Online Stochastic Gradient Descent with Arbitrary Initialization Solves Non-smooth, Non-convex Phase Retrieval
JOURNAL OF MACHINE LEARNING RESEARCH(2023)
摘要
In recent literature, a general two step procedure has been formulated for solving the problem of phase retrieval. First, a spectral technique is used to obtain a constant-error initial estimate, following which, the estimate is refined to arbitrary precision by first -order optimization of a non-convex loss function. Numerical experiments, however, seem to suggest that simply running the iterative schemes from a random initialization may also lead to convergence, albeit at the cost of slightly higher sample complexity. In this paper, we prove that, in fact, constant step size online stochastic gradient descent (SGD) converges from arbitrary initializations for the non-smooth, non-convex amplitude squared loss objective. In this setting, online SGD is also equivalent to the randomized Kaczmarz algorithm from numerical analysis. Our analysis can easily be generalized to other single index models. It also makes use of new ideas from stochastic process theory, including the notion of a summary state space, which we believe will be of use for the broader field of non-convex optimization.
更多查看译文
关键词
Phase retrieval,SGD,non -convex,random initialization,optimization,polynomial time convergence.
AI 理解论文
溯源树
样例
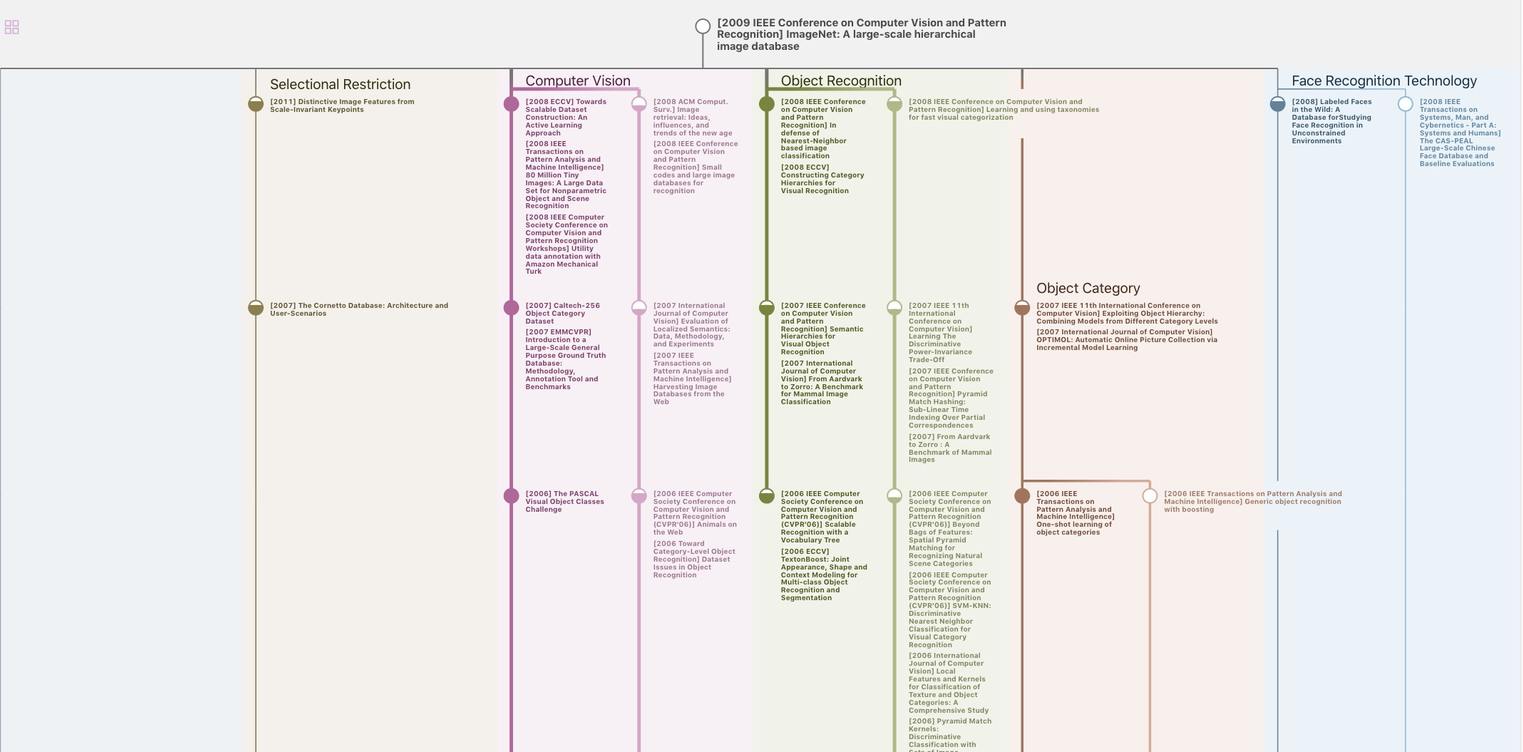
生成溯源树,研究论文发展脉络
Chat Paper
正在生成论文摘要