Limiting Behavior of Largest Entry of Random Tensor Constructed by High-Dimensional Data
Journal of Theoretical Probability(2019)
摘要
Let X_k=(x_k1, … , x_kp)', k=1,… ,n , be a random sample of size n coming from a p -dimensional population. For a fixed integer m≥ 2 , consider a hypercubic random tensor 𝐓 of m th order and rank n with 𝐓= ∑ _k=1^nX_k⊗⋯⊗X_k_multiplicity m= (∑ _k=1^n x_ki_1x_ki_2⋯ x_ki_m )_1≤ i_1,… , i_m≤ p. Let W_n be the largest off-diagonal entry of 𝐓 . We derive the asymptotic distribution of W_n under a suitable normalization for two cases. They are the ultra-high-dimension case with p→∞ and log p=o(n^β) and the high-dimension case with p→∞ and p=O(n^α) where α ,β >0 . The normalizing constant of W_n depends on m and the limiting distribution of W_n is a Gumbel-type distribution involved with parameter m .
更多查看译文
关键词
Tensor,Extreme-value distribution,High-dimensional data,Stein–Chen Poisson approximation
AI 理解论文
溯源树
样例
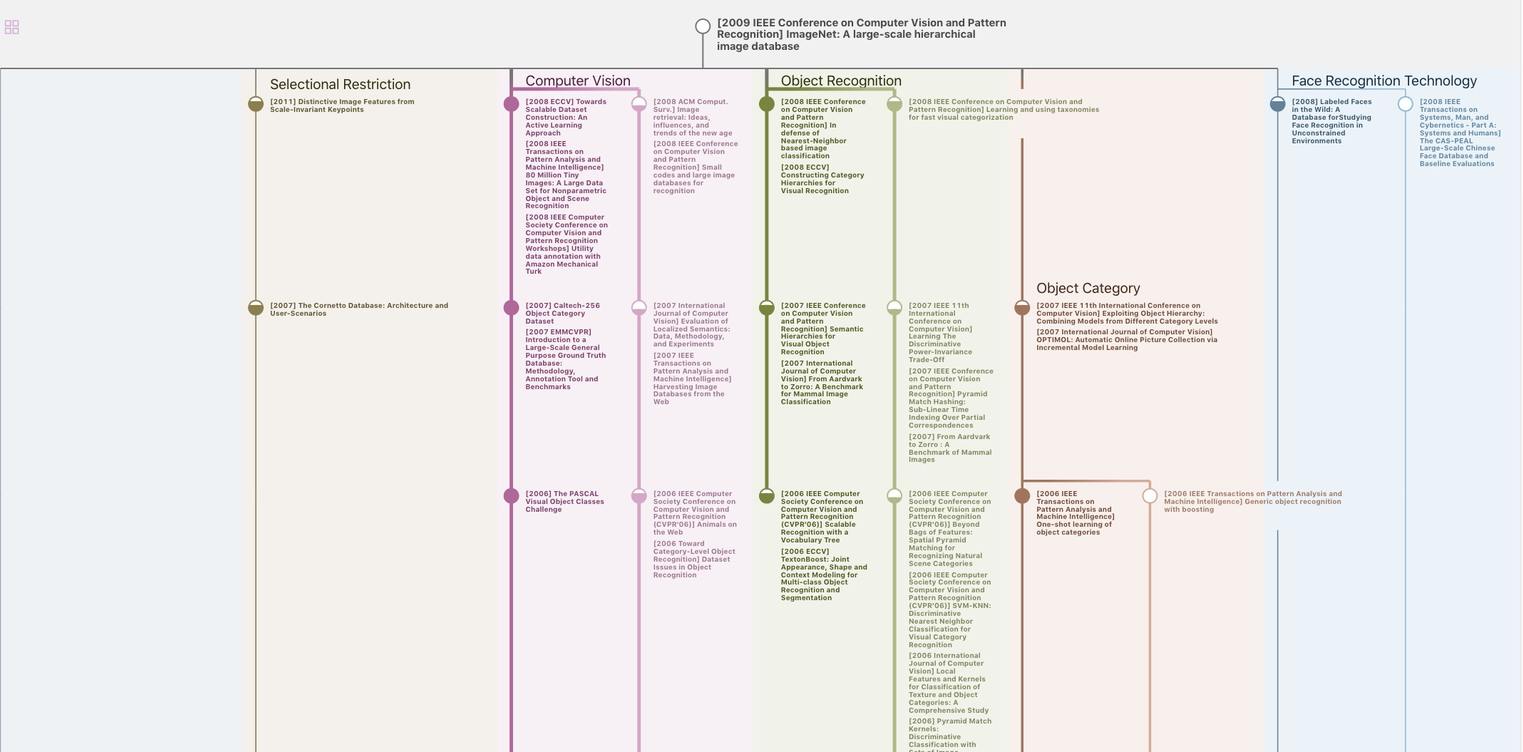
生成溯源树,研究论文发展脉络
Chat Paper
正在生成论文摘要