Evolutionary Dynamic Multi-objective Optimization Via Regression Transfer Learning
2019 IEEE SYMPOSIUM SERIES ON COMPUTATIONAL INTELLIGENCE (IEEE SSCI 2019)(2019)
摘要
Dynamic multi-objective optimization problems (DMOPs) remain a challenge to be settled, because of conflicting objective functions change over time. In recent years, transfer learning has been proven to be a kind of effective approach in solving DMOPs. In this paper, a novel transfer learning based dynamic multi-objective optimization algorithm (DMOA) is proposed called regression transfer learning prediction based DMOA (RTLP-DMOA). The algorithm aims to generate an excellent initial population to accelerate the evolutionary process and improve the evolutionary performance in solving DMOPs. When an environmental change is detected, a regression transfer learning prediction model is constructed by reusing the historical population, which can predict objective values. Then, with the assistance of this prediction model, some high-quality solutions with better predicted objective values are selected as the initial population, which can improve the performance of the evolutionary process. We compare the proposed algorithm with three state-of-the-art algorithms on benchmark functions. Experimental results indicate that the proposed algorithm can significantly enhance the performance of static multi-objective optimization algorithms and is competitive in convergence and diversity.
更多查看译文
关键词
evolutionary algorithm, dynamic multi-objective optimization, transfer learning, regression prediction
AI 理解论文
溯源树
样例
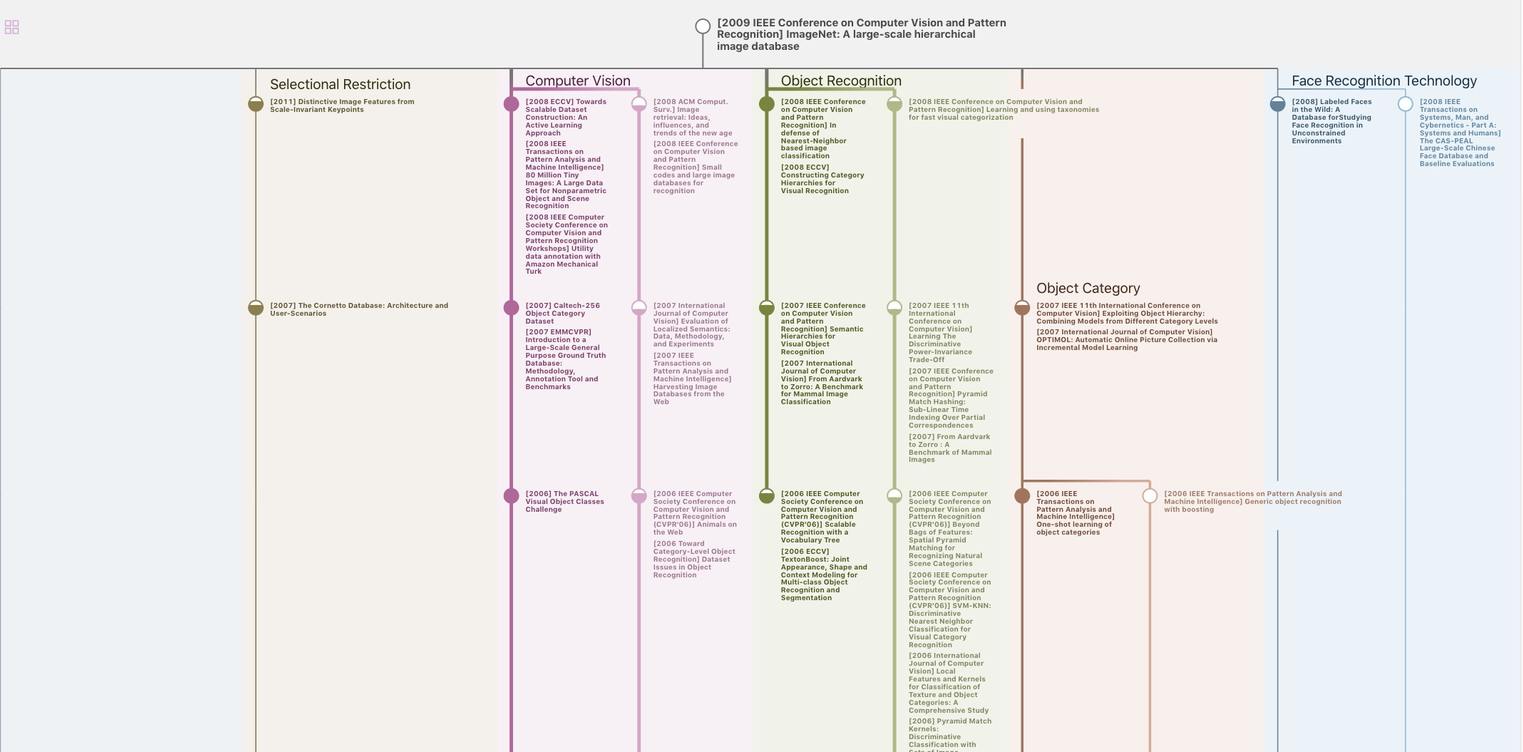
生成溯源树,研究论文发展脉络
Chat Paper
正在生成论文摘要