Towards Instantaneous Recovery from Autonomous System Failures via Predictive Crowdsourcing
The Adjunct Publication of the 32nd Annual ACM Symposium on User Interface Software and Technology(2019)
摘要
Autonomous systems (e.g., long-distance driverless trucks) aim to reduce the need for people to complete tedious tasks. In many domains, automation is challenging because systems may fail to recognize or comprehend all relevant aspects of its current state. When an unknown or uncertain state is encountered in a mission-critical setting, recovery often requires human intervention or hand-off. However, human intervention is associated with decision (and communication, if remote) delays that prevent recovery in low-latency settings. Instantaneous crowdsourcing approaches that leverage predictive techniques reduce this latency by preparing human responses for possible near future states before they occur. Unfortunately, the number of possible future states can be vast and considering all of them is intractable in all but the simplest of settings. Instead, to reduce the number of states that must later be explored, we propose the approach that uses the crowd to first predict the most relevant or likely future states. We examine the latency and accuracy of crowd workers in a simple future state prediction task, and find that more than half of crowd workers were able to provide accurate answers within one second. Our results show that crowd predictions can filter out critical future states in tasks where decisions are required in less than three seconds.
更多查看译文
关键词
human computation, prediction, real-time crowdsourcing
AI 理解论文
溯源树
样例
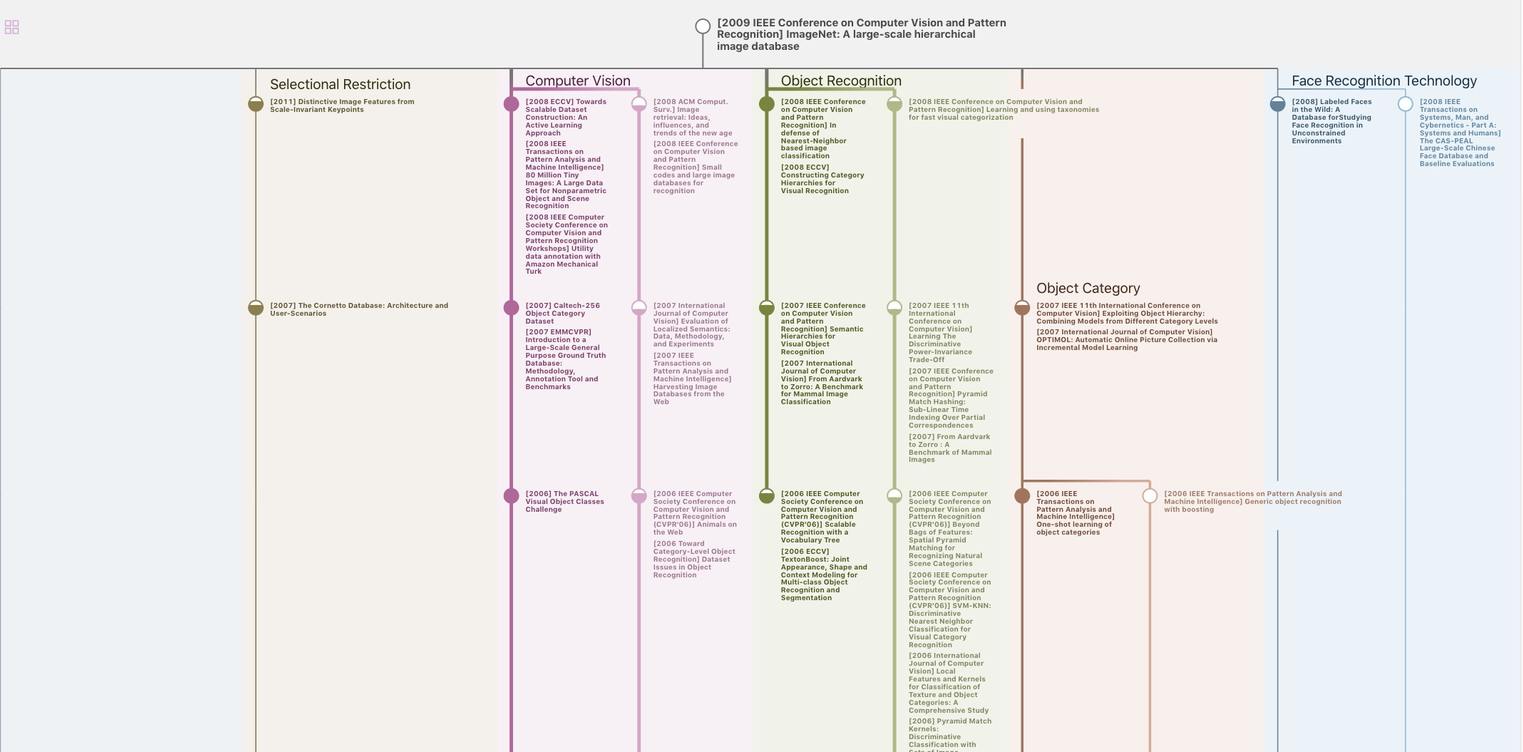
生成溯源树,研究论文发展脉络
Chat Paper
正在生成论文摘要