Online Bagging for Anytime Transfer Learning
2019 IEEE SYMPOSIUM SERIES ON COMPUTATIONAL INTELLIGENCE (IEEE SSCI 2019)(2019)
摘要
Transfer learning techniques have been widely used in the reality that it is difficult to obtain sufficient labeled data in the target domain, but a large amount of auxiliary data can be obtained in the relevant source domain. But most of the existing methods are based on offline data. In practical applications, it is often necessary to face online learning problems in which the data samples are achieved sequentially. In this paper, We are committed to applying the ensemble approach to solving the problem of online transfer learning so that it can be used in anytime setting. More specifically, we propose a novel online transfer learning framework, which applies the idea of online bagging methods to anytime transfer learning problems, and constructs strong classifiers through online iterations of the usefulness of multiple weak classifiers. Further, our algorithm also provides two extension schemes to reduce the impact of negative transfer. Experiments on three real data sets show that the effectiveness of our proposed algorithms.
更多查看译文
关键词
online transfer learning, online bagging, ensemble learning, negative transfer
AI 理解论文
溯源树
样例
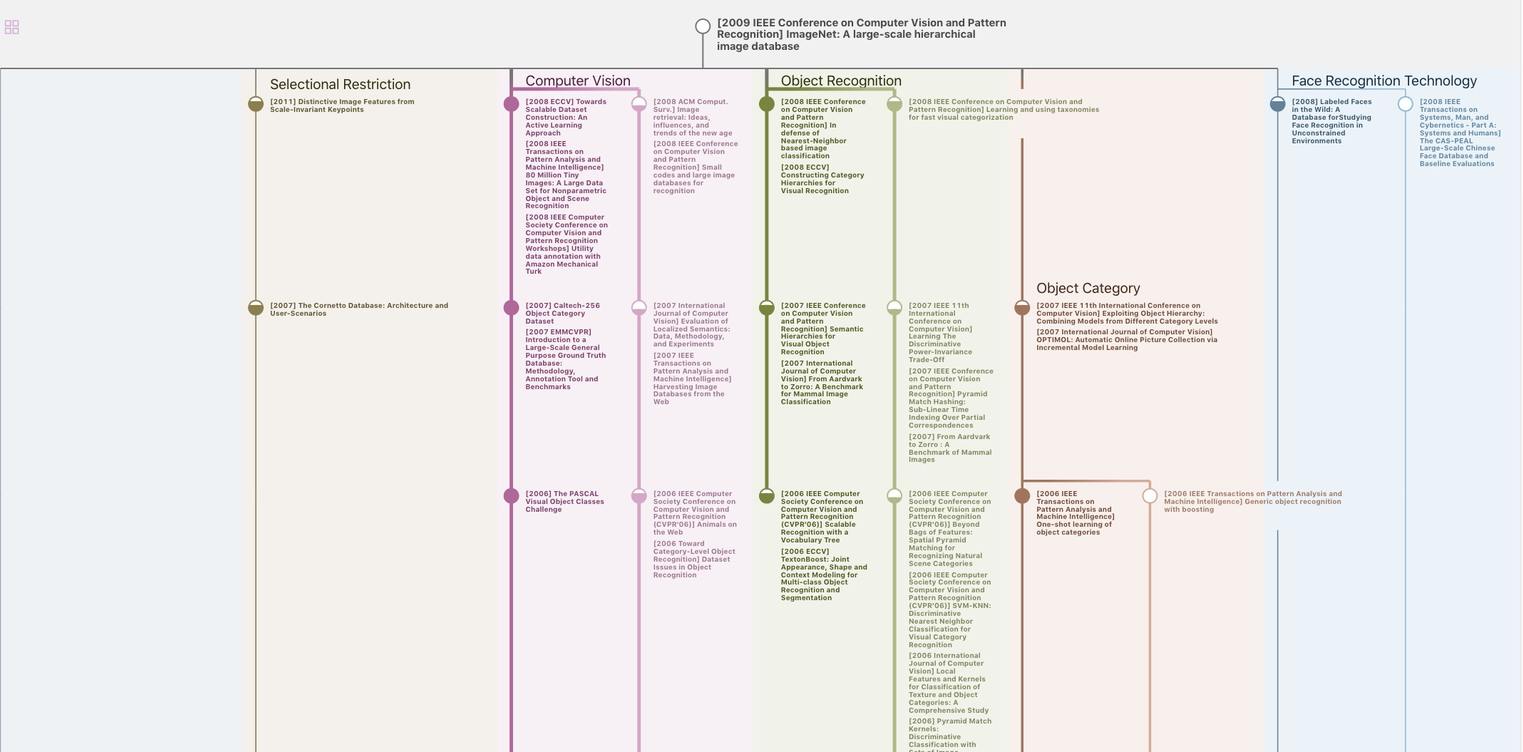
生成溯源树,研究论文发展脉络
Chat Paper
正在生成论文摘要