Understanding and Quantifying Adversarial Examples Existence in Linear Classification
2022 International Conference on Machine Learning and Cybernetics (ICMLC)(2022)
摘要
State-of-art deep neural networks (DNN) are vulnerable to attacks by adversarial examples: a carefully designed small perturbation to the input, that is imperceptible to human, can mislead DNN. To understand the root cause of adversarial examples, we quantify the probability of adversarial example existence for linear classifiers. Previous mathematical definition of adversarial examples only involves the overall perturbation amount, and we propose a more practical relevant definition of strong adversarial examples that separately limits the perturbation along the signal direction also. We show that linear classifiers can be made robust to strong adversarial examples attack in cases where no adversarial robust linear classifiers exist under the previous definition. The results suggest that designing general strong-adversarial-robust learning systems is feasible but only through incorporating human knowledge of the underlying classification problem.
更多查看译文
关键词
(Strong) Adversarial examples,(Strong) Adversarial Robustness,Gaussian mixture model
AI 理解论文
溯源树
样例
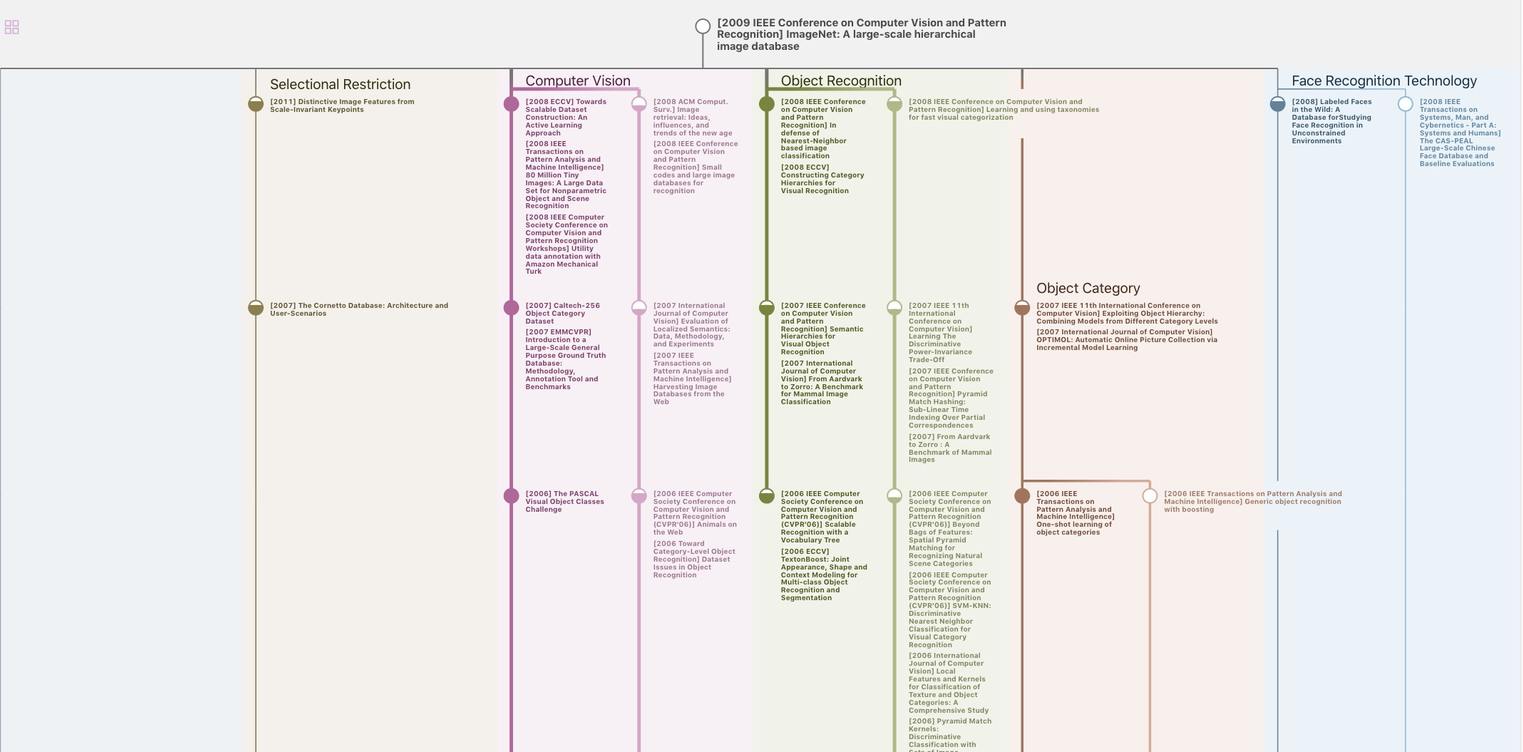
生成溯源树,研究论文发展脉络
Chat Paper
正在生成论文摘要