Learning Domain Invariant Representations For Child-Adult Classification From Speech
2020 IEEE INTERNATIONAL CONFERENCE ON ACOUSTICS, SPEECH, AND SIGNAL PROCESSING(2020)
摘要
Diagnostic procedures for ASD (autism spectrum disorder) involve semi-naturalistic interactions between the child and a clinician. Computational methods to analyze these sessions require an end-to-end speech and language processing pipeline that goes from raw audio to clinically-meaningful behavioral features. An important component of this pipeline is the ability to automatically detect who is speaking when i.e., perform child-adult speaker classification. This binary classification task is often confounded due to variability associated with the participants' speech and background conditions. Further, scarcity of training data often restricts direct application of conventional deep learning methods. In this work, we address two major sources of variability-age of the child and data source collection location-using domain adversarial learning which does not require labeled target domain data. We use two methods, generative adversarial training with inverted label loss and gradient reversal layer to learn speaker embeddings invariant to the above sources of variability, and analyze different conditions under which the proposed techniques improve over conventional learning methods. Using a large corpus of ADOS-2 (autism diagnostic observation schedule, 2nd edition) sessions, we demonstrate up to 13.45% and 6.44% relative improvements over conventional learning methods.
更多查看译文
关键词
Child speech, domain adversarial learning, gradient reversal, autism spectrum disorder
AI 理解论文
溯源树
样例
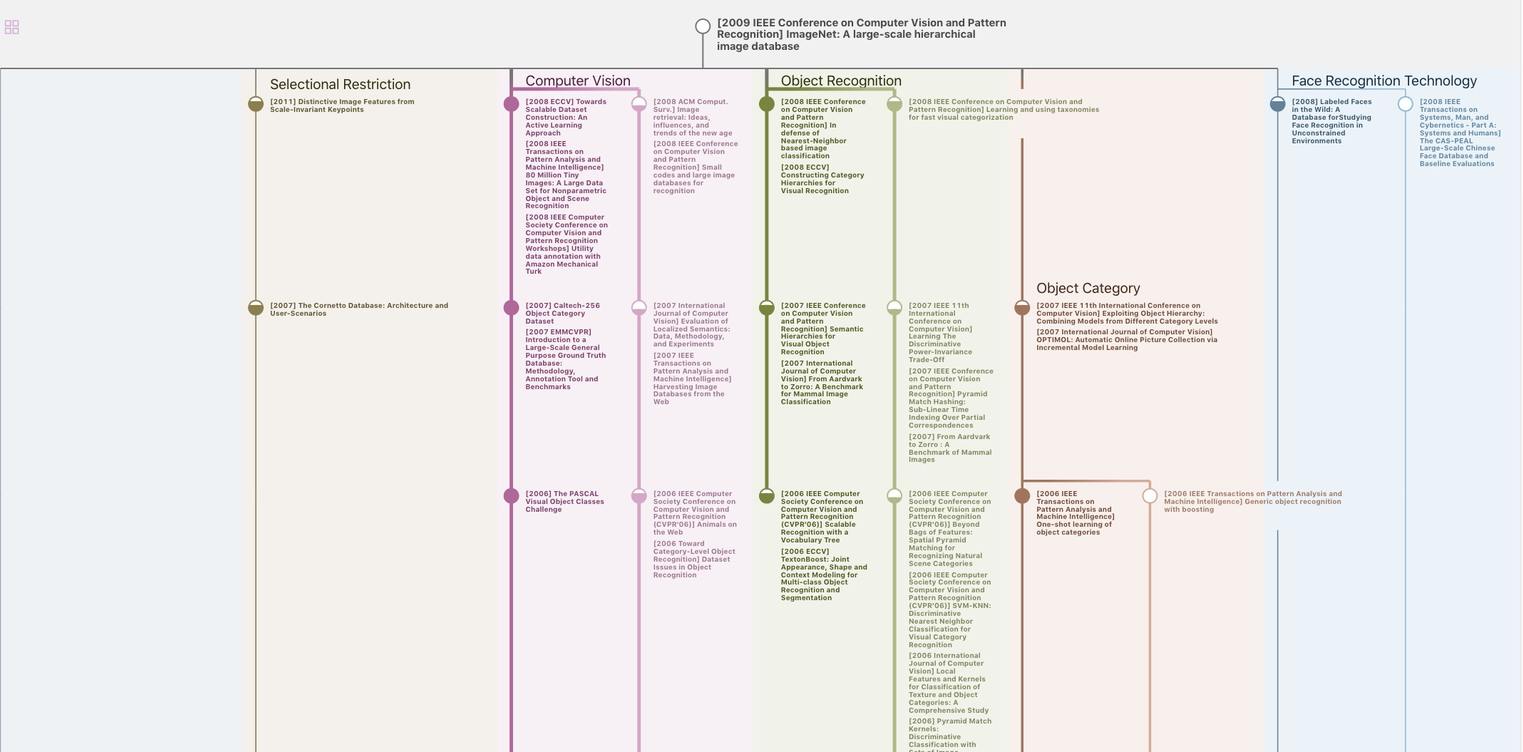
生成溯源树,研究论文发展脉络
Chat Paper
正在生成论文摘要