Non-Local Convlstm For Video Compression Artifact Reduction
2019 IEEE/CVF INTERNATIONAL CONFERENCE ON COMPUTER VISION (ICCV 2019)(2019)
摘要
Video compression artifact reduction aims to recover high-quality videos from low-quality compressed videos. Most existing approaches use a single neighboring frame or a pair of neighboring frames (preceding and/or following the target frame) for this task. Furthermore, as frames of high quality overall may contain low-quality patches, and high-quality patches may exist in frames of low quality overall, current methods focusing on nearby peak-quality frames (PQFs) may miss high-quality details in low-quality frames. To remedy these shortcomings, in this paper we propose a novel end-to-end deep neural network called non-local ConvLSTM (NL-ConvLSTM in short) that exploits multiple consecutive frames. An approximate non-local strategy is introduced in NL-ConvLSTM to capture global motion patterns and trace the spatiotemporal dependency in a video sequence. This approximate strategy makes the non-local module work in a fast and low space-cost way. Our method uses the preceding and following frames of the target frame to generate a residual, from which a higher quality frame is reconstructed. Experiments on two datasets show that NL-ConvLSTM outperforms the existing methods.
更多查看译文
关键词
nonlocal ConvLSTM,video compression artifact reduction,high-quality videos,low-quality compressed videos,low-quality patches,high-quality patches,low-quality frames,end-to-end deep neural network,NL-ConvLSTM,approximate nonlocal strategy,video sequence,nonlocal module,peak-quality frames,global motion patterns,spatiotemporal dependency
AI 理解论文
溯源树
样例
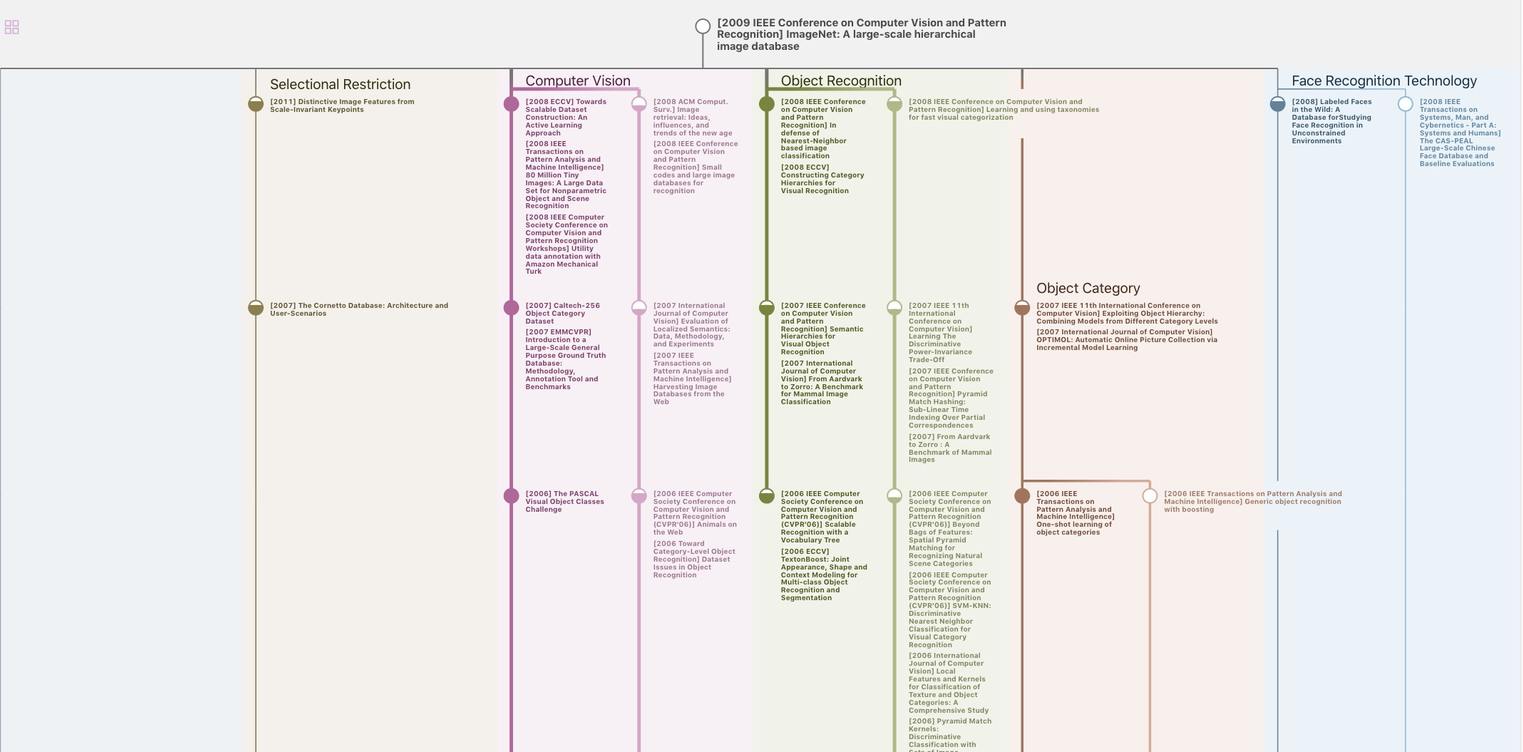
生成溯源树,研究论文发展脉络
Chat Paper
正在生成论文摘要