Attention for Inference Compilation
PROCEEDINGS OF THE 12TH INTERNATIONAL CONFERENCE ON SIMULATION AND MODELING METHODOLOGIES, TECHNOLOGIES AND APPLICATIONS (SIMULTECH)(2022)
摘要
We present a neural network architecture for automatic amortized inference in universal probabilistic programs which improves on the performance of current architectures. Our approach extends inference compilation (IC), a technique which uses deep neural networks to approximate a posterior distribution over latent variables in a probabilistic program. A challenge with existing IC network architectures is that they can fail to capture long-range dependencies between latent variables. To address this, we introduce an attention mechanism that attends to the most salient variables previously sampled in the execution of a probabilistic program. We demonstrate that the addition of attention allows the proposal distributions to better match the true posterior, enhancing inference about latent variables in simulators.
更多查看译文
关键词
Attention, Bayesian Inference, Probabilistic Programming, Inference Compilation
AI 理解论文
溯源树
样例
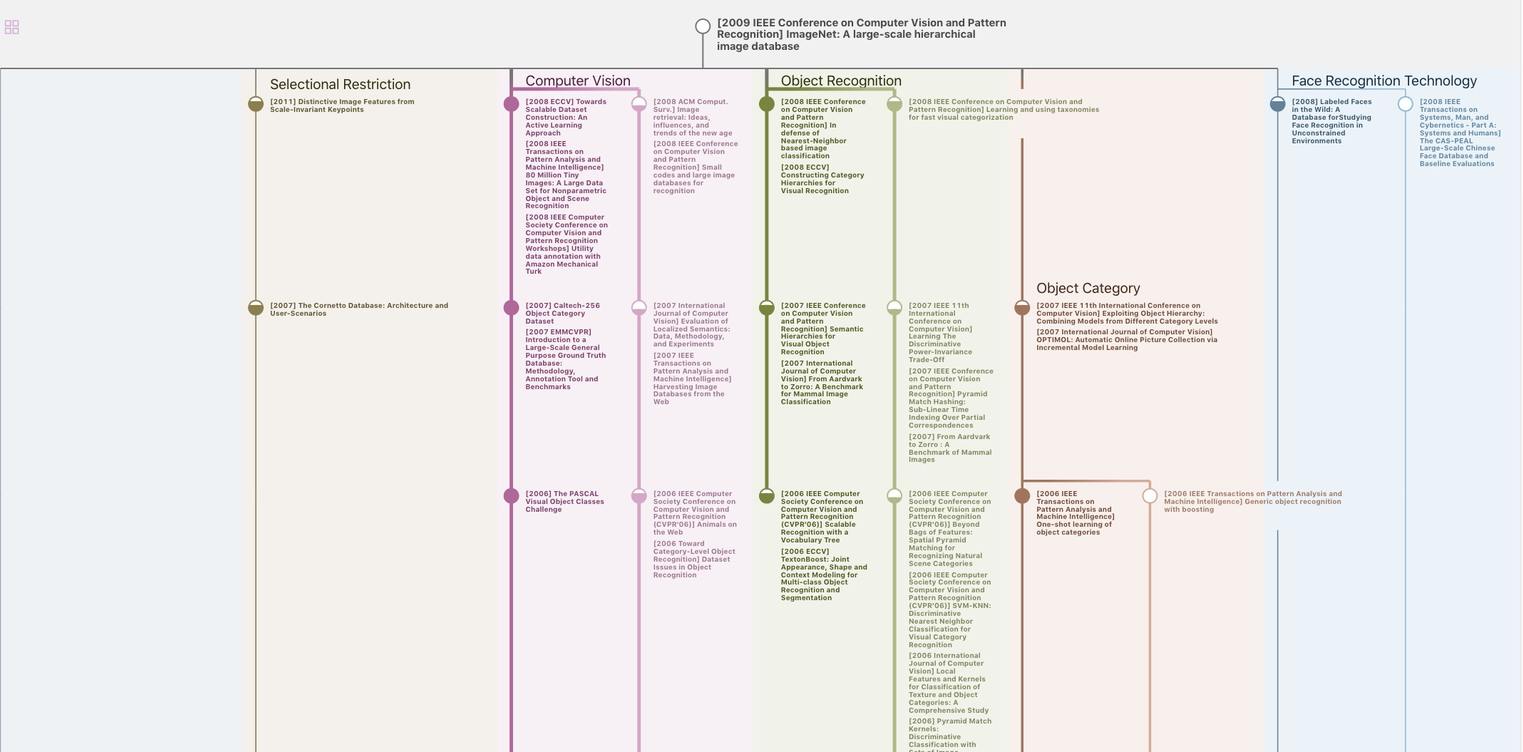
生成溯源树,研究论文发展脉络
Chat Paper
正在生成论文摘要