Energy-efficient and reliable in-memory classifier for machine-learning applications.
IET Computers & Digital Techniques(2019)
摘要
Large-scale machine-learning (ML) algorithms require extensive memory interactions. Managing or reducing data movement can significantly increase the speed and efficiency of many ML tasks. Towards this end, the authors devise an energy efficient in-memory computing (IMC) kernel for linear classification and design an initial prototype. The authors achieve a power savings of over 6.4 times than a conventional discrete system while improving reliability by 54.67%. The authors employ a split-data-aware technique to manage process, voltage, and temperature variations and to achieve fair trade-offs between energy efficiency, area requirements, and accuracy. The authors utilise a trimodal architecture with a hierarchical tree structure to further decrease power consumption. The authors also explore alternatives to the hierarchical tree structure with a significantly reduced number of linear regression blocks, while maintaining a competitive classification accuracy. Overall, the scheme provides a fast, energy efficient, and competitively accurate binary classification kernel.
更多查看译文
关键词
trees (mathematics),learning (artificial intelligence),buffer storage
AI 理解论文
溯源树
样例
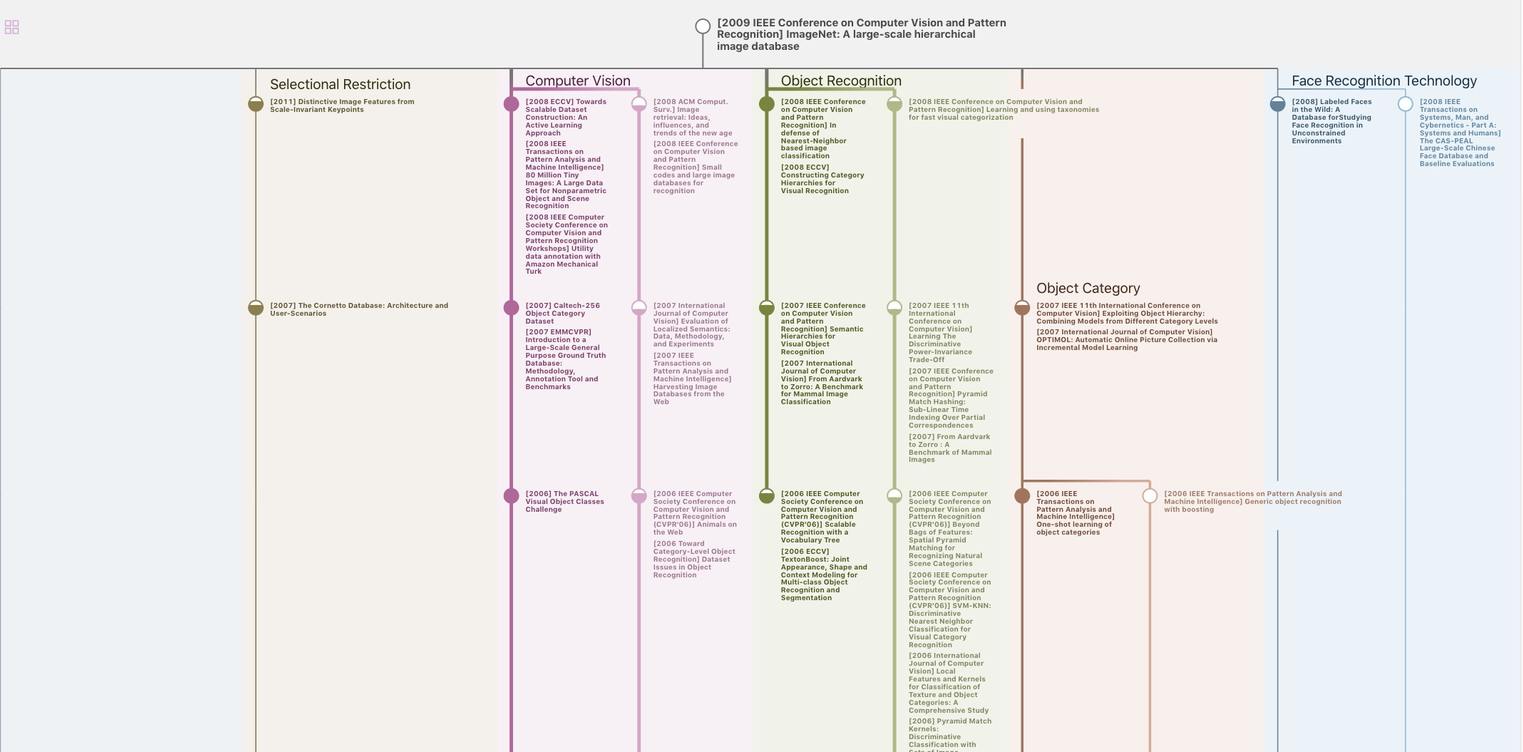
生成溯源树,研究论文发展脉络
Chat Paper
正在生成论文摘要