Sequence-to-sequence Singing Synthesis Using the Feed-forward Transformer
2020 IEEE INTERNATIONAL CONFERENCE ON ACOUSTICS, SPEECH, AND SIGNAL PROCESSING(2019)
摘要
We propose a sequence-to-sequence singing synthesizer, which avoids the need for training data with pre-aligned phonetic and acoustic features. Rather than the more common approach of a content-based attention mechanism combined with an autoregressive decoder, we use a different mechanism suitable for feed-forward synthesis. Given that phonetic timings in singing are highly constrained by the musical score, we derive an approximate initial alignment with the help of a simple duration model. Then, using a decoder based on a feed-forward variant of the Transformer model, a series of self-attention and convolutional layers refines the result of the initial alignment to reach the target acoustic features. Advantages of this approach include faster inference and avoiding the exposure bias issues that affect autoregressive models trained by teacher forcing. We evaluate the effectiveness of this model compared to an autoregressive baseline, the importance of self-attention, and the importance of the accuracy of the duration model.
更多查看译文
关键词
Singing synthesis, sequence-to-sequence, self-attention, feed-forward, transformer
AI 理解论文
溯源树
样例
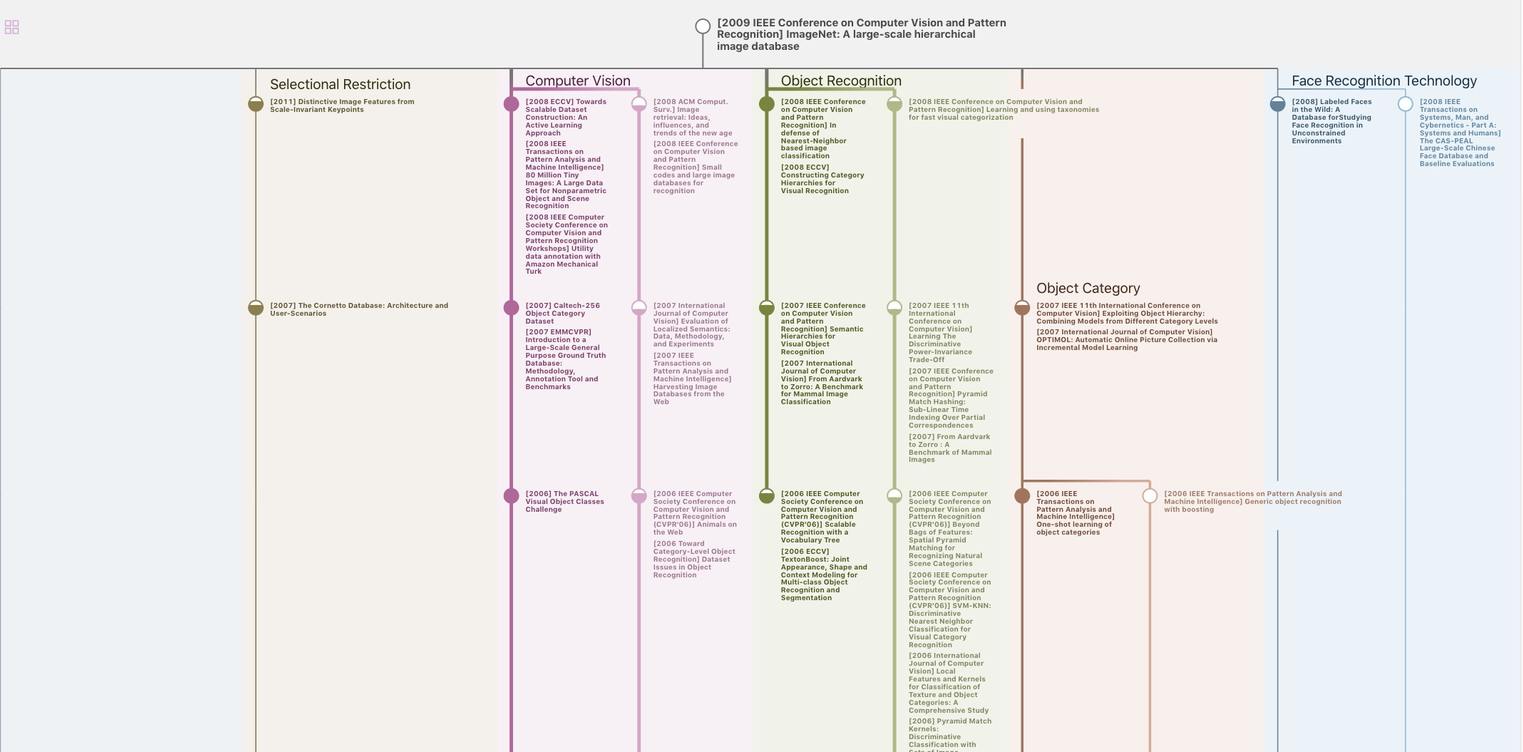
生成溯源树,研究论文发展脉络
Chat Paper
正在生成论文摘要