ADMM for multiaffine constrained optimization
OPTIMIZATION METHODS & SOFTWARE(2020)
摘要
We expand the scope of the alternating direction method of multipliers (ADMM). Specifically, we show that ADMM, when employed to solve problems with multiaffine constraints that satisfy certain verifiable assumptions, converges to the set of constrained stationary points if the penalty parameter in the augmented Lagrangian is sufficiently large. When the Kurdyka-Lojasiewicz (K-L) property holds, this is strengthened to convergence to a single constrained stationary point. Our analysis applies under assumptions that we have endeavoured to make as weak as possible. It applies to problems that involve nonconvex and/or nonsmooth objective terms, in addition to the multiaffine constraints that can involve multiple (three or more) blocks of variables. To illustrate the applicability of our results, we describe examples including nonnegative matrix factorization, sparse learning, risk parity portfolio selection, nonconvex formulations of convex problems and neural network training. In each case, our ADMM approach encounters only subproblems that have closed-form solutions.
更多查看译文
关键词
ADMM,nonconvex optimization,multilinear functions
AI 理解论文
溯源树
样例
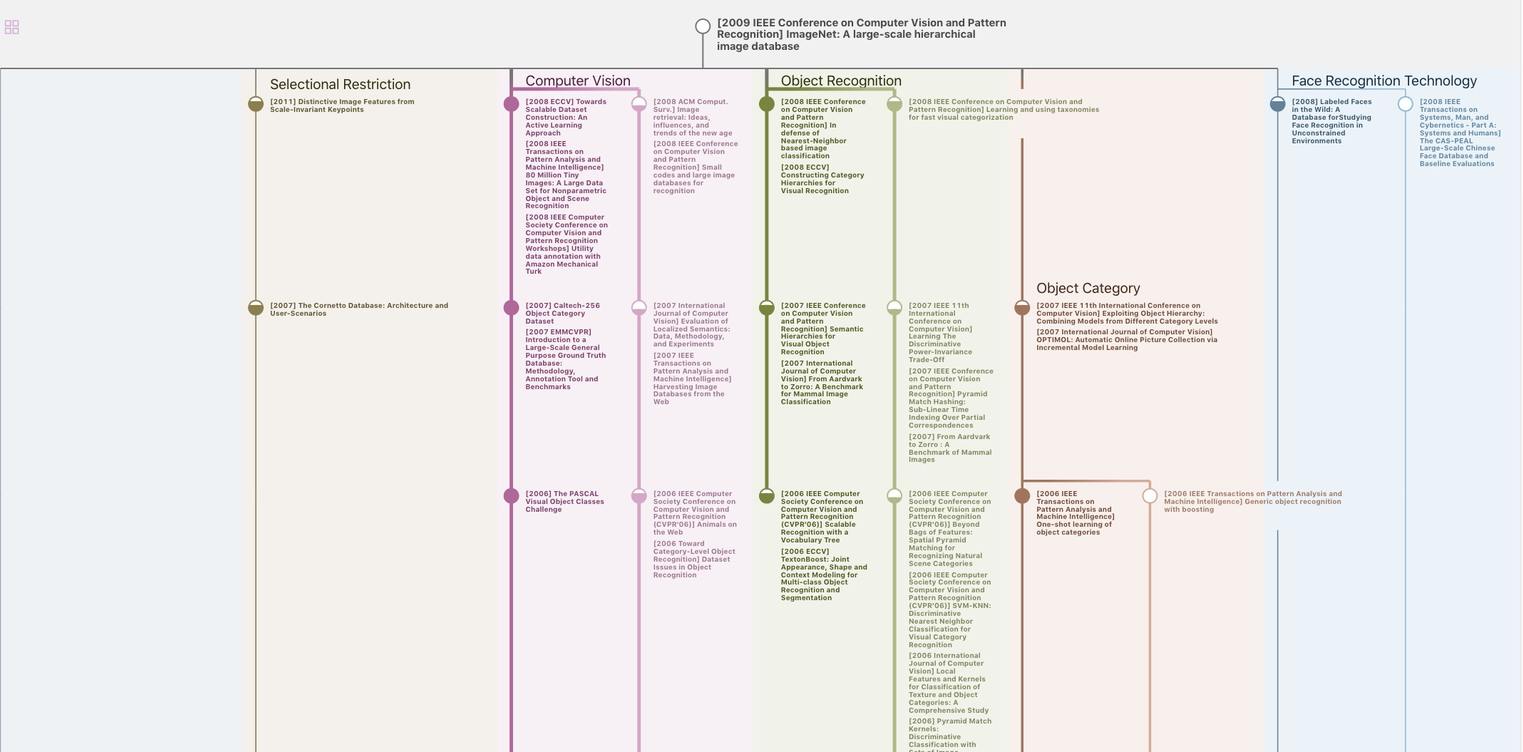
生成溯源树,研究论文发展脉络
Chat Paper
正在生成论文摘要