Fourier-Based Parametrization of Convolutional Neural Networks for Robust Time Series Forecasting.
DS(2019)
摘要
Classical statistical models for time series forecasting most often make a number of assumptions about the data at hand, therewith, requiring intensive manual preprocessing steps prior to modeling. As a consequence, it is very challenging to come up with a more generic forecasting framework. Extensive hyperparameter optimization and ensemble architectures are common strategies to tackle this problem, however, this comes at the cost of high computational complexity. Instead of optimizing hyperparameters by training multiple models, we propose a method to estimate optimal hyperparameters directly from the characteristics of the time series at hand. To that end, we use Convolutional Neural Networks (CNNs) for time series forecasting and determine a part of the network layout based on the time series' Fourier coefficients. Our approach significantly reduces the amount of required model configuration time and shows competitive performance on time series data across various domains. A comparison to popular, state of the art forecasting algorithms reveals further improvements in runtime and practicability.
更多查看译文
关键词
Time series forecasting, Neural networks, Fourier analysis
AI 理解论文
溯源树
样例
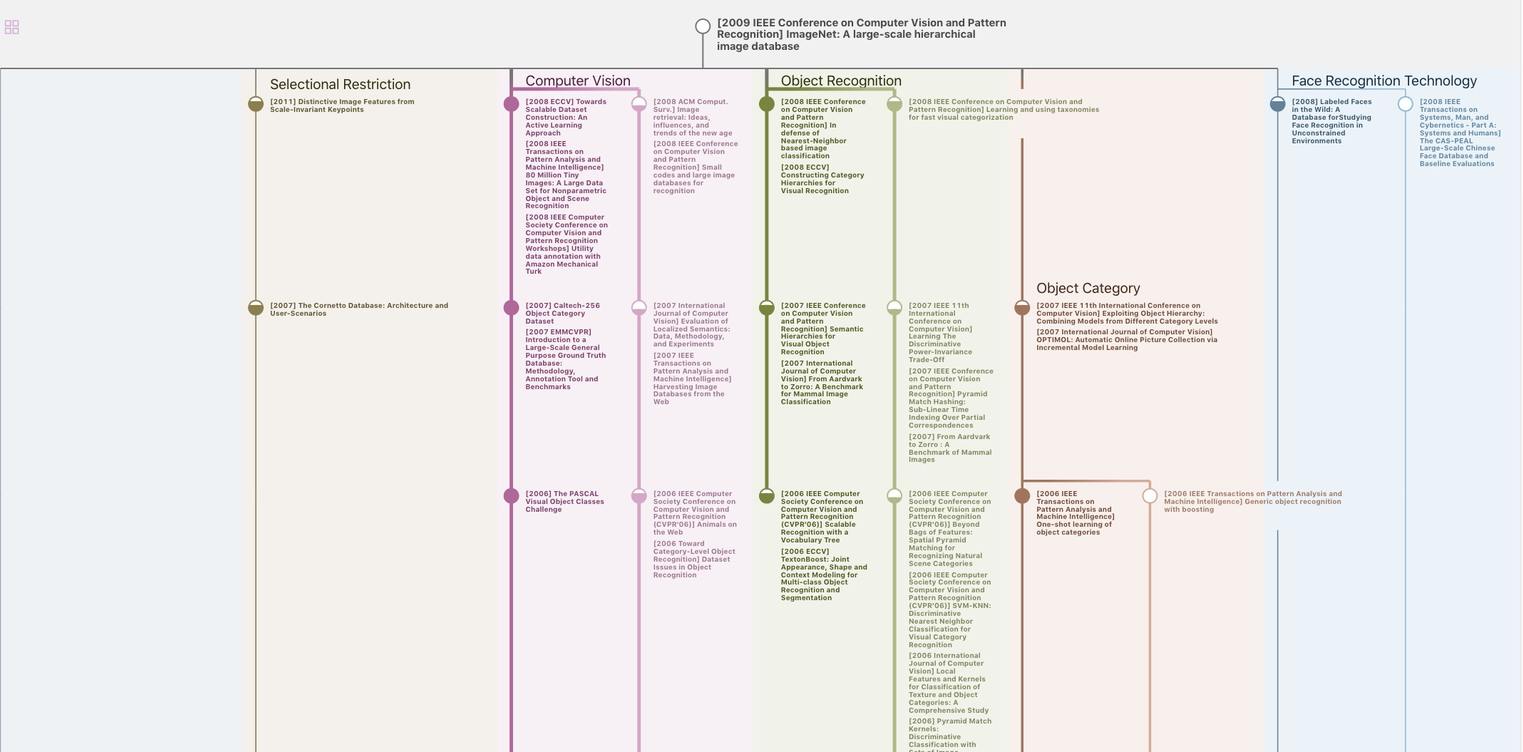
生成溯源树,研究论文发展脉络
Chat Paper
正在生成论文摘要