Performance Evaluation of Word and Sentence Embeddings for Finance Headlines Sentiment Analysis.
Communications in Computer and Information Science(2019)
摘要
Nowadays, tremendous number of financial online articles are published every day. Numerous natural language processing (NLP) algorithms and methodologies have arose, not only for correct, but also for fast financial sentiment extraction. Currently, word and sentence encoders are popular topic in NLP field, due to their ability to represent them as dense vectors in a continuous real numbers space, referred to as embeddings. These low dimensional embedding vectors are appropriate for deep neural networks (DNN) inputs, and their invention boosted the performance of multiple of NLP tasks. In this paper, we evaluate different word and sentence embeddings in combination with standard machine learning and deep-learning classifiers for financial texts sentiment extraction. Our evaluation shows the BiGRU+Attention architecture with word embedding as features, give the best score in overall evaluation.
更多查看译文
关键词
Sentiment analysis,Finance,Deep learning,Word embedding,Sentence embedding
AI 理解论文
溯源树
样例
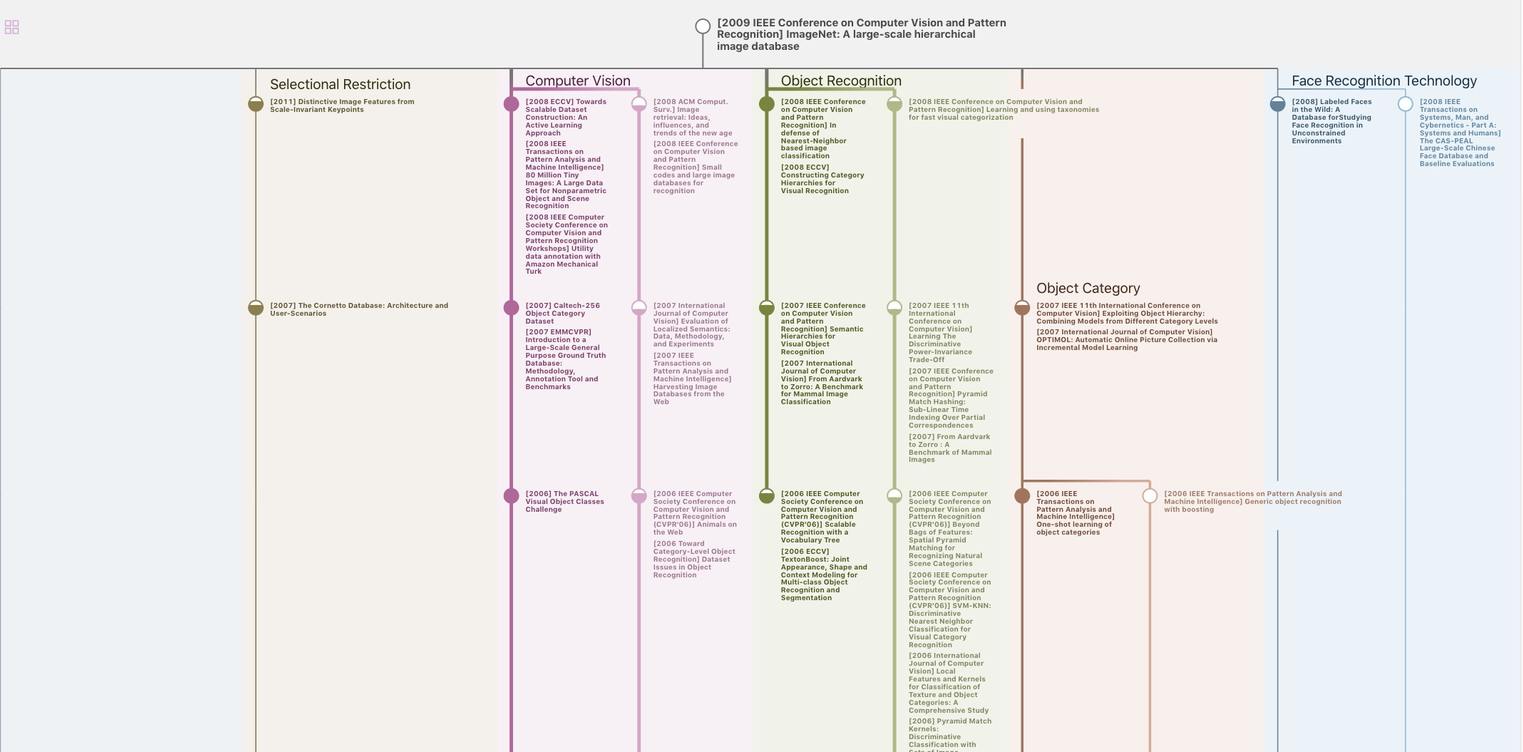
生成溯源树,研究论文发展脉络
Chat Paper
正在生成论文摘要