Component Attention Guided Face Super-Resolution Network: CAGFace
2020 IEEE Winter Conference on Applications of Computer Vision (WACV)(2020)
摘要
To make the best use of the underlying structure of faces, the collective information through face datasets and the intermediate estimates during the upsampling process, here we introduce a fully convolutional multi-stage neural network for 4× super-resolution for face images. We implicitly impose facial component-wise attention maps using a segmentation network to allow our network to focus on face-inherent patterns. Each stage of our network is composed of a stem layer, a residual backbone, and spatial upsampling layers. We recurrently apply stages to reconstruct an intermediate image, and then reuse its space-to-depth converted versions to bootstrap and enhance image quality progressively. Our experiments show that our face super-resolution method achieves quantitatively superior and perceptually pleasing results in comparison to state of the art.
更多查看译文
关键词
face datasets,facial component-wise attention maps,segmentation network,face-inherent patterns,spatial upsampling layers,face super-resolution method,convolutional multistage neural network
AI 理解论文
溯源树
样例
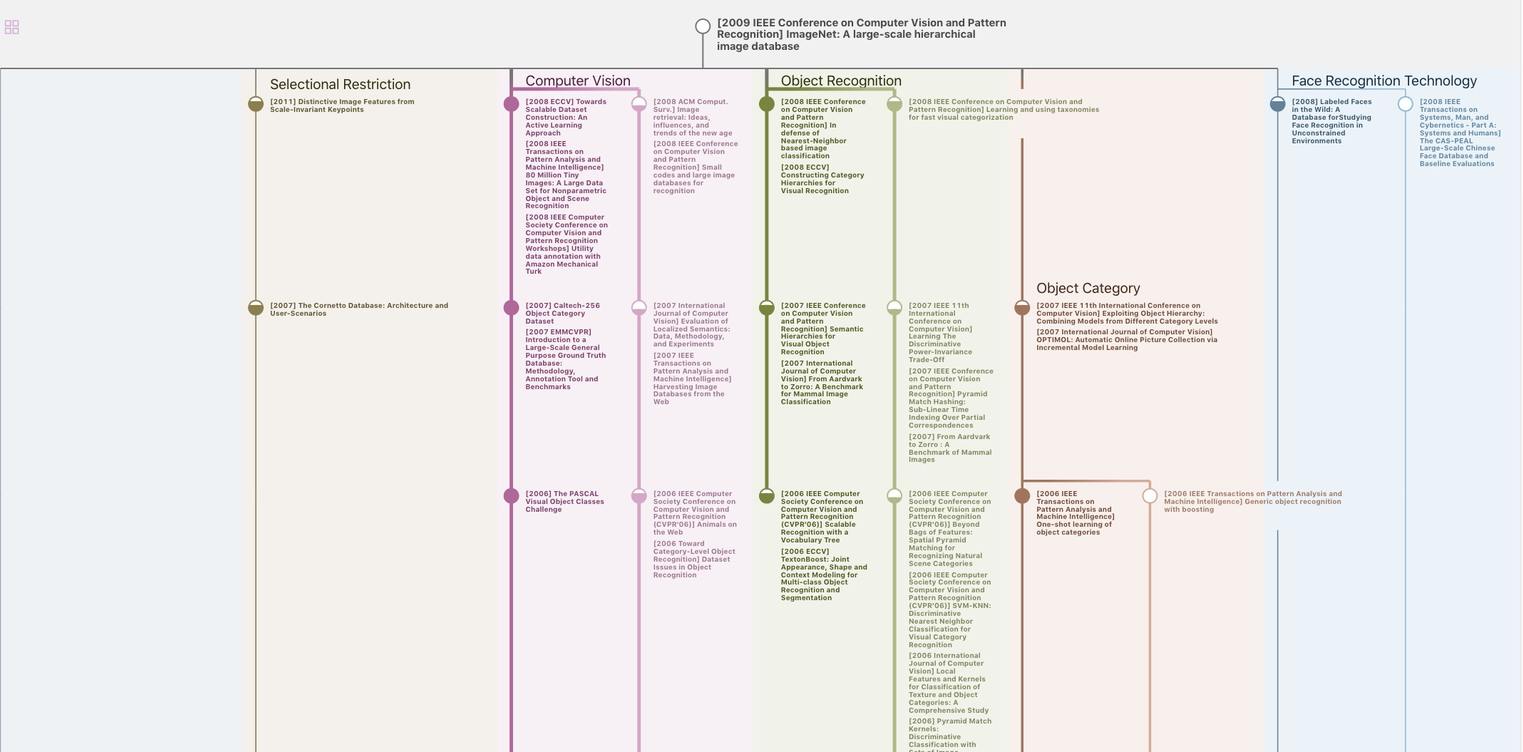
生成溯源树,研究论文发展脉络
Chat Paper
正在生成论文摘要