Observer-Based Adaptive Neural Tracking Control For A Class Of Stochastic Nonlinear Systems
INTERNATIONAL JOURNAL OF CONTROL(2021)
摘要
In this paper, an observer-based adaptive neural tracking control approach is proposed for a class of stochastic nonlinear systems with immeasurable states. The radial basis function neural networks (RBFNNs) are used to approximate the unknown nonlinear functions, and a linear reduced-order state observer is designed for estimating the unmeasured states. Based on the designed the state observer, an adaptive neural output feedback control approach is developed via backstepping control design. It is shown that the proposed controller ensures that all signals of the closed-loop system remain bounded in probability, and the tracking error converges to an arbitrarily small neighbourhood around the origin in the sense of mean quartic value. Finally, two examples are given to illustrate the effectiveness of the proposed design approach.
更多查看译文
关键词
Stochastic nonlinear systems, neural networks, reduced-order state observer, adaptive tracking control, output feedback
AI 理解论文
溯源树
样例
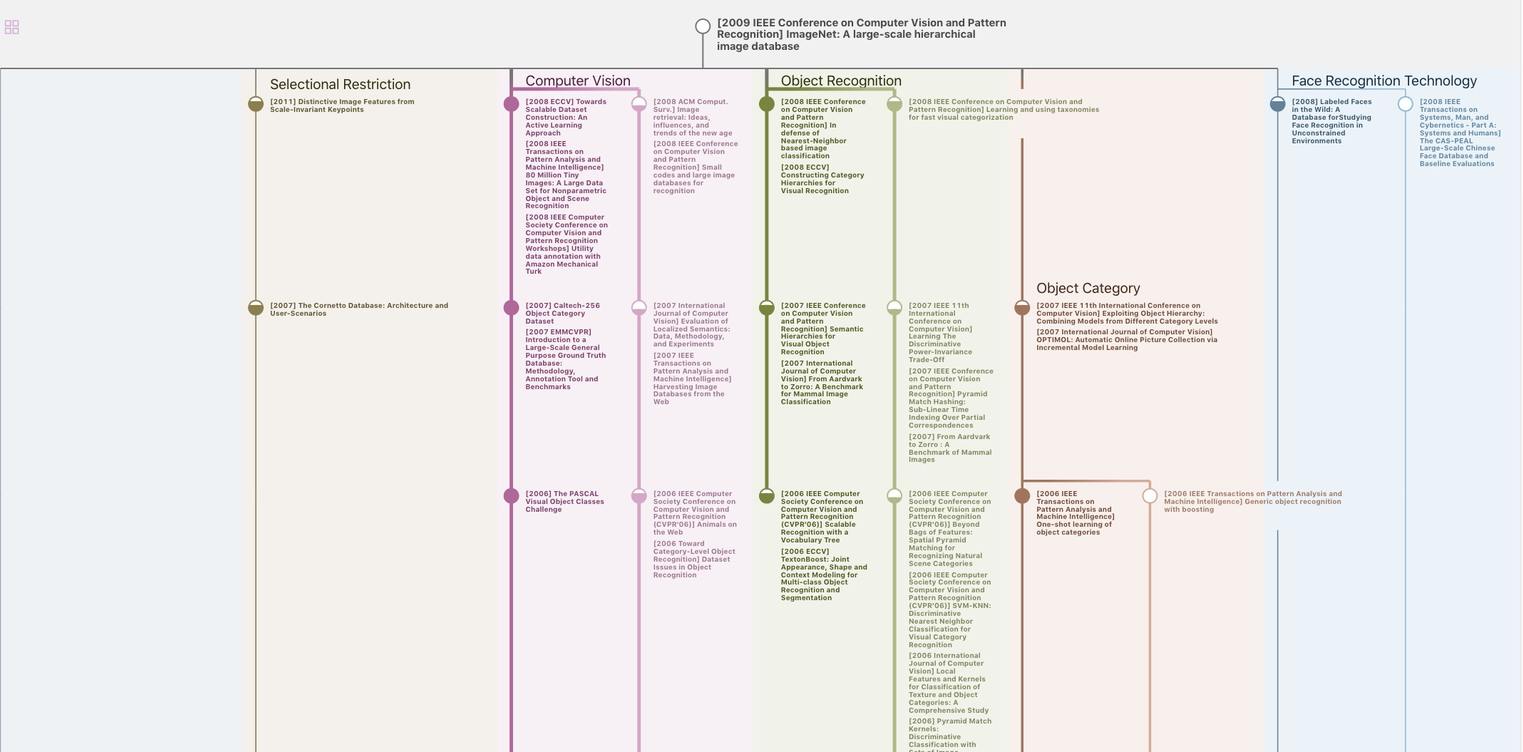
生成溯源树,研究论文发展脉络
Chat Paper
正在生成论文摘要