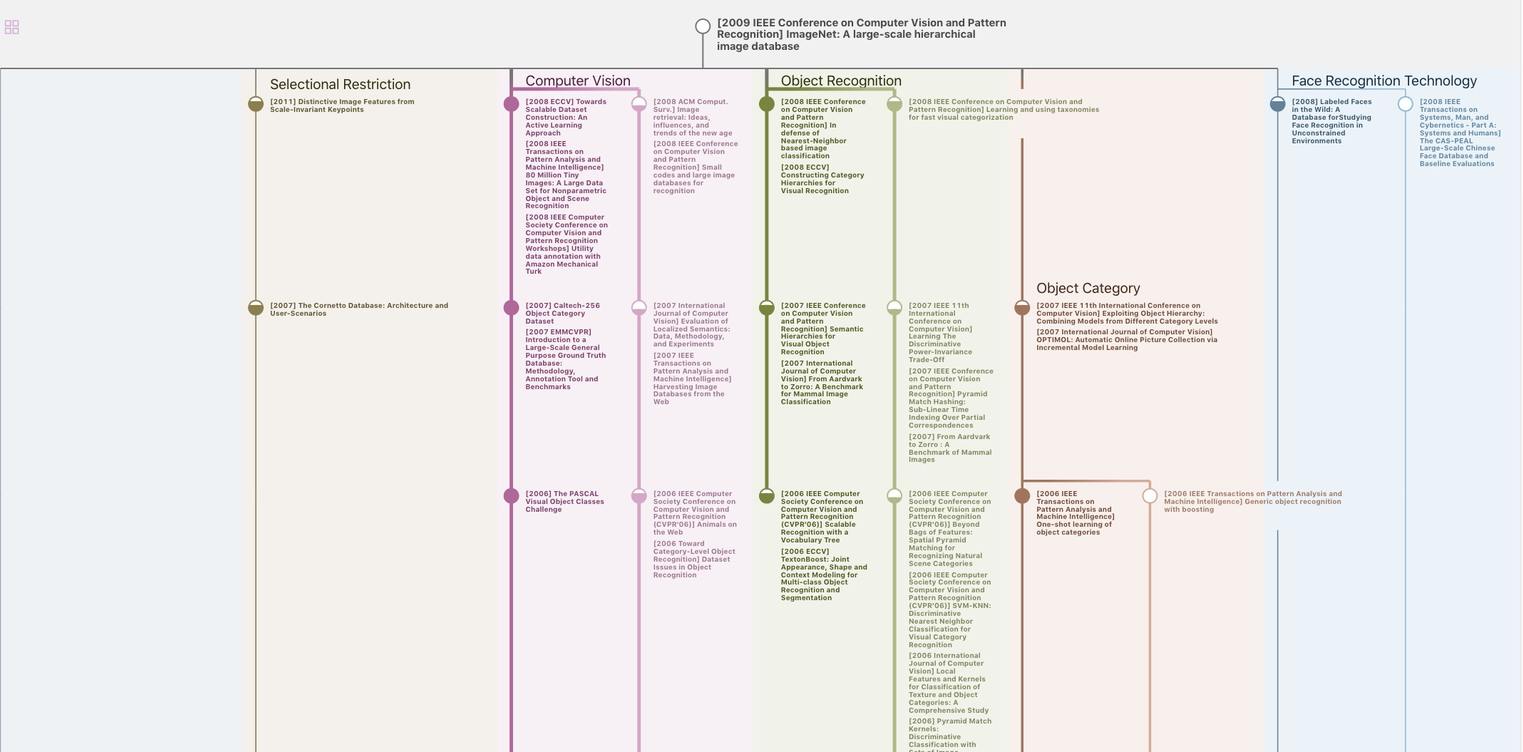
生成溯源树,研究论文发展脉络
Chat Paper
正在生成论文摘要