Robust Spoken Language Understanding with Acoustic and Domain Knowledge.
ICMI(2019)
摘要
Spoken language understanding (SLU) converts user utterances into structured semantic forms. There are still two main issues for SLU: robustness to ASR-errors and the data sparsity of new and extended domains. In this paper, we propose a robust SLU system by leveraging both acoustic and domain knowledge. We extract audio features by training ASR models on a large number of utterances without semantic annotations. For exploiting domain knowledge, we design lexicon features from the domain ontology and propose an error elimination algorithm to help predicted values recovered from ASR-errors. The results of CATSLU challenge show that our systems can outperform all of the other teams across four domains.
更多查看译文
关键词
Spoken Language Understanding, Robustness
AI 理解论文
溯源树
样例
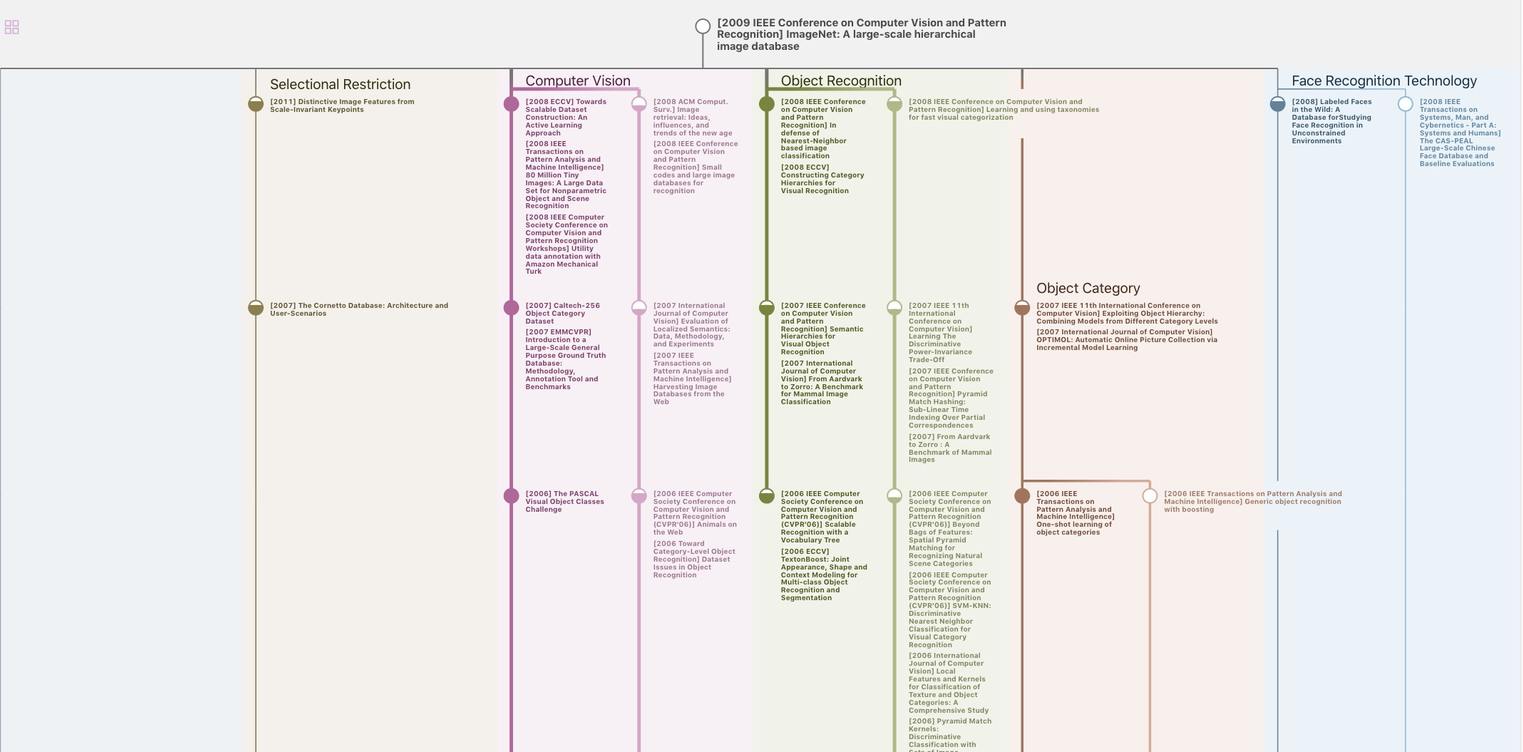
生成溯源树,研究论文发展脉络
Chat Paper
正在生成论文摘要