Improving the Generalization of Colorized Image Detection with Enhanced Training of CNN
2019 11th International Symposium on Image and Signal Processing and Analysis (ISPA)(2019)
摘要
Image colorization achieves more and more realistic results with the increasing power of recent deep learning techniques. It becomes more difficult to identify the synthetic colorized images by human eyes. In the literature, handcrafted-feature-based and convolutional neural network (CNN)-based forensic methods are proposed to distinguish between natural images (NIs) and colorized images (CIs). Although a recent CNN-based method achieves very good detection performance, an important issue (i.e., the blind detection problem) still remains and is not thoroughly studied. In this work, we focus on this challenging scenario of blind detection, i.e., no training sample is available from “unknown” colorization algorithm that we may encounter during the testing phase. This blind detection performance can be regarded as the generalization capability of a forensic detector. In this paper, we propose to first automatically construct negative samples through linear interpolation of paired natural and colorized images. Then, we progressively insert these negative samples into the original training dataset and continue to train the network. Experimental results demonstrate that our enhanced training can significantly improve the generalization performance of different CNN models.
更多查看译文
关键词
Image forensics,natural image,colorized image,convolutional neural network,generalization,negative samples
AI 理解论文
溯源树
样例
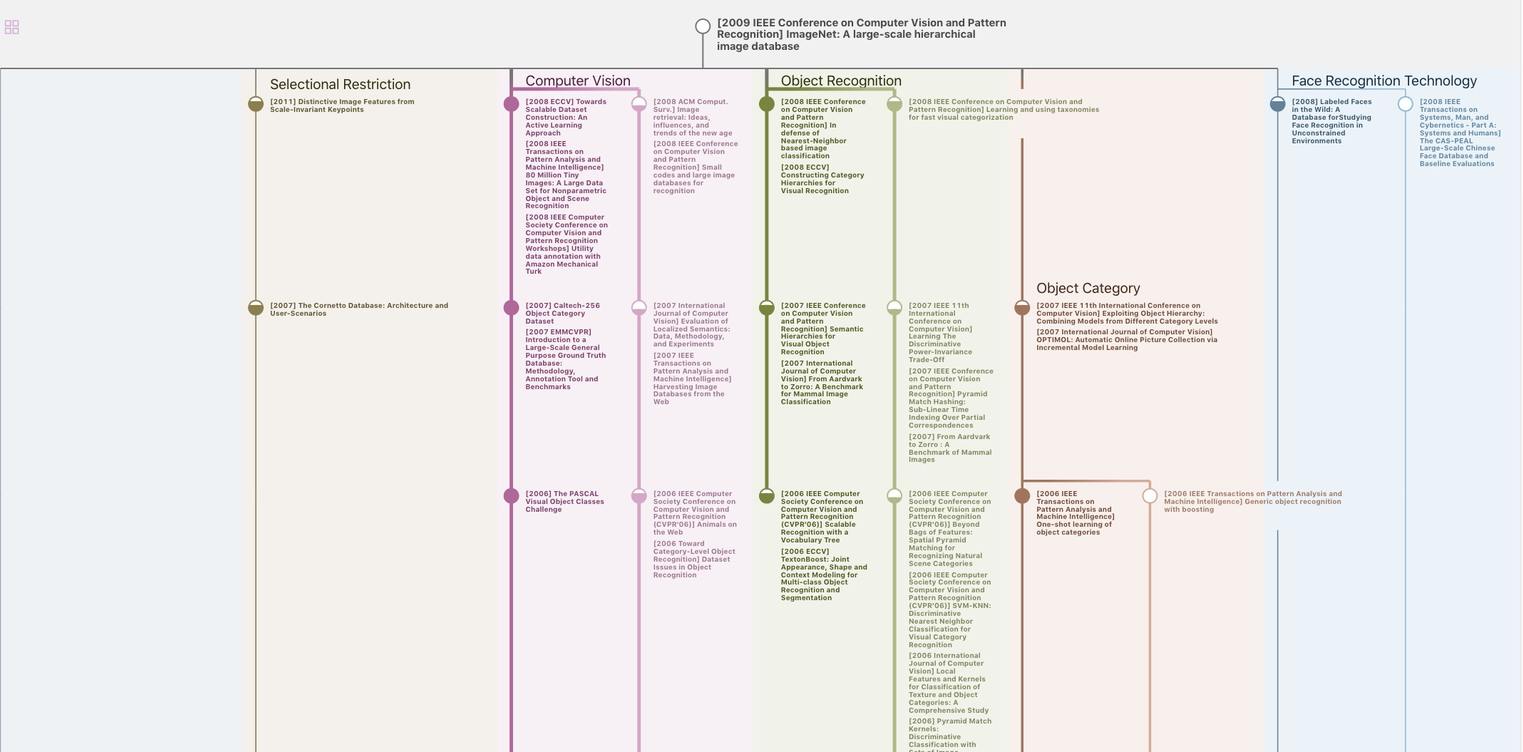
生成溯源树,研究论文发展脉络
Chat Paper
正在生成论文摘要