Toward 3D object reconstruction from stereo images
Neurocomputing(2021)
摘要
Inferring the complete 3D shape of an object from an RGB image has shown impressive results, however, existing methods rely primarily on recognizing the most similar 3D model from the training set to solve the problem. These methods suffer from poor generalization and may lead to low-quality reconstructions for unseen objects. Nowadays, stereo cameras are pervasive in emerging devices such as dual-lens smartphones and robots, which enables the use of the two-view nature of stereo images to explore the 3D structure and thus improve the reconstruction performance. In this paper, we propose a new deep learning framework for reconstructing the 3D shape of an object from a pair of stereo images, which reasons about the 3D structure of the object by taking bidirectional disparities and feature correspondences between the two views into account. Besides, we present a large-scale synthetic benchmarking dataset, namely StereoShapeNet, containing 1,052,976 pairs of stereo images rendered from ShapeNet along with the corresponding bidirectional depth and disparity maps. Experimental results on the StereoShapeNet benchmark demonstrate that the proposed framework outperforms the state-of-the-art methods. The project page is available at https://haozhexie.com/project/stereo-3d-reconstruction.
更多查看译文
关键词
3D object reconstruction,Stereo vision,Voxel,Point cloud,Neural network
AI 理解论文
溯源树
样例
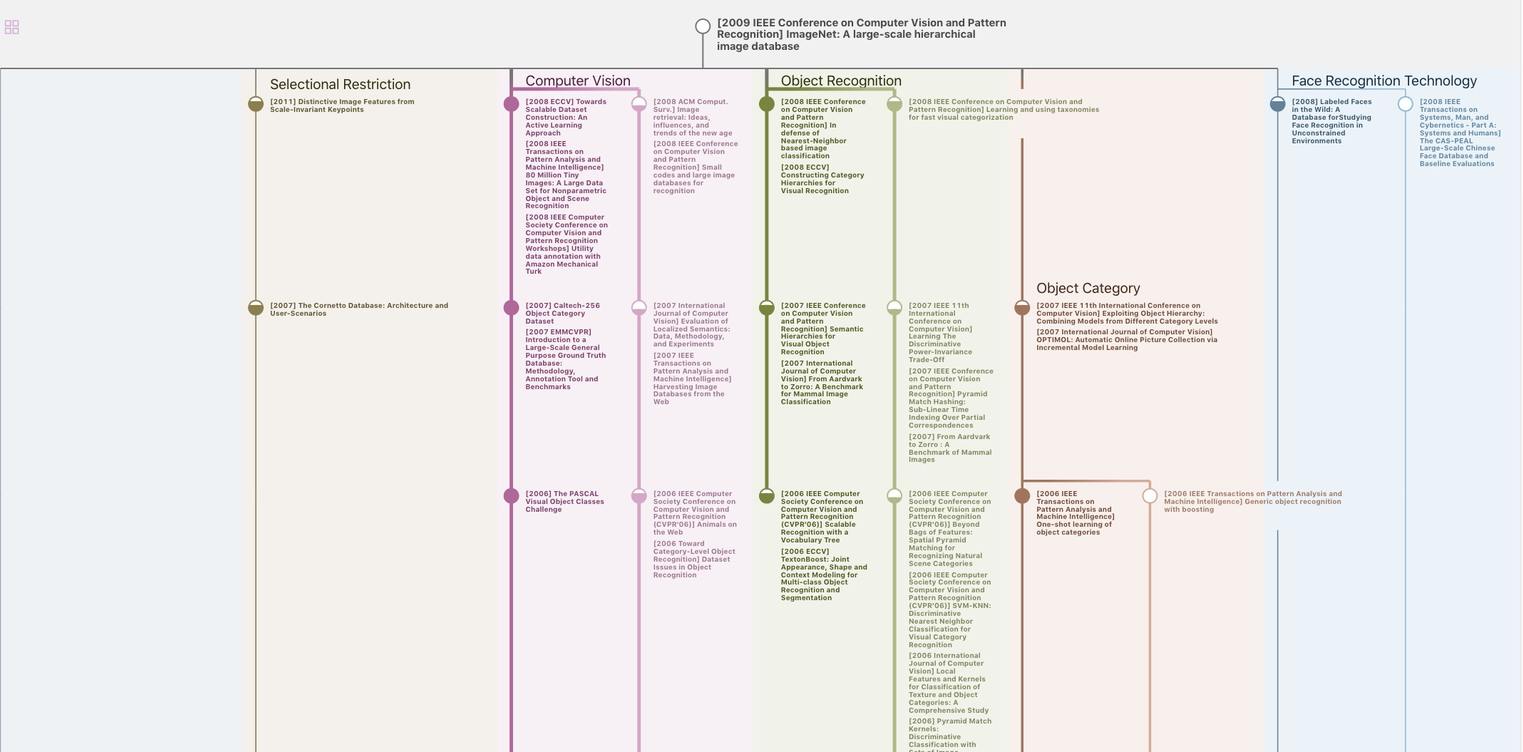
生成溯源树,研究论文发展脉络
Chat Paper
正在生成论文摘要