Sparse Temporal Causal Convolution for Efficient Action Modeling
Proceedings of the 27th ACM International Conference on Multimedia(2019)
摘要
Recently, spatio-temporal convolutional networks have achieved prominent performance in action classification. However, debates on the importance of temporal information lead to the rethinking of these architectures. In this work, we propose to employ sparse temporal convolutional operations in networks for efficient action modeling. We demonstrate that the explicit temporal feature interactions can be largely reduced without any degradation. And towards better scalability, we use causal convolutions for temporal feature learning. Under causality constraints, we replenish the model with auxiliary self-supervised tasks, namely video prediction and frame order discrimination. Besides, a gradient based multi-task learning algorithm is introduced for guaranteeing the dominance of action recognition task. The proposed model matches or outperforms the state-of-the-art methods on Kinetics, Something-Something V2, UCF101 and HMDB51 datasets.
更多查看译文
关键词
action recognition, causal modeling, multi-task learning
AI 理解论文
溯源树
样例
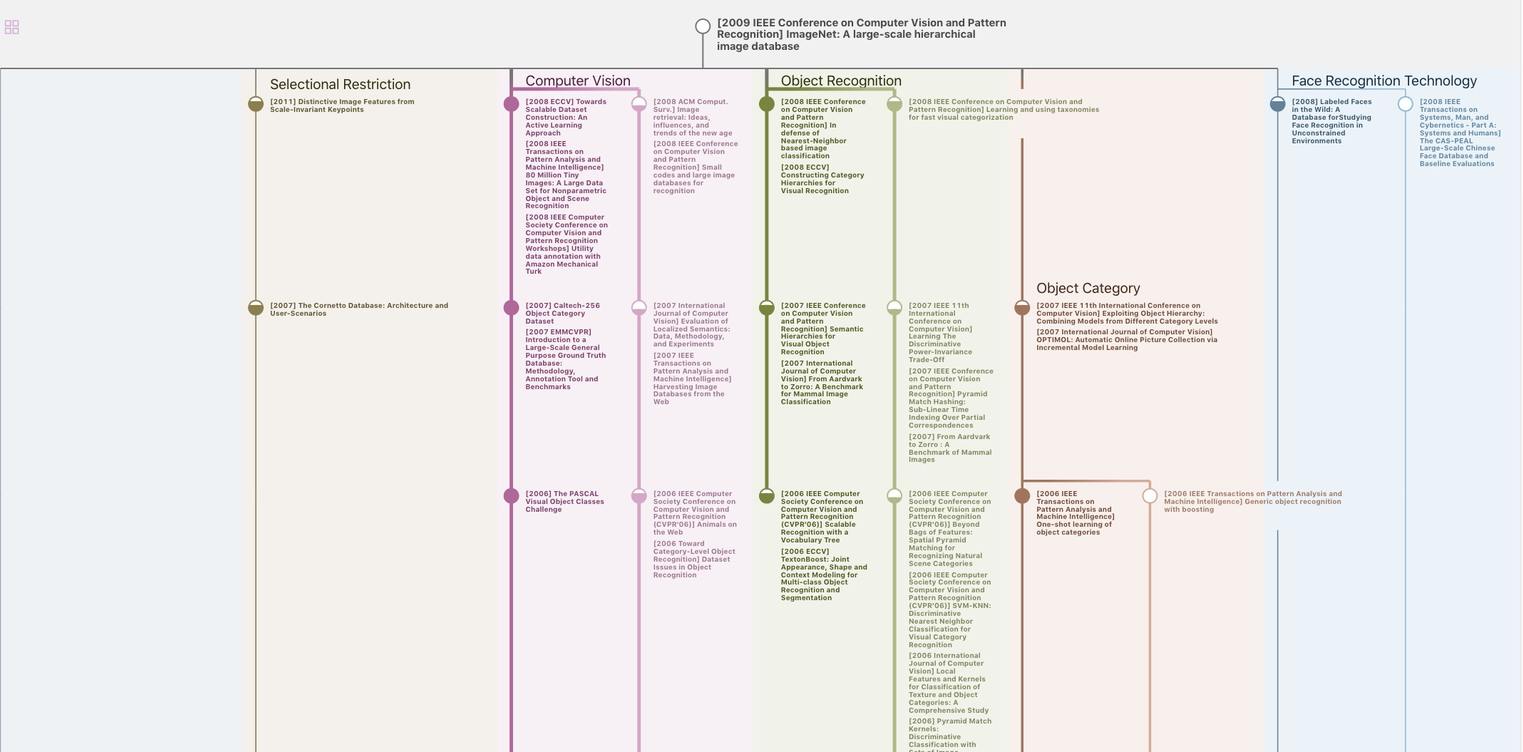
生成溯源树,研究论文发展脉络
Chat Paper
正在生成论文摘要