Multi-Scale Densely Connected Dehazing Network
INTELLIGENT ROBOTICS AND APPLICATIONS, ICIRA 2019, PT IV(2019)
摘要
Single image dehazing is a challenging ill-posed problem. The traditional methods mainly focus on estimating the transmission of atmospheric-light medium with some priors or constraints. In this paper, we propose a novel end-to-end convolutional neural network (CNN) for image dehazing, called multi-scale densely connected dehazing network (MDCDN). The proposed network consists of a parallel multi-scale densely connected CNN network and an encoder-decoder U net. The parallel multi-scale dense-net can estimate transmission map accurately. The encoder-decoder U net is used to estimate the atmospheric light intensity. The all-in-one training can jointly learn the transmission map, atmospheric light, and dehazing images all together with jointly MSE error and a discriminator loss. We also create a dataset with indoor and outdoor data based on the LFSD, NLPR, and NYU2 depth datasets to train our network. Extensive experiments demonstrate that, in most cases, the proposed method achieves significant improvements over the state-of-the-art methods.
更多查看译文
关键词
Deep learning image dehazing, Multi-scale dense network, One-in-all training, Large-scale dataset
AI 理解论文
溯源树
样例
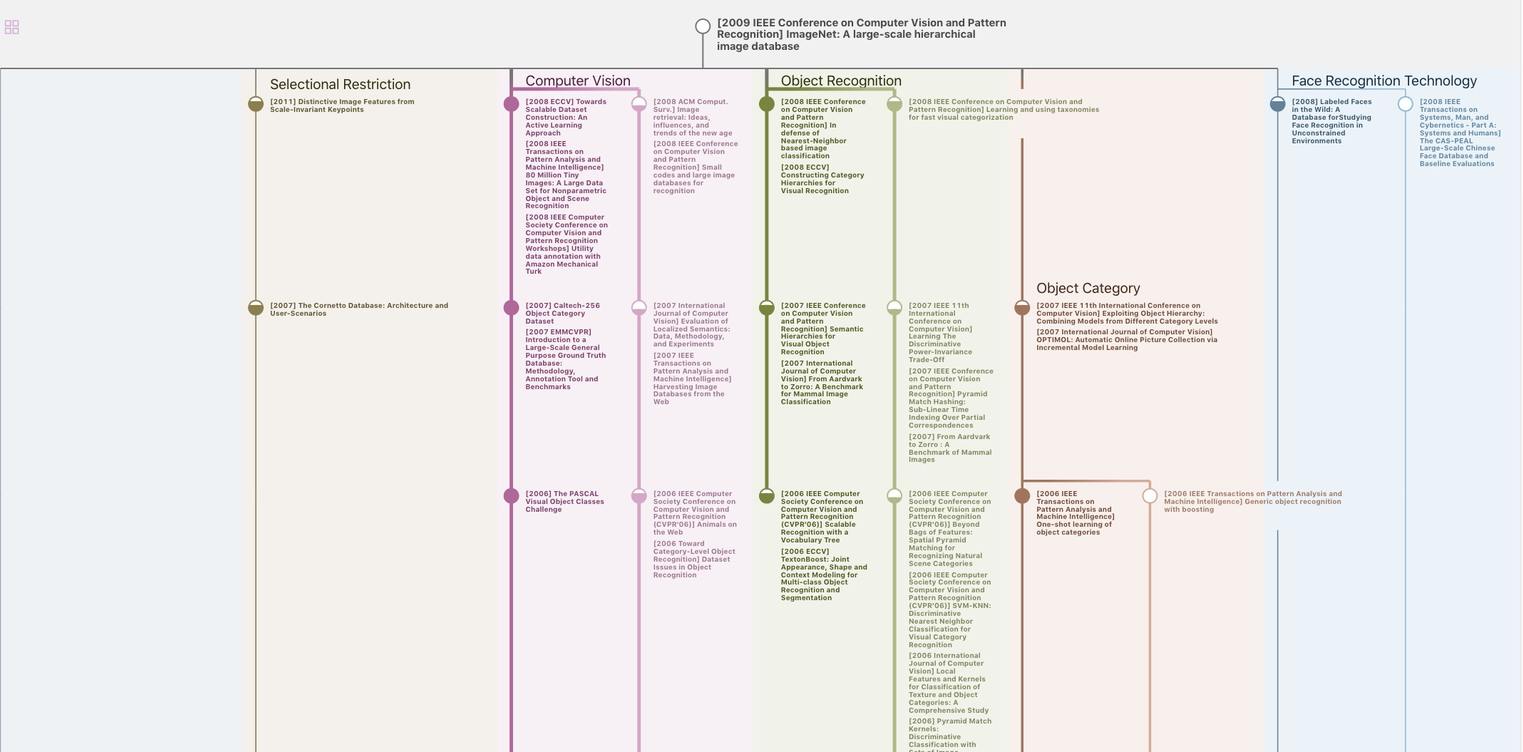
生成溯源树,研究论文发展脉络
Chat Paper
正在生成论文摘要