A Bin Encoding Training of a Spiking Neural Network-based Voice Activity Detection
arxiv(2019)
摘要
Advances of deep learning for Artificial Neural Networks(ANNs) have led to significant improvements in the performance of digital signal processing systems implemented on digital chips. Although recent progress in low-power chips is remarkable, neuromorphic chips that run Spiking Neural Networks (SNNs) based applications offer an even lower power consumption, as a consequence of the ensuing sparse spike-based coding scheme. In this work, we develop a SNN-based Voice Activity Detection (VAD) system that belongs to the building blocks of any audio and speech processing system. We propose to use the bin encoding, a novel method to convert log mel filterbank bins of single-time frames into spike patterns. We integrate the proposed scheme in a bilayer spiking architecture which was evaluated on the QUT-NOISE-TIMIT corpus. Our approach shows that SNNs enable an ultra low-power implementation of a VAD classifier that consumes only 3.8$\mu$W, while achieving state-of-the-art performance.
更多查看译文
关键词
spiking neural networks, voice activity detection, bin encoding, supervised learning
AI 理解论文
溯源树
样例
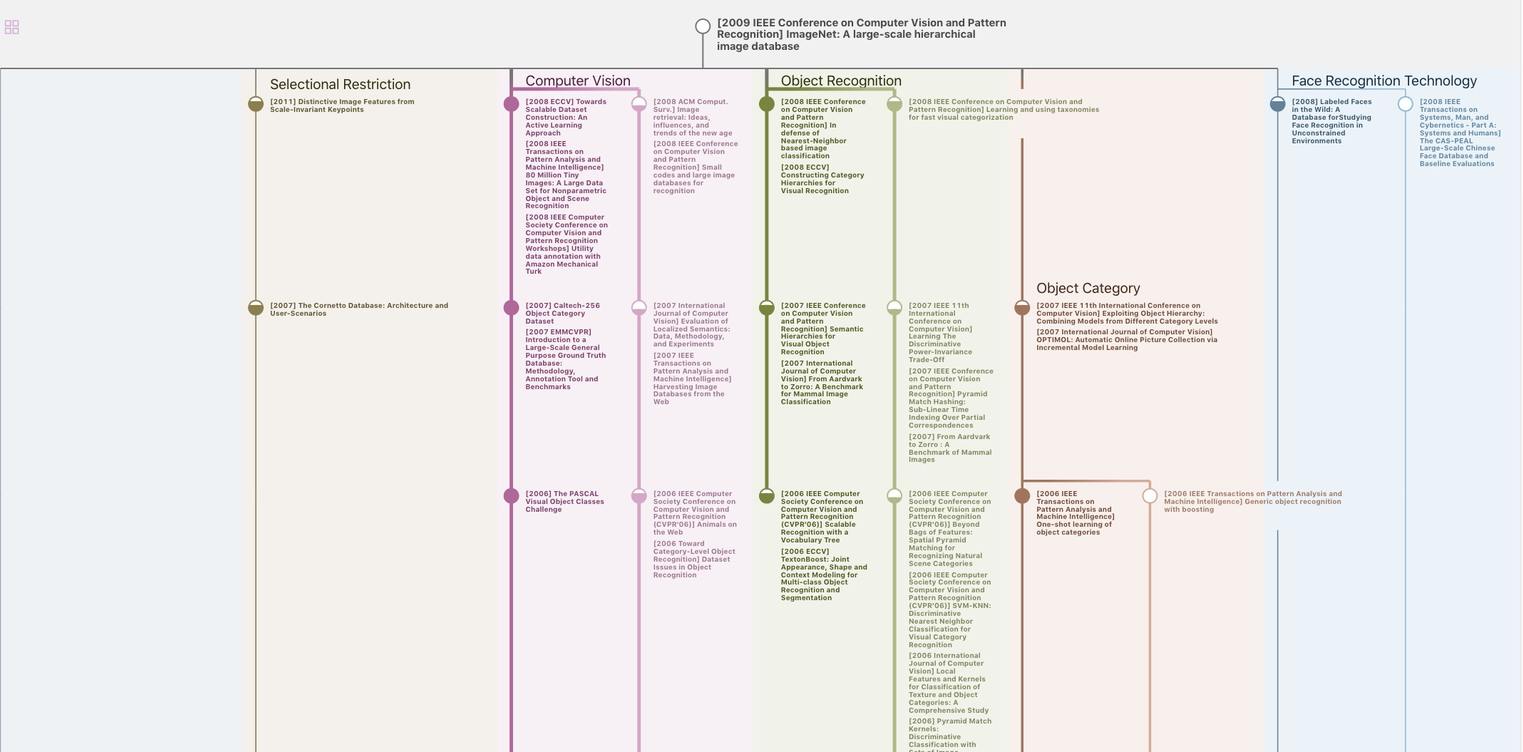
生成溯源树,研究论文发展脉络
Chat Paper
正在生成论文摘要