Multilevel dimension-independent likelihood-informed MCMC for large-scale inverse problems
INVERSE PROBLEMS(2024)
摘要
We present a non-trivial integration of dimension-independent likelihood-informed (DILI) MCMC (Cui et al 2016) and the multilevel MCMC (Dodwell et al 2015) to explore the hierarchy of posterior distributions. This integration offers several advantages: First, DILI-MCMC employs an intrinsic likelihood-informed subspace (LIS) (Cui et al 2014)-which involves a number of forward and adjoint model simulations-to design accelerated operator-weighted proposals. By exploiting the multilevel structure of the discretised parameters and discretised forward models, we design a Rayleigh-Ritz procedure to significantly reduce the computational effort in building the LIS and operating with DILI proposals. Second, the resulting DILI-MCMC can drastically improve the sampling efficiency of MCMC at each level, and hence reduce the integration error of the multilevel algorithm for fixed CPU time. Numerical results confirm the improved computational efficiency of the multilevel DILI approach.
更多查看译文
关键词
multilevel Monte Carlo,likelihood-informed subspaces,dimension-independent MCMC,inverse problems
AI 理解论文
溯源树
样例
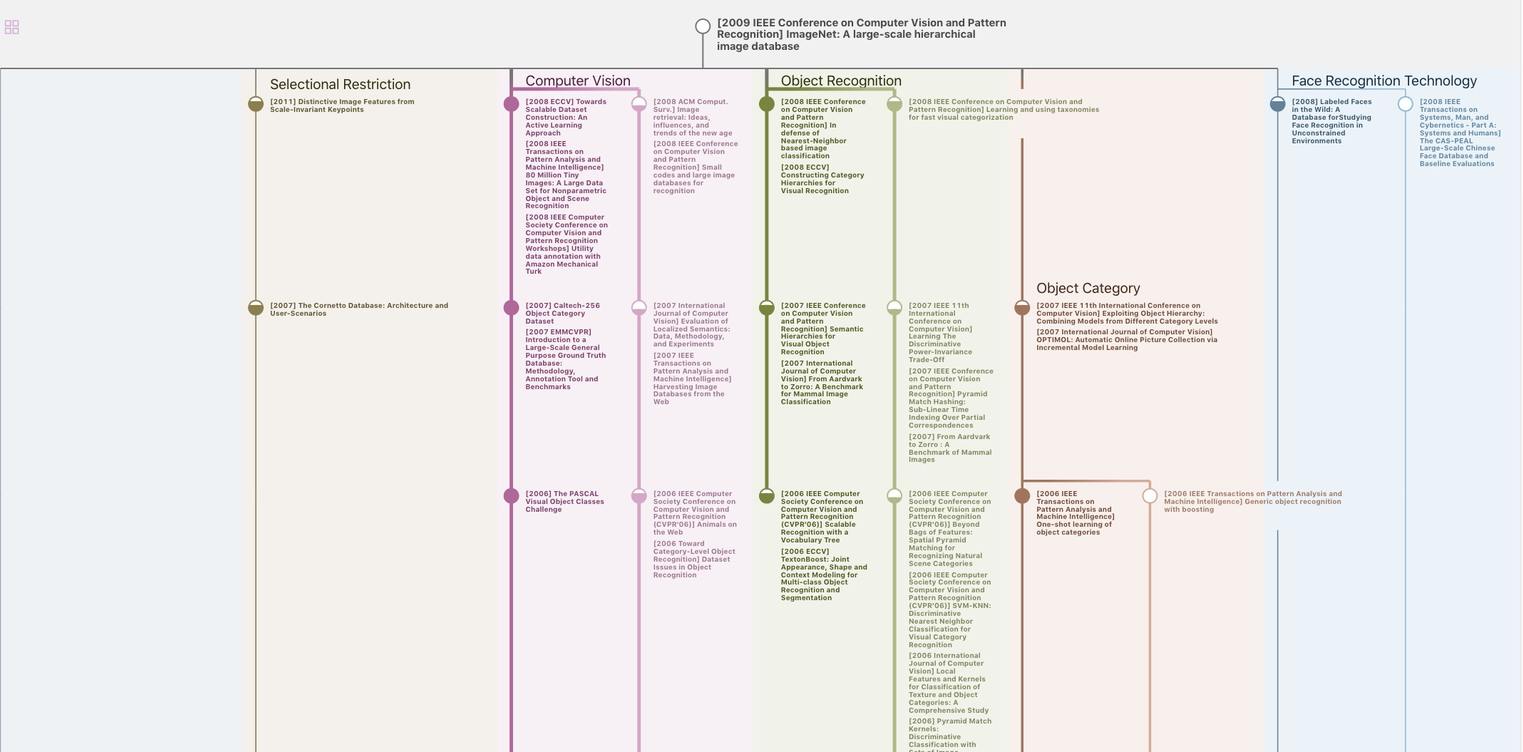
生成溯源树,研究论文发展脉络
Chat Paper
正在生成论文摘要