Spiking Neural Networks Trained With Backpropagation For Low Power Neuromorphic Implementation Of Voice Activity Detection
2020 IEEE INTERNATIONAL CONFERENCE ON ACOUSTICS, SPEECH, AND SIGNAL PROCESSING(2020)
摘要
Recent advances in Voice Activity Detection (VAD) are driven by artificial and Recurrent Neural Networks (RNNs), however, using a VAD system in battery-operated devices requires further power efficiency. This can be achieved by neuromorphic hardware, which enables Spiking Neural Networks (SNNs) to perform inference at very low energy consumption. Spiking networks are characterized by their ability to process information efficiently, in a sparse cascade of binary events in time called spikes. However, a big performance gap separates artificial from spiking networks, mostly due to a lack of powerful SNN training algorithms. To overcome this problem we exploit an SNN model that can be recast into a recurrent network and trained with known deep learning techniques. We describe a training procedure that achieves low spiking activity and apply pruning algorithms to remove up to 85% of the network connections with no performance loss. The model competes with state-of-the-art performance at a fraction of the power consumption comparing to other methods.
更多查看译文
关键词
Spiking Neural Networks, Voice Activity Detection, Power Efficiency, Backpropagation, Neuromorphic Microchips
AI 理解论文
溯源树
样例
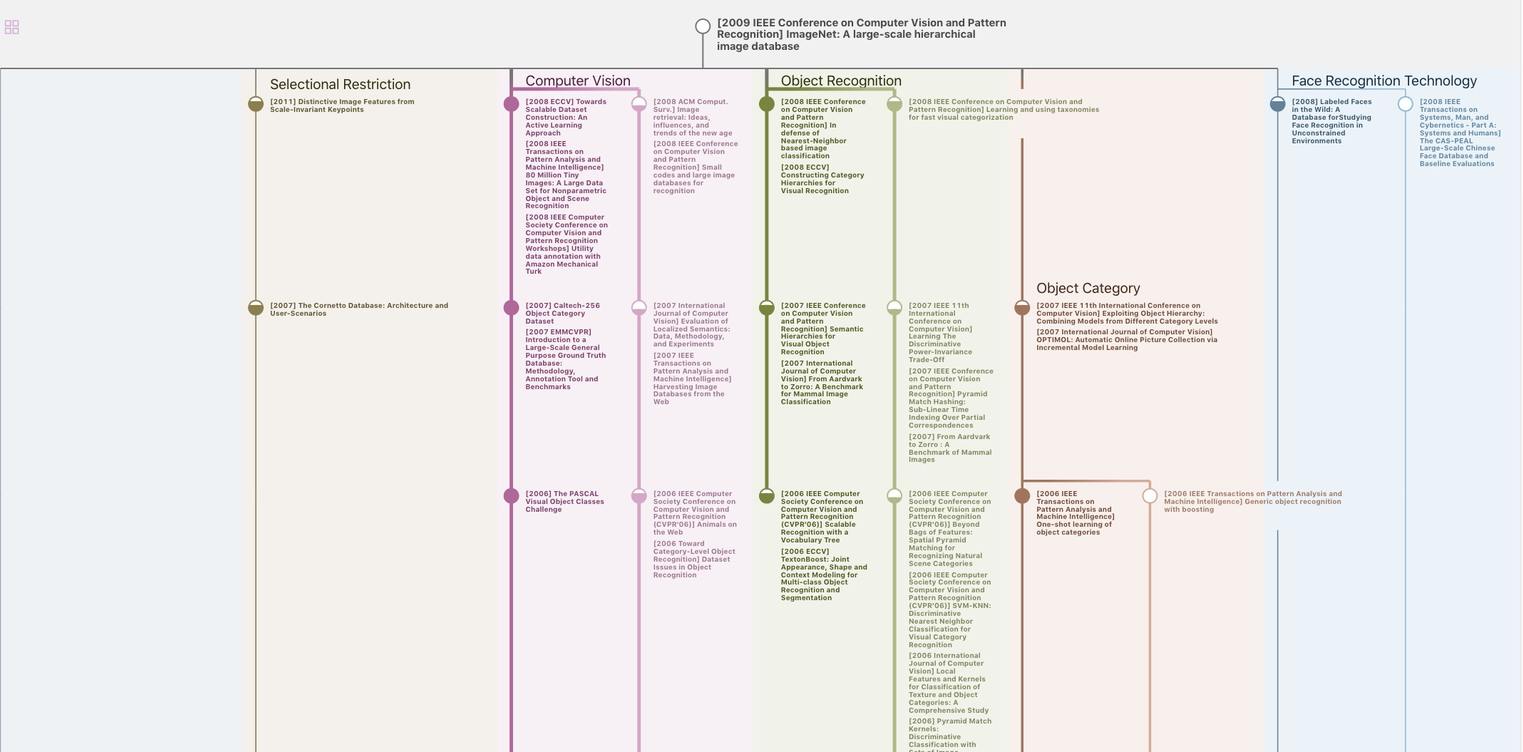
生成溯源树,研究论文发展脉络
Chat Paper
正在生成论文摘要