Joint Graph Regularization in a Homogeneous Subspace for Cross-Media Retrieval.
JOURNAL OF ADVANCED COMPUTATIONAL INTELLIGENCE AND INTELLIGENT INFORMATICS(2019)
摘要
The heterogeneity of multimodal data is the main challenge in cross-media retrieval; many methods have already been developed to address the problem. At present, subspace learning is one of the mainstream approaches for cross-media retrieval; its aim is to learn a latent shared subspace so that similarities within cross-modal data can be measured in this subspace. However, most existing subspace learning algorithms only focus on supervised information, using labeled data for training to obtain one pair of mapping matrices. In this paper, we propose joint graph regularization based on semi-supervised learning cross-media retrieval (JGRHS), which makes full use of labeled and unlabeled data. We jointly considered correlation analysis and semantic information when learning projection matrices to maintain the closeness of pairwise data and semantic consistency; graph regularization is used to make learned transformation consistent with similarity constraints in both modalities. In addition, the retrieval results on three datasets indicate that the proposed method achieves good efficiency in theoretical research and practical applications.
更多查看译文
关键词
cross-media retrieval,subspace learning,semi-supervised,graph Laplacian
AI 理解论文
溯源树
样例
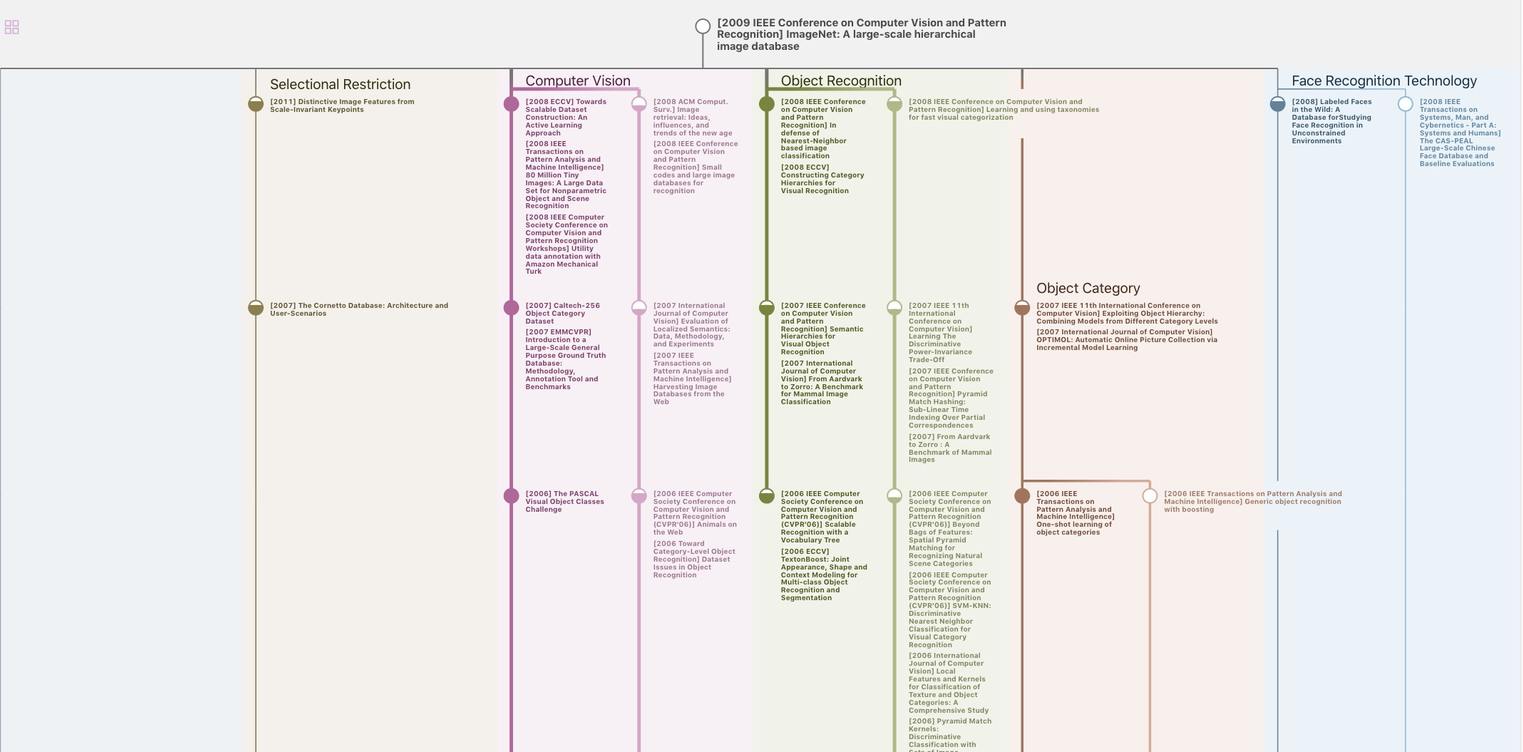
生成溯源树,研究论文发展脉络
Chat Paper
正在生成论文摘要