Machine Learning Based Web-Traffic Analysis For Detection Of Fraudulent Resource Consumption Attack In Cloud
2019 IEEE/WIC/ACM INTERNATIONAL CONFERENCE ON WEB INTELLIGENCE (WI 2019)(2019)
摘要
Attackers can orchestrate a fraudulent resource consumption (FRC) attack by wittingly consuming metered resources of the cloud servers for a long duration of time. The skillful over-consumption of the resources results in significant financial burden to the client. These attacks differ in intent but not in content, hence they are hard to detect. In this paper, we propose a novel scheme for the detection of the FRC attack on a cloud based web-server. We first divide the web-pages into a number of quantiles based on their popularity index. Next, we compute the number of requests per hour for each of these quantiles. DiscreteWavelet Transform is then applied to these quantiles to remove any high-frequency anomaly and smoothen the time series data. The n-tuple data from these quantiles along with their label (attack or normal) is used to train an Artificial Neural Network model. Our trained model for low percent of FRC attack (5%) obtained an accuracy of 98.51% with a precision of 0.983 and recall of 0.987 in detecting the FRC attack.
更多查看译文
关键词
Fraudulent Resource Consumption Attack, Cloud Infrastructure, Denial of Service Attack, Artificial Neural Network, Discrete Wavelet Transform
AI 理解论文
溯源树
样例
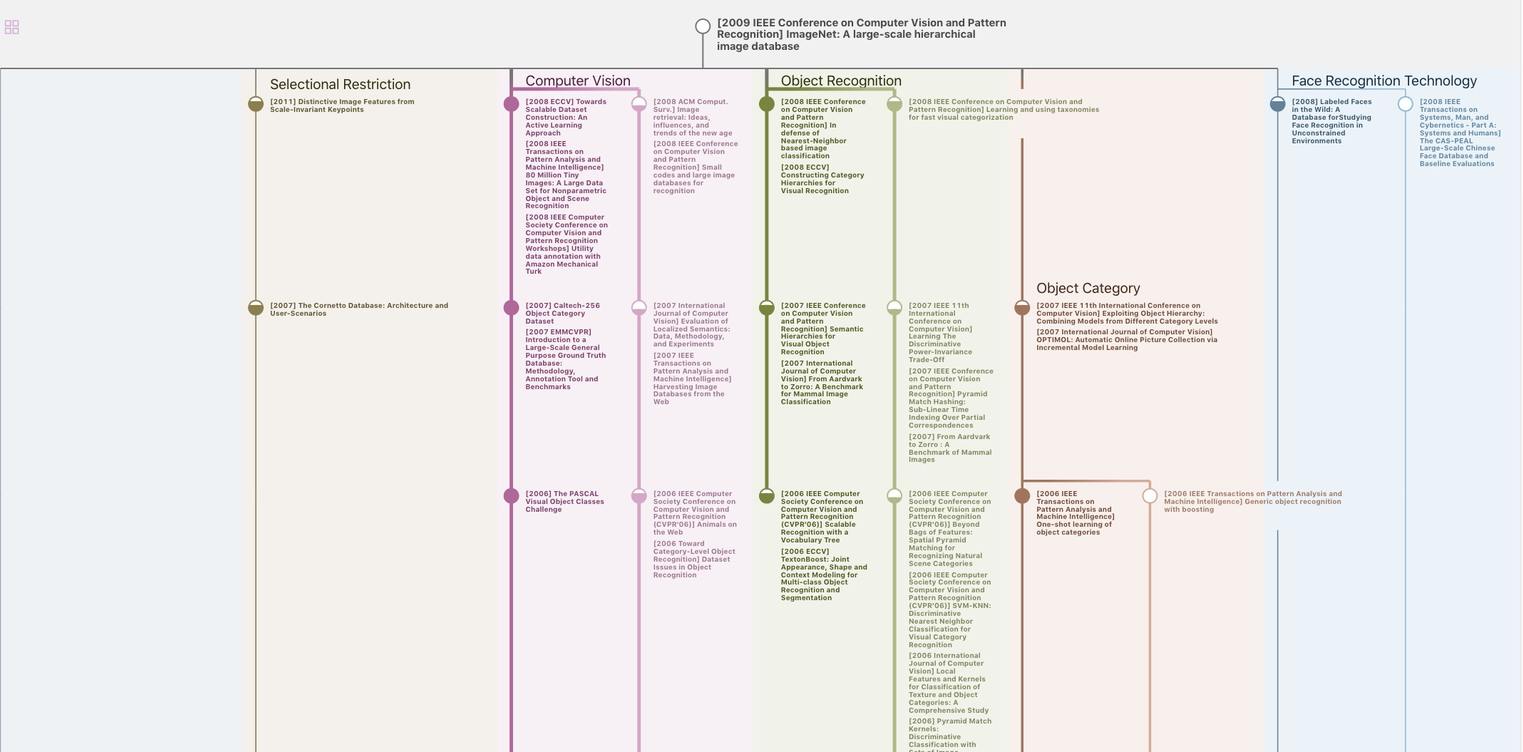
生成溯源树,研究论文发展脉络
Chat Paper
正在生成论文摘要