Cloud Detection Of Rgb Color Remote Sensing Images Based On Improved M-Net
LASER & OPTOELECTRONICS PROGRESS(2019)
摘要
Cloud detection is prone to error and considerable loss of details because clouds do not have obvious color distribution and texture pattern in RGB color images. Therefore, this study proposes an improved M-Net model called the RM-Net model to achieve end-to-end pixel-level semantic segmentation. An original dataset is enhanced and a corresponding pixel-level label is marked. Multi-scale image features are extracted without losing data via atrous spatial pyramid pooling, and residual units are combined to make the network resistant to degradation. Global context informations of the images are extracted using the encoder module and the left path. The spatial resolutions of the images are restored using the decoder module and the right path. Each pixel's category probability is determined based on fused features, and pixel-level cloud and non-cloud segmentation are performed using the input classifier. When training and testing Landsat8 and GaoFen-1 WFV RGB color images, experimental results show that the proposed method can well detect cloud edge details under various conditions and achieve high-precision cloud shadow detection, thus demonstrating that the proposed method has high generalization and robustness.
更多查看译文
关键词
image processing, cloud detection, remote sensing images, M-Net, atrous spatial pyramid pooling, residual units, cloud shadow detection
AI 理解论文
溯源树
样例
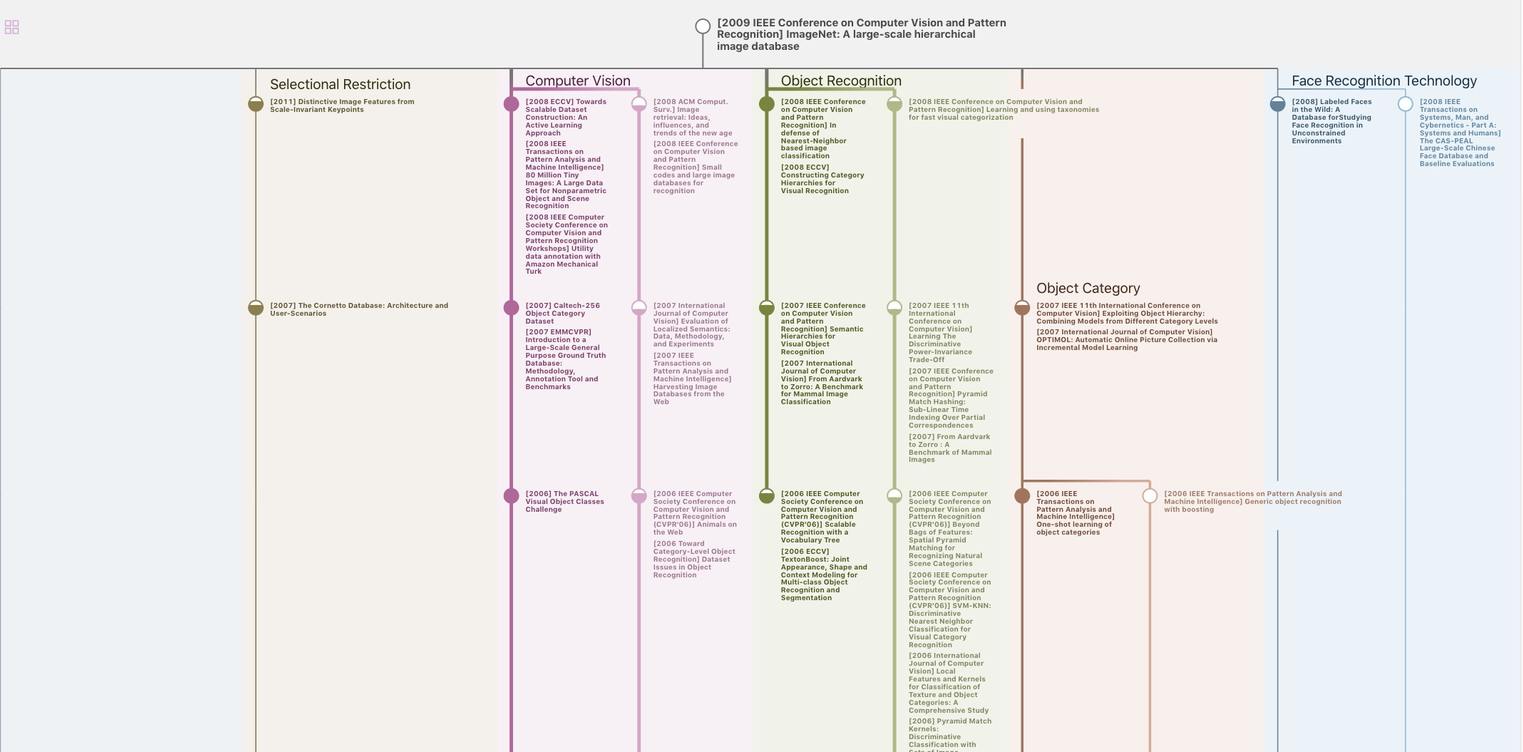
生成溯源树,研究论文发展脉络
Chat Paper
正在生成论文摘要